- 1Department of Pathophysiology, Anhui Medical University, Hefei, China
- 2Department of Biological Sciences, Georgia Institute of Technology, Atlanta, GA, United States
Breast cancer development and progression rely not only on the proliferation of neoplastic cells but also on the significant heterogeneity in the surrounding tumor microenvironment. Its unique microenvironment, including tumor-infiltrating lymphocytes, complex myeloid cells, lipid-associated macrophages, cancer-associated fibroblasts (CAFs), and other molecules that promote the growth and migration of tumor cells, has been shown to play a crucial role in the occurrence, growth, and metastasis of breast cancer. However, a detailed understanding of the complex microenvironment in breast cancer remains largely unknown. The unique pattern of breast cancer microenvironment cells has been poorly studied, and neither has the supportive role of these cells in pathogenesis been assessed. Single-cell multiomics biotechnology, especially single-cell RNA sequencing (scRNA-seq) reveals single-cell expression levels at much higher resolution, finely dissecting the molecular characteristics of tumor microenvironment. Here, we review the recent literature on breast cancer microenvironment, focusing on scRNA-seq studies and analyzing heterogeneity and spatial location of different cells, including T and B cells, macrophages/monocytes, neutrophils, and stromal cells. This review aims to provide a more comprehensive perception of breast cancer microenvironment and annotation for their clinical classification, diagnosis, and treatment. Furthermore, we discuss the impact of novel single-cell omics technologies, such as abundant omics exploration strategies, multiomics conjoint analysis mode, and deep learning network architecture, on the future research of breast cancer immune microenvironment.
Introduction
Breast cancer is the most frequent cancer and the leading cause of cancer-related death in women worldwide. Breast cancer progression is a complex process that coordinates the crosstalk between tumor cells and the components of tumor microenvironment (TME) (1). Breast TME as tumor components can dynamically program tumor growth (1). Tumor microenvironment generally includes immune cells, stromal cells, blood vessels, and extracellular matrix (ECM). Most of the stromal cells and immune cells experience some changes and play roles in both the suppression and progression of tumor (2, 3). In breast cancer, some TME components can modulate immune cells to counteract their intrinsic antitumor activity. For example, neutrophils exhibit tumor cytotoxicity during early disease stages, whereas in high burden tumor, they can be reprogramed to promote disease progression and dissemination (4). Therefore, breast TME is essential for the survival and immunosuppression of tumor cells.
Tumor environment supports tumor cell survival and evolution in the face of various tumor-adverse interventions (5), and destruction of the TME homeostasis can force tumor cell apoptosis and activate the T-cell-mediated cytotoxicity (6–8). TME can also modulate angiogenesis, cytokine secretions, and immune cell recruitment (9, 10).
Traditionally, biological experiments for TME analysis such as immunohistochemistry (IHC), immunofluorescence (IF), the emerging cytometry by time-of-flight (CyTOF) (11), or latest multiplexed ion beam imaging by time-of-flight (MIBI-TOF) (12) could only target certain cell populations preventing a holistic analysis of the highly heterogenous TME. However, the rise of single-cell omics in the past 10 years allowed us to understand the changes in cell populations, biochemical profile, and immune state of the TME during disease progression and partially addressed the shortcomings of purely biological assays.
The emergence of these novel technologies explores a myriad of factors in the TME that were previously unattainable. FACS-based smart-seq2 or nanowell-based platforms explore cell alternative splicing (13, 14), while Sci-RNA-seq can detect rare cell populations such as cancer stem cell, circulating tumor cell, or rare immune cells (15, 16). Single-cell assay for transposase-accessible chromatin sequencing (scATAC-seq) and ChIP sequencing (scChIP-seq) epigenetically explore chromatin accessibility and transcriptional factor regulation (17, 18); whereas, scDNA-seq has become the most widely used assay to evaluate single-cell copy-number aberration (19). Meanwhile, the continuous design of integrated tools for single-cell omics not only detected cell heterogeneity but also extended analysis for transcription-based cell cloning aberration (20), cell traceability (21), cell-to-cell interaction (22, 23), rare cell resolution (24), and disease process simulation (25), giving us a deeper understanding of the intricate tumor malignancy. Therefore, this review summarizes the current state of the art on the analysis of breast cancer tumor microenvironment from single-cell omics (Figure 1). Altogether, we construct a comprehensive view of the TME and track the complex dynamic relationship between immune and stromal cells. Lastly, we discuss the near future research tendencies of single-cell omics and its impact in breast cancer research.
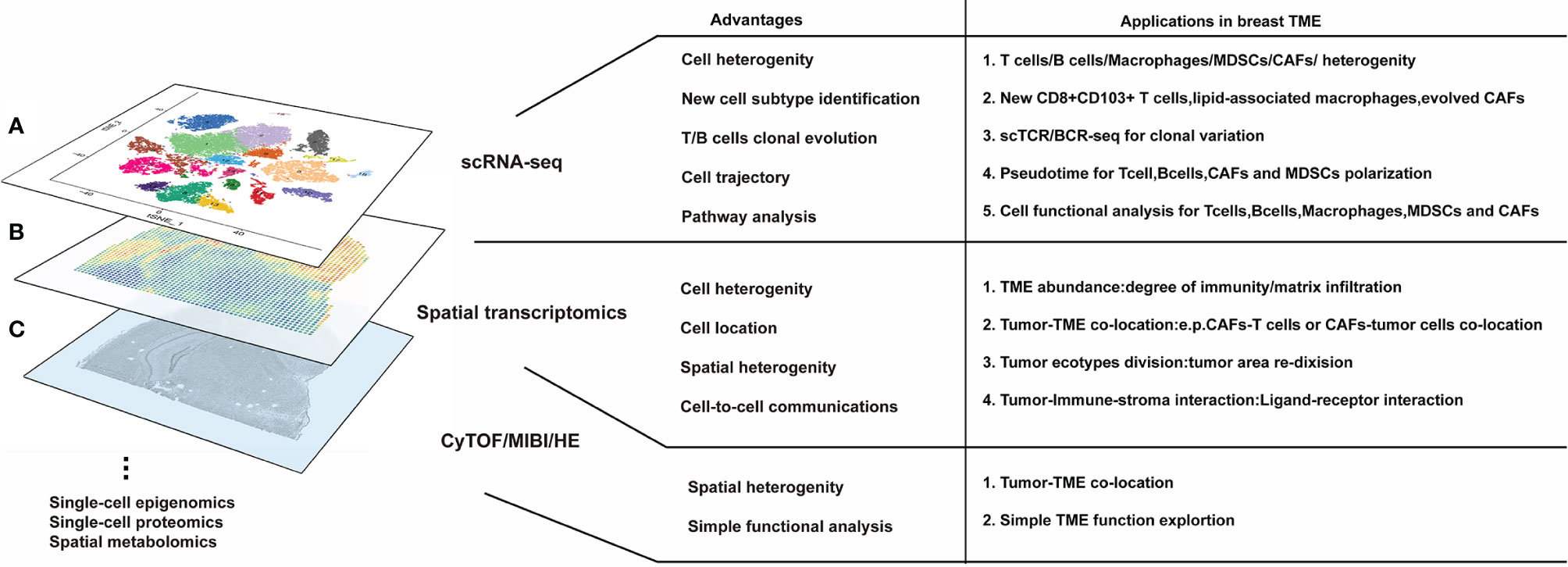
Figure 1 Several high-dimensional approaches for understanding breast tumor microenvironment (TME) composition and interaction. (A) The advantages of single-cell RNA sequencing. scRNA-seq is the most modern and popular technology for breast TME analysis, which is applicable for cell heterogeneity analysis and new cell subtype identification; moreover, scRNA-seq can be derived for T/B cell clonal evolution, cell trajectory, and pathway analysis in breast TME studies. (B) Meanwhile, the advantages of spatial transcriptomics are used to research cell orientation, tumor ecotypes, and cell-to-cell communication in breast TME. (C) The basal HE/CyTOF/MIBI are always used for visualization and assessment of breast TME; however, they have limited information for breast TME analysis. None of these methods can reach the three standards of single-cell level, high-throughput, and in situ reproducibility at the same time, so scRNAseq, spatial transcriptomics, or HE/CyTOF/MIBI are often combined to analyze the tumor microenvironment from multiple dimensions. Furthermore, a variety of omics methods (such as single-cell epigenomics, proteomics, and spatial metabolomics) can still be utilized for breast TME research but are more difficult to implement due to lack of evidence or technical limitations.
The Plasticity of Breast Cancer Microenvironment
Surprisingly, preneoplastic cells do not significantly change the microenvironment for early malignant transformation and there is no difference of the breast microenvironment between preneoplastic and normal breast tissues (26). In fact, malignant cells are responsible for tumor microenvironment reprogramming likely caused by the exponential division of tumor cells and immune cell recruitment. Therefore, the microenvironment is constantly changing with tumor progression, from tiny tumor foci to palpable tumor mass. Early “indolent” tumor areas are dominated by infiltrating T cells and B cells with immune killing properties. However, progressive tumor regions require increased proliferation and an immunosuppressive milieu. Immune-suppressive T cells secrete IL-17 to recruit neutrophils and macrophages (27), which play an important role in higher myeloid cell infiltration and tumor metastasis in the clinical patients.
The Heterogeneity of Lyphocytes in Breast TME
Classical prediction analysis of microarray 50 (PAM50) classifies breast cancer into luminal A, luminal B, HER2+, and basal-like subtypes according to the expression of estrogen receptor (ER), progesterone receptor (PR), and HER2 (28, 29). Regardless, clone aberration generates high heterogeneity even within each breast cancer subtype (30), so do with TME. Elham Azizi et al. used scDrop-seq to analyze CD45+ immune cell in 8 breast cancer patients and found that the immune cell subtypes were highly heterogeneous. T-cell fractions are the most abundant immune cells (21%–96%) in breast TME, followed by myeloid cells. Moreover, T cells in tumor and adjacent lesions had transcriptional similarities, which were significantly different from those in the peripheral circulatory system, indicating reprogramming of T cells by local primary tumors. Functionally, T cells were flexible in oxidative phosphorylation, IFN, TNF-a, TGF-b, IL-6/JAK/STAT, hypoxia, proinflammation, activation, and cytolytic effector pathways in local primary tumors. These reflected various differentiated and activated states in 32 T-cell clusters, but still immunogenic and exerting immune responses. Moreover, combined antigenic TCR stimulation and environmental factors reprogrammed similar biological function to form T-cell niches. For example, similar CD4+ T-cell populations exist in different breast cancer patients, such as homogenous population of Treg immunosuppressive cells prevalently present in all breast cancer subtypes. Interestingly, in addition to naturally Treg cells, traceability analysis found tumors could repolarize CD4+ conventional T cells to immunosuppressive Tregs (31). Whereas CD4+ and CD8+ T cells are widely dysfunctional, some representative CD8+ T-cell subgroups had significant immune suppressive activity (26, 32). These interact with Tregs and PD-L1+ tumor-associated macrophages (TAMs) to establish complex immunosuppressive niches (33). Therefore, the elimination of Tregs and PD-L1+ TAMs in the tumor may recover cytotoxic effect of T cells and enhance the effect of immune checkpoint blockade (ICB). Simultaneously, some T cells expressed less PD-1 but other immune checkpoints such as TIGIT and LAG3 which might prove novel ICB targets (34). Furthermore, a typical CD8+CD103+ tissue-resident memory cell population was also considered to be immunosuppressive. This T-cell subset seems to respond to immune checkpoint blockade (ICB) (35).
Interestingly, when comparing the immune microenvironment in different PAM50 subtypes, ER− patients had the most Tregs, PD-L1+ TAMs, and PD-1 high CTLA-4+ CD38+ exhausted T cells, revealing that breast cancer microenvironment is remodeled by the endocrine system to promote immunosuppressive function. This also explained why ER− patients are more likely to benefit from immunotherapy (33).
The specific role of B cells is still largely unknown in breast cancer and other cancers. It remains uncertain if B cells promote or restrict tumor growth. Hu et al. performed scRNA-seq-based BCR-seq analysis on B cells of breast cancer patients and showed that the B cell increased BCR diversity in the tumor, demonstrating the clone evolution and complex immunogenicity. The B-cell populations in the tumor were classified into 7 groups, including naïve B cells, IGM+CD27+ memory B cells, IGM+CD27− atypical memory B cells, class-switched memory B cells, plasma cells, germinal center B cells, and CD14+ atypical B cells. Among these, memory B cells were the most abundant cell subgroup in the tumor, which differs from the enriched naïve B-cell population in the peripheral blood. Nevertheless, intratumoral B cells mainly showed potential immunogenicity and antigen presentation activity, and no specific cell population seemed to contribute directly to cytotoxic or immunosuppressive function. Hence, the specific function of B cells in the TME remained unclear (36). Recently, B cells were associated to ICB response in renal cell carcinoma (37), melanoma (38), and sarcoma (39). Sc-RNAseq analysis of B-cell function in breast cancer patients proved its respond to immune therapy and produced cascaded antibodies to activate cytotoxic T cells and amplify the ICB effect (40). Noteworthy, B cells in response to chemotherapy retained plasticity, as chemotherapeutic TME signals, such as complement signaling and inflammatory response, generate ICOSL+ B cells. These boost effector T-cell activation or inversely reverse B cells into immunosuppressive CD55+ B cells for chemoresistance (41). Hence, the function of B cells seems multidirectional, and proper induction of memory B-cell activation and antibody secretion is likely necessary for immune activation.
Complex Myeloid Cell Subsets in Breast Cancer
Myeloid cells, including neutrophils, monocytes, and macrophages, propel tumor progression, mainly via immunosuppression and cytokines secretion, but huge heterogeneity exits in myeloid cells (42). Macrophages are the most widespread myeloid cell group in tumor lesions and can polarize towards proinflammatory M1 or immunosuppressive M2 phenotype. M2-type genes, such as CD276, CD163, MS4A6A, and TGFB1, are widely expressed in tumor-associated macrophages and showed the characteristics of cascade M2 differentiation (32, 34, 43).
Recently, depletion of protumor macrophages with CSF1R neutralizing antibodies was shown inefficient to inhibit tumor progression (44). Sc-RNAseq identified gene sets related to M1 and M2 phenotypes, and the conversion of M2 phenotype to M1 immune-activated macrophages was an effective measure to stimulate the immune response (45, 46) and amplify ICB therapy. Moreover, a new type of lipid-associated macrophages has been found in tumors that highly express lipid metabolism genes such as Fabp5 or Apoe but not in the conventional M1/M2 classification. These macrophages also expressed PD-L1 and PD-L2 for immunoregulation (47). Considering the rich lipid sources of breast TME and the fatty acid dependence of tumor cells (48), lipid-associated macrophages may belong to the tissue-resident macrophage population that are reprogrammed by tumor cells and the metabolic drugs might be targeted.
Finally, bone marrow-derived suppressor cells (MDSCs), including polymorphonucler MDSCs (PMN-MDSCs) and monocytic MDSCs (M-MDSCs), were identified in breast tumor patients and gradually infiltrate tumor site with disease progression (27). Similar to T cells, the heterogeneity of neutrophils and monocytes could still be seen in tumors and spleen. Both tissues contained both normal mature myeloid cell populations (Camp/Lcn2/Ltf+ neutrophils) and immunosuppressive bone marrow-derived suppressor cells (CD84/Il1b/Spi1+ PMN-MDSCs). These two cell types coexist and are different from a common myeloid progenitor, proving the ability of tumor cells to modulate myeloid differentiation rather than the repolarizing mature neutrophils into CD84 and ROS high MDSC subgroup, which mainly support the immunosuppressive environment (49). In fact, compared with their role at the tumor site, neutrophils seem to play a more prominent role in tumor dissemination, metastasis, and recurrence (50–54). Circulating tumor cells (CTCs) secret cytokines CSF1, CSF3, TGF-β3, and IL-15 to recruit PMN-MDSCs to physically cluster with CTCs. Subsequently, CTC-bonded PMN-MDSCs secrete inflammatory factors TNF-α, OSM, IL-1β, and IL-6 in CTC-PMN-MDSCs niche to improve the proliferation, stress resistance of tumor cells, and CTC cluster formation (55).
CAFs Functional Subclusters in Breast Cancer
Cancer-associated fibroblasts (CAFs) are a major component of the stroma in tumor. Healthy breast matrix is destroyed with tumor progression as healthy fibroblasts reduce and tumor cells reprogram fibroblasts to CAFs (56). CAFs are scattered inside the tumor tissue rather than in the surrounding area, relying on its strong secretion and tissue adhesion capacity. Traditional CAFs promote tumor progression mainly through stromal remodeling, immunosuppression, and neovascularization (57), but CAFs are a group of heterogeneous cells without a unified cell maker and of uncertain origin. Stromal cells such as MSCs, endothelial cells, and pericytes, are strong candidates for CAF progenitor cells (58).
Single-cell transcriptomic studies of intratumoral heterogeneous CAFs showed two prime functions including nidogen+ perivascular fibroblasts and fibulin+ stromal fibroblasts. Nidogen+ perivascular fibroblasts highly expressed vascular production regulators NOTCH3, EPAS1, COL18A1, and NR2F2 and were enriched for perivascular markers ACTA2, MCAM, CAV1, TAGLN, MYH11, MYLK, and RGS5, suggesting a role in the regulation of neovascularization in tumors under increased oxygen demand settings. Another fibulin+ stroma-related CAFs highly expressed matrix-related DCN, LUM, VCAN, LOX, secret collagens, and chemokines CXCL12 and CXCL14. These seemed to act as tumor functional fibroblasts that participate in tumor stromal formation and immune response. Interestingly, stroma-related CAFs gradually shrunk with tumor progression, which may be due to tumor space occupation, low tumor adhesion, and metastasis requirements (59, 60).
Alternatively, CAFs were divided into three functional subgroups: (1) α-SMA+ myofibroblasts (myCAFs) which maintain tumor structural stability and ECM remodeling, (2) inflammatory fibroblasts (iCAFs) which regulate immune response, and (3) extracellular matrix fibroblasts (ECM-CAF) which remodel the extracellular matrix of tumors. These functional cells can directly or indirectly interact with tumor cells, myeloid cells, and T cells to promote immunosuppressive milieu (61–63). Spatially, dispersed iCAFs colocalized with all lymphocyte cells, responding to chemokines (CXCL12/CXCL14-CXCR4 and CXCL10-CXCR3), complement, transforming growth factor-β (TGFB1/TGFB3-TGFBR2), and lymphocyte inhibitory/activation molecules (LTB-LTBR, TNFSF14-LTBR and LTB-CD40, VTCN1/B7H4-BTLA). In contrast, marginal myCAFs only interact directly with CD8+ T cells for tumor invasion and matrix remodeling (47). Interestingly, in the high-grade pregnancy-associated breast cancer, the tumor reprograms these fibroblasts into a more function-evolved phenotype. Those fibroblasts commonly express higher COL1A1, CXCL12, TGFB1, and MMP3 and have a unique fatty acid metabolism, peroxisome, and inflammatory profile (62). Therefore, the degree of malignancy of breast tumor may affect the function of CAFs.
Breast Cancer Immunotherapy Response Microenvironment
ScRNA-seq-based supervision of the immune response following ICB is necessary to fully understand mechanism of action and expose emerging resistance pathways (64). Breast cancer patients benefiting from ICB therapy already exhibit expanded PD-1+ T cells before ICB therapy compared with nonresponders. Matured CD4+ Th1 and Tfh cells and exhausted CD8+ effector T cells greatly expand after anti-PD-1 therapy, with higher proliferation, immune checkpoint protein (LAG3, HAVCR2, PDCD1), effector (IFNG, NKG7), and cytotoxicity (GMZB, PRF1), higher TCR richness, and lower TCR clonality. These responsive T cells positively correlate with PD-L1+PD-L2+ DCs, PDL1+ CCR2+, or MMP9+ macrophages or MHC I/II+ cancer cells, mainly through costimulatory CD28-CD80, ICOS-ICOSLG, coinhibitory PDCD1-CD274/PDCD1LG2, HAVCR2-LGALS9, and CLTA4-CD80/CD86. In contrast, TCF7+ Sell+ naïve T cells and CX3CR1+ or C3+ macrophages are inversely correlated with T-cell expansion. More importantly, a specific gene set related to T-cell expansion has been identified for the prediction of ICB response (65). Similar to the functional B cells in chemotherapy (41), the abundant B-cell population in breast tumors are activated by antigen presentation after ICB therapy to promote T follicular helper cell expansion and cytotoxic CD8+ T cells for immunotherapy sensitization (40), which recalls breast TME into “immune hot” microenvironment.
Spatial Distribution of Breast TME
Besides the heterogeneity and TCR/BCR clonal evolution of stromal and immune cells, tumor histological regions are demarcated by TME to form heterogeneous tumor organization (30). However, single-cell dissociation destroys the intact tissue structure in scRNA-seq (33) and cell location and orientation information were lost during tissue dissociation. Therefore, tremendous effort has been put into the development of a variety of a three-dimensional (3D) near-realistic cell environment. Spatial transcriptome analysis and single-cell-level depth of tumor pathology (66) was developed to evaluate cell types and their locations. In 2018, Keren et al. first used multiplexed ion beam imaging by time-of-flight (MIBI-TOF) to simultaneously analyze 36 proteins at single-cell resolution and draw a rough single-cell map of breast cancer immune distribution. This study highlighted that function-similar immunoregulator cells cluster together for stronger effects. For example, KI67+ proliferating cells or IDO+ immunoregulatory cells formed proliferated or immunoregulatory units within the tumor. Moreover, tumors were divided into three immune categories: (1) “cold tumors” with less immune cell infiltration, (2) “compartmentalized tumors” where immune cells were distributed around tumor cells in an organized regional orientation, and CD4+ T cells were mainly near PD-1+ cells in the tumor-immune border, and (3) “mixed tumors” with tumor cells and immune cells mixed together without borders, where CD8+ T cells were dominant and had relative stronger immunosuppression with worse prognosis (12).
Based on stromal distribution, topological network of high-dimensional single-cell mass cytometry images, tumors were compartmentalized in: (1) low stromal environment (including immune cell infiltration and non-TME cell infiltration), (2) highly vascularized regions, (3) vimentin high fibroblasts regions, (4) fibronectin high fibroblasts regions, and (5) multicellular dispersive types, where fibroblasts are distributed around tumor cells and blood vessels which are consistent with previous observation (59). Meanwhile Ki67+ tumor cells and T-cell infiltration were increased around the blood vessels.
The traditional PAM50 is too generic to reveal the tumor composition within individual patients. Based on tumor cell metacluster compositions and environmental interaction, tumor lesions can be divided into 18 single-cell pathology subgroups (SPCs), and then matched with stromal models to establish 11 stromal environment subgroups. Interestingly, these immunological patterns and pathological subtypes were correlated with overall survival. For example, hypoxic SPC17 TNBC often exhibited large, stroma-deficient tumor regions, whereas SPC 13-16 TNBC showed T-cell-enriched or macrophage-enriched regions, and HR+ tumor was immune cold, accompanied by a range of fibroblast-enriched stromal environment (67). For now, the basic elementary interaction between tumor cells and TME have been explored, but more complex interactions with specific tumor regions and cell subtypes still need further elucidation.
High-resolution spatial transcriptomics can distinguish hundreds of different spots in the tissue (68); each containing tens of cells which expression profile can be deconvoluted with RNA sequencing. Coupled with the resolution of fluorescence in situ hybridization (FISH) and in situ sequencing (ISS), spatial transcriptome analysis of intact tissue sections can expose spatial nanoscale-resolution imaging (68, 69). When combined with sc-RNAseq and spatial transcriptome positioning on breast cancer patients, this technique confirmed the aforementioned cell heterogeneity and explored the interaction within specific functional TME cells (Figure 2). Primary tumors were first divided into 9 different gene regulatory pattern regions based on tumor biology and TME components (tumor ecotypes). Some ecotypes enriched basal subtype classification, cycling and luminal progenitor cells, and few immune cell infiltrations, which corresponded to very poor 5-year survival. Another highly immune cell-infiltrating ecotype is represented by a higher response to ICB therapy (47).
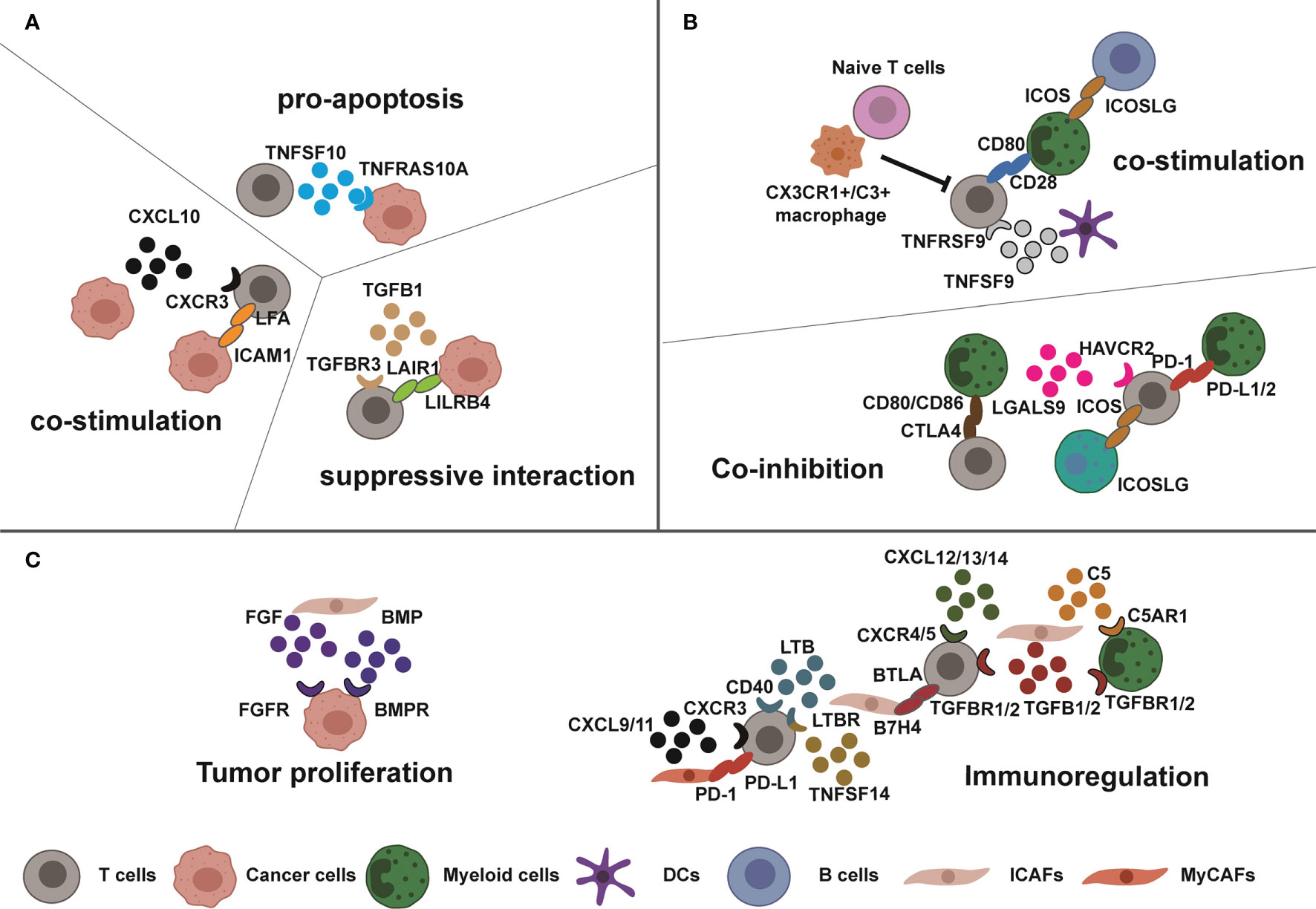
Figure 2 Complex interactions in breast tumor microenvironment. Tumor cells, immune cells, and CAFs exhibit high interactions which dynamically change cellular functions. (A, B) Sometimes, the functions of these interactions are opposite such as the costimulatory or suppressive interaction between T cells and tumor cells or myeloid cells due to specific tumor homeostasis. (C) Meanwhile, the CAFs interact with T cells, cancer cells, and myeloid cells for tumor progression and immunosuppression through cytokines and immunomodulatory proteins.
Recently, deep learning convolutional algorithms were established to directly learn single-cell spatial transcriptomes and matched them to any HE-stained tissue section. These can be used for tumor and TME cell model diagnosis prediction (70). Hopefully, after accumulating enough spatial transcriptome patterns, clinical pathological deep learning algorithm-based HE images can directly recognize accurate tumor subtypes and make more precise therapeutic interventions.
Outlook
The current single-cell omics results have drawn a relatively detailed map of the breast TME, distinguished stromal cells and immune cells into functional populations (Table 1), and greatly enriched the TME components for clinical diagnosis and targeted therapy intervention. Unfortunately, many more in-depth technical methods and joint application methods have not yet fully matured, since single-cell omics only just emerged. The microenvironment related to the occurrence and development of breast cancer has not been fully researched.
1. Some cell populations in tumors such as NK cells, neutrophils, and DCs have not been fully understood,
2. The microenvironment composition is still unclear in the tumor initiation, dissemination, and metastasis. Macrophages are necessary for early breast tumorigenesis (71), but single-cell transcriptome can hardly identify the microenvironment composition of early tumorigenesis due to the difficulty to capture small lesions and cell number limitations.
3. The current microenvironment recognition method is mainly established by single-cell transcriptome and antibody-dependent multidimensional imaging. However, immune cells are functionally regulated by numerous transcription factors (21), hence, other single-cell strategies such as scATAC-seq, scChIP-seq, and the emerging CRISPR screening (72, 73) will be useful for breast TME analysis.
4. TME analysis needs to pay more attention to the combination of cell function and spatial distribution in the future. For example, metabolic deprivation of polytrophic tumor cells transforms the shared TME metabolism dependence (74, 75); whereas, various TME cells have different substrate dependencies for its activation or immunosuppression, such as the correlation between TCA cycle and phenotypic states of T cells (32), high lipid metabolism in lipid-related macrophages (47), and high fatty acid metabolism in function-evolved CAFs (62). However, there is still no systematic research on breast TME immunometabolism. Indeed, combining scRNA-seq and spatial transcriptomics enables detailed analysis of cellular metabolic pathways as well as regional metabolic pathway enrichment. Moreover, the combination of spatial transcriptome and spatial metabolome (76, 77) will clarify the metabolic status in indicated tumor lesions. Using spatial metabolome to analyze TME metabolic pathways, combined with spatial transcriptome diagnosis of TME cell subtypes, can accurately detect the metabolite preference of TME cells. Further research is needed to explore these methods in detail.
5. Similarly, single-cell proteomics has not yet been completed (78). Current scLC-MS-based proteomics technology SCoPE-MS and NanoPOTs sample preparation technology can only quantify ~1,000 proteins per cell across thousands of individual cells (79–81), not to say the detailed biological analysis, carrier proteome, and data standardization (82–84).
6. Deep learning-based neural networks may identify novel breast cancer subtypes, applying more cost-effective and rapid single-cell analysis to clinical tumor patients. This will strengthen pathological diagnosis and therapeutic intervention optimization.
In summary, the evolution of novel single-cell omics technology, including abundant omics exploration strategies, multiomics conjoint analysis mode, and deep learning network architecture is still developing and has the potential to revolutionize our understanding of the TME, its changes with disease progression, and its response to therapy.
Here, we focused on the applications and advances of single-cell omics to unveil the heterogeneity, pathogenesis, and treatment of breast cancer, describing the complex model of breast cancer microenvironment composition in detail. Despite the massive heterogeneity between breast cancer patients and tumors, the components of the immune microenvironment seem to reflect the patient’s survival and response to immunotherapy, highlighting the importance of fully understanding TME changes and progression.
Author Contributions
HZ and ZT conceived and conducted the project. HZ and SW supervised the project. ZT and HZ wrote the paper. ZT, CK, FY, MW, and MS performed the review organization and analysis. MW contributed to manuscript editing. All authors listed have made a substantial, direct, and intellectual contribution to the work and approved it for publication.
Funding
This work was supported by the National Natural Science Foundation of China (reference numbers 81670097, 81870085, and 81273004), Grants for Scientific Research Enhancement of Anhui Medical University (2019xkjT004 and XJ2020019), and Grants for Collaborative Innovation Project of Colleges and Universities in Anhui Province (GXXT-2021-063).
Conflict of Interest
The authors declare that the research was conducted in the absence of any commercial or financial relationships that could be construed as a potential conflict of interest.
Publisher’s Note
All claims expressed in this article are solely those of the authors and do not necessarily represent those of their affiliated organizations, or those of the publisher, the editors and the reviewers. Any product that may be evaluated in this article, or claim that may be made by its manufacturer, is not guaranteed or endorsed by the publisher.
Acknowledgments
We thank everyone in the Pathophysiology Department of Anhui Medical University for all their constructive comments and advice.
References
1. Binnewies M, Roberts EW, Kersten K, Chan V, Fearon DF, Merad M, et al. Understanding the Tumor Immune Microenvironment (TIME) for Effective Therapy. Nat Med (2018) 24(5):541–50. doi: 10.1038/s41591-018-0014-x
2. Turley SJ, Cremasco V, Astarita JL. Immunological Hallmarks of Stromal Cells in the Tumour Microenvironment. Nat Rev Immunol (2015) 15(11):669–82. doi: 10.1038/nri3902
3. Guo S, Deng CX. Effect of Stromal Cells in Tumor Microenvironment on Metastasis Initiation. Int J Biol Sci (2018) 14(14):2083–93. doi: 10.7150/ijbs.25720
4. Wu M, Ma M, Tan Z, Zheng H, Liu X. Neutrophil: A New Player in Metastatic Cancers. Front Immunol (2020) 11:565165. doi: 10.3389/fimmu.2020.565165
5. Plaks V, Kong N, Werb Z. The Cancer Stem Cell Niche: How Essential is the Niche in Regulating Stemness of Tumor Cells? Cell Stem Cell (2015) 16(3):225–38. doi: 10.1016/j.stem.2015.02.015
6. Lim B, Woodward WA, Wang X, Reuben JM, Ueno NT. Inflammatory Breast Cancer Biology: The Tumour Microenvironment is Key. Nat Rev Cancer (2018) 18(8):485–99. doi: 10.1038/s41568-018-0010-y
7. Jia H, Truica CI, Wang B, Wang Y, Ren X, Harvey HA, et al. Immunotherapy for Triple-Negative Breast Cancer: Existing Challenges and Exciting Prospects. Drug Resist Update (2017) 32:1–15. doi: 10.1016/j.drup.2017.07.002
8. Keenan TE, Tolaney SM. Role of Immunotherapy in Triple-Negative Breast Cancer. J Natl Compr Canc Netw (2020) 18(4):479–89. doi: 10.6004/jnccn.2020.7554
9. Hinshaw DC, Shevde LA. The Tumor Microenvironment Innately Modulates Cancer Progression. Cancer Res (2019) 79(18):4557–66. doi: 10.1158/0008-5472.CAN-18-3962
10. Vitale I, Shema E, Loi S, Galluzzi L. Intratumoral Heterogeneity in Cancer Progression and Response to Immunotherapy. Nat Med (2021) 27(2):212–24. doi: 10.1038/s41591-021-01233-9
11. Xu Y, Su GH, Ma D, Xiao Y, Shao ZM, Jiang YZ. Technological Advances in Cancer Immunity: From Immunogenomics to Single-Cell Analysis and Artificial Intelligence. Signal Transduct Target Ther (2021) 6(1):312. doi: 10.1038/s41392-021-00729-7
12. Keren L, Bosse M, Marquez D, Angoshtari R, Jain S, Varma S, et al. A Structured Tumor-Immune Microenvironment in Triple Negative Breast Cancer Revealed by Multiplexed Ion Beam Imaging. Cell (2018) 174(6):1373–1387.e19. doi: 10.1016/j.cell.2018.08.039
13. Ramskold D, Luo S, Wang YC, Li R, Deng Q, Faridani OR, et al. Full-Length mRNA-Seq From Single-Cell Levels of RNA and Individual Circulating Tumor Cells. Nat Biotechnol (2012) 30(8):777–82. doi: 10.1038/nbt.2282
14. Goldstein LD, Chen YJ, Dunne J, Mir A, Hubschle H, Guillory J, et al. Massively Parallel Nanowell-Based Single-Cell Gene Expression Profiling. BMC Genomics (2017) 18(1):519. doi: 10.1186/s12864-017-3893-1
15. Cao J, Spielmann M, Qiu X, Huang X, Ibrahim DM, Hill AJ, et al. The Single-Cell Transcriptional Landscape of Mammalian Organogenesis. Nature (2019) 566(7745):496–502. doi: 10.1038/s41586-019-0969-x
16. Cao J, Packer JS, Ramani V, Cusanovich DA, Huynh C, Daza R, et al. Comprehensive Single-Cell Transcriptional Profiling of a Multicellular Organism. Science (2017) 357(6352):661–7. doi: 10.1126/science.aam8940
17. Lareau CA, Duarte FM, Chew JG, Kartha VK, Burkett ZD, Kohlway AS, et al. Droplet-Based Combinatorial Indexing for Massive-Scale Single-Cell Chromatin Accessibility. Nat Biotechnol (2019) 37(8):916–24. doi: 10.1038/s41587-019-0147-6
18. Grosselin K, Durand A, Marsolier J, Poitou A, Marangoni E, Nemati F, et al. High-Throughput Single-Cell ChIP-Seq Identifies Heterogeneity of Chromatin States in Breast Cancer. Nat Genet (2019) 51(6):1060–6. doi: 10.1038/s41588-019-0424-9
19. Yin Y, Jiang Y, Lam KG, Berletch JB, Disteche CM, Noble WS, et al. High-Throughput Single-Cell Sequencing With Linear Amplification. Mol Cell (2019) 76(4):676–90.e10. doi: 10.1016/j.molcel.2019.08.002
20. Gao R, Bai S, Henderson YC, Lin Y, Schalck A, Yan Y, et al. Delineating Copy Number and Clonal Substructure in Human Tumors From Single-Cell Transcriptomes. Nat Biotechnol (2021) 39(5):599–608. doi: 10.1038/s41587-020-00795-2
21. Buenrostro JD, Corces MR, Lareau CA, Wu B, Schep AN, Aryee MJ, et al. Integrated Single-Cell Analysis Maps the Continuous Regulatory Landscape of Human Hematopoietic Differentiation. Cell (2018) 173(6):1535–48.e16. doi: 10.1016/j.cell.2018.03.074
22. Kumar MP, Du J, Lagoudas G, Jiao Y, Sawyer A, Drummond DC, et al. Analysis of Single-Cell RNA-Seq Identifies Cell-Cell Communication Associated With Tumor Characteristics. Cell Rep (2018) 25(6):1458–68.e4. doi: 10.1016/j.celrep.2018.10.047
23. Giladi A, Cohen M, Medaglia C, Baran Y, Li B, Zada M, et al. Dissecting Cellular Crosstalk by Sequencing Physically Interacting Cells. Nat Biotechnol (2020) 38(5):629–37. doi: 10.1038/s41587-020-0442-2
24. Ebinger S, Ozdemir EZ, Ziegenhain C, Tiedt S, Castro Alves C, Grunert M, et al. Characterization of Rare, Dormant, and Therapy-Resistant Cells in Acute Lymphoblastic Leukemia. Cancer Cell (2016) 30(6):849–62. doi: 10.1016/j.ccell.2016.11.002
25. Van Egeren D, Escabi J, Nguyen M, Liu S, Reilly CR, Patel S, et al. Reconstructing the Lineage Histories and Differentiation Trajectories of Individual Cancer Cells in Myeloproliferative Neoplasms. Cell Stem Cell (2021) 28(3):514–23.e9. doi: 10.1016/j.stem.2021.02.001
26. Pal B, Chen Y, Vaillant F, Capaldo BD, Joyce R, Song X, et al. A Single-Cell RNA Expression Atlas of Normal, Preneoplastic and Tumorigenic States in the Human Breast. EMBO J (2021) 40(11):e107333. doi: 10.15252/embj.2020107333
27. Sinha VC, Rinkenbaugh AL, Xu M, Zhou X, Zhang X, Jeter-Jones S, et al. Single-Cell Evaluation Reveals Shifts in the Tumor-Immune Niches That Shape and Maintain Aggressive Lesions in the Breast. Nat Commun (2021) 12(1):5024. doi: 10.1038/s41467-021-25240-z
28. Parker JS, Mullins M, Cheang MC, Leung S, Voduc D, Vickery T, et al. Supervised Risk Predictor of Breast Cancer Based on Intrinsic Subtypes. J Clin Oncol (2009) 27(8):1160–7. doi: 10.1200/JCO.2008.18.1370
29. Harbeck N, Gnant M. Breast Cancer. Lancet (2017) 389(10074):1134–50. doi: 10.1016/S0140-6736(16)31891-8
30. Casasent AK, Schalck A, Gao R, Sei E, Long A, Pangburn W, et al. Multiclonal Invasion in Breast Tumors Identified by Topographic Single Cell Sequencing. Cell (2018) 172(1-2):205–17.e12. doi: 10.1016/j.cell.2017.12.007
31. Xydia M, Rahbari R, Ruggiero E, Macaulay I, Tarabichi M, Lohmayer R, et al. Common Clonal Origin of Conventional T Cells and Induced Regulatory T Cells in Breast Cancer Patients. Nat Commun (2021) 12(1):1119. doi: 10.1038/s41467-021-21297-y
32. Azizi E, Carr AJ, Plitas G, Cornish AE, Konopacki C, Prabhakaran S, et al. Single-Cell Map of Diverse Immune Phenotypes in the Breast Tumor Microenvironment. Cell (2018) 174(5):1293–308.e36. doi: 10.1016/j.cell.2018.05.060
33. Wagner J, Rapsomaniki MA, Chevrier S, Anzeneder T, Langwieder C, Dykgers A, et al. A Single-Cell Atlas of the Tumor and Immune Ecosystem of Human Breast Cancer. Cell (2019) 177(5):1330–45.e18. doi: 10.1016/j.cell.2019.03.005
34. Chung W, Eum HH, Lee HO, Lee KM, Lee HB, Kim KT, et al. Single-Cell RNA-Seq Enables Comprehensive Tumour and Immune Cell Profiling in Primary Breast Cancer. Nat Commun (2017) 8:15081. doi: 10.1038/ncomms15081
35. Savas P, Virassamy B, Ye C, Salim A, Mintoff CP, Caramia F, et al. Single-Cell Profiling of Breast Cancer T Cells Reveals a Tissue-Resident Memory Subset Associated With Improved Prognosis. Nat Med (2018) 24(7):986–93. doi: 10.1038/s41591-018-0078-7
36. Hu Q, Hong Y, Qi P, Lu G, Mai X, Xu S, et al. Atlas of Breast Cancer Infiltrated B-Lymphocytes Revealed by Paired Single-Cell RNA-Sequencing and Antigen Receptor Profiling. Nat Commun (2021) 12(1):2186. doi: 10.1038/s41467-021-22300-2
37. Helmink BA, Reddy SM, Gao J, Zhang S, Basar R, Thakur R, et al. B Cells and Tertiary Lymphoid Structures Promote Immunotherapy Response. Nature (2020) 577(7791):549–55. doi: 10.1038/s41586-019-1922-8
38. Cabrita R, Lauss M, Sanna A, Donia M, Skaarup Larsen M, Mitra S, et al. Tertiary Lymphoid Structures Improve Immunotherapy and Survival in Melanoma. Nature (2020) 577(7791):561–5. doi: 10.1038/s41586-019-1914-8
39. Petitprez F, de Reynies A, Keung EZ, Chen TW, Sun CM, Calderaro J, et al. B Cells are Associated With Survival and Immunotherapy Response in Sarcoma. Nature (2020) 577(7791):556–60. doi: 10.1038/s41586-019-1906-8
40. Hollern DP, Xu N, Thennavan A, Glodowski C, Garcia-Recio S, Mott KR, et al. B Cells and T Follicular Helper Cells Mediate Response to Checkpoint Inhibitors in High Mutation Burden Mouse Models of Breast Cancer. Cell (2019) 179(5):1191–206.e21. doi: 10.1016/j.cell.2019.10.028
41. Lu Y, Zhao Q, Liao JY, Song E, Xia Q, Pan J, et al. Complement Signals Determine Opposite Effects of B Cells in Chemotherapy-Induced Immunity. Cell (2020) 180(6):1081–97.e24. doi: 10.1016/j.cell.2020.02.015
42. Zilionis R, Engblom C, Pfirschke C, Savova V, Zemmour D, Saatcioglu HD, et al. Single-Cell Transcriptomics of Human and Mouse Lung Cancers Reveals Conserved Myeloid Populations Across Individuals and Species. Immunity (2019) 50(5):1317–34.e10. doi: 10.1016/j.immuni.2019.03.009
43. Bao X, Shi R, Zhao T, Wang Y, Anastasov N, Rosemann M, et al. Integrated Analysis of Single-Cell RNA-Seq and Bulk RNA-Seq Unravels Tumour Heterogeneity Plus M2-Like Tumour-Associated Macrophage Infiltration and Aggressiveness in TNBC. Cancer Immunol Immunother (2021) 70(1):189–202. doi: 10.1007/s00262-020-02669-7
44. Kumar V, Donthireddy L, Marvel D, Condamine T, Wang F, Lavilla-Alonso S, et al. Cancer-Associated Fibroblasts Neutralize the Anti-Tumor Effect of CSF1 Receptor Blockade by Inducing PMN-MDSC Infiltration of Tumors. Cancer Cell (2017) 32(5):654–68.e5. doi: 10.1016/j.ccell.2017.10.005
45. Molgora M, Esaulova E, Vermi W, Hou J, Chen Y, Luo J, et al. TREM2 Modulation Remodels the Tumor Myeloid Landscape Enhancing Anti-PD-1 Immunotherapy. Cell (2020) 182(4):886–900.e17. doi: 10.1016/j.cell.2020.07.013
46. Sun L, Kees T, Almeida AS, Liu B, He XY, Ng D, et al. Activating a Collaborative Innate-Adaptive Immune Response to Control Metastasis. Cancer Cell (2021) 39(10):1361–74.e9. doi: 10.1016/j.ccell.2021.08.005
47. Wu SZ, Al-Eryani G, Roden DL, Junankar S, Harvey K, Andersson A, et al. A Single-Cell and Spatially Resolved Atlas of Human Breast Cancers. Nat Genet (2021) 53(9):1334–47. doi: 10.1038/s41588-021-00911-1
48. Wang T, Fahrmann JF, Lee H, Li YJ, Tripathi SC, Yue C, et al. JAK/STAT3-Regulated Fatty Acid Beta-Oxidation Is Critical for Breast Cancer Stem Cell Self-Renewal and Chemoresistance. Cell Metab (2018) 27(1):136–50.e5. doi: 10.1016/j.cmet.2017.11.001
49. Alshetaiwi H, Pervolarakis N, McIntyre LL, Ma D, Nguyen Q, Rath JA, et al. Defining the Emergence of Myeloid-Derived Suppressor Cells in Breast Cancer Using Single-Cell Transcriptomics. Sci Immunol (2020) 5(44):eaay6017. doi: 10.1126/sciimmunol.aay6017
50. Yang L, Liu Q, Zhang X, Liu X, Zhou B, Chen J, et al. DNA of Neutrophil Extracellular Traps Promotes Cancer Metastasis via CCDC25. Nature (2020) 583(7814):133–8. doi: 10.1038/s41586-020-2394-6
51. Albrengues J, Shields MA, Ng D, Park CG, Ambrico A, Poindexter ME, et al. Neutrophil Extracellular Traps Produced During Inflammation Awaken Dormant Cancer Cells in Mice. Science (2018) 361(6409):eaao4227. doi: 10.1126/science.aao4227
52. Chen MB, Hajal C, Benjamin DC, Yu C, Azizgolshani H, Hynes RO, et al. Inflamed Neutrophils Sequestered at Entrapped Tumor Cells via Chemotactic Confinement Promote Tumor Cell Extravasation. Proc Natl Acad Sci USA (2018) 115(27):7022–7. doi: 10.1073/pnas.1715932115
53. Sprouse ML, Welte T, Boral D, Liu HN, Yin W, Vishnoi M, et al. PMN-MDSCs Enhance CTC Metastatic Properties Through Reciprocal Interactions via ROS/Notch/Nodal Signaling. Int J Mol Sci (2019) 20(8):1916. doi: 10.3390/ijms20081916
54. Xiao Y, Cong M, Li J, He D, Wu Q, Tian P, et al. Cathepsin C Promotes Breast Cancer Lung Metastasis by Modulating Neutrophil Infiltration and Neutrophil Extracellular Trap Formation. Cancer Cell (2021) 39(3):423–37.e7. doi: 10.1016/j.ccell.2020.12.012
55. Szczerba BM, Castro-Giner F, Vetter M, Krol I, Gkountela S, Landin J, et al. Neutrophils Escort Circulating Tumour Cells to Enable Cell Cycle Progression. Nature (2019) 566(7745):553–7. doi: 10.1038/s41586-019-0915-y
56. Mao X, Xu J, Wang W, Liang C, Hua J, Liu J, et al. Crosstalk Between Cancer-Associated Fibroblasts and Immune Cells in the Tumor Microenvironment: New Findings and Future Perspectives. Mol Cancer (2021) 20(1):131. doi: 10.1186/s12943-021-01428-1
57. Mhaidly R, Mechta-Grigoriou F. Fibroblast Heterogeneity in Tumor Micro-Environment: Role in Immunosuppression and New Therapies. Semin Immunol (2020) 48:101417. doi: 10.1016/j.smim.2020.101417
58. Biffi G, Tuveson DA. Diversity and Biology of Cancer-Associated Fibroblasts. Physiol Rev (2021) 101(1):147–76. doi: 10.1152/physrev.00048.2019
59. Bartoschek M, Oskolkov N, Bocci M, Lovrot J, Larsson C, Sommarin M, et al. Spatially and Functionally Distinct Subclasses of Breast Cancer-Associated Fibroblasts Revealed by Single Cell RNA Sequencing. Nat Commun (2018) 9(1):5150. doi: 10.1038/s41467-018-07582-3
60. Wu SZ, Roden DL, Wang C, Holliday H, Harvey K, Cazet AS, et al. Stromal Cell Diversity Associated With Immune Evasion in Human Triple-Negative Breast Cancer. EMBO J (2020) 39(19):e104063. doi: 10.15252/embj.2019104063
61. Sebastian A, Hum NR, Martin KA, Gilmore SF, Peran I, Byers SW, et al. Single-Cell Transcriptomic Analysis of Tumor-Derived Fibroblasts and Normal Tissue-Resident Fibroblasts Reveals Fibroblast Heterogeneity in Breast Cancer. Cancers (Basel) (2020) 12(5):1307. doi: 10.3390/cancers12051307
62. Valdes-Mora F, Salomon R, Gloss BS, Law AMK, Venhuizen J, Castillo L, et al. Single-Cell Transcriptomics Reveals Involution Mimicry During the Specification of the Basal Breast Cancer Subtype. Cell Rep (2021) 35(2):108945. doi: 10.1016/j.celrep.2021.108945
63. Kieffer Y, Hocine HR, Gentric G, Pelon F, Bernard C, Bourachot B, et al. Single-Cell Analysis Reveals Fibroblast Clusters Linked to Immunotherapy Resistance in Cancer. Cancer Discov (2020) 10(9):1330–51. doi: 10.1158/2159-8290.CD-19-1384
64. Jerby-Arnon L, Shah P, Cuoco MS, Rodman C, Su MJ, Melms JC, et al. A Cancer Cell Program Promotes T Cell Exclusion and Resistance to Checkpoint Blockade. Cell (2018) 175(4):984–97.e24. doi: 10.1016/j.cell.2018.09.006
65. Bassez A, Vos H, Van Dyck L, Floris G, Arijs I, Desmedt C, et al. A Single-Cell Map of Intratumoral Changes During Anti-PD1 Treatment of Patients With Breast Cancer. Nat Med (2021) 27(5):820–32. doi: 10.1038/s41591-021-01323-8
66. Noorbakhsh J, Farahmand S, Foroughi Pour A, Namburi S, Caruana D, Rimm D, et al. Deep Learning-Based Cross-Classifications Reveal Conserved Spatial Behaviors Within Tumor Histological Images. Nat Commun (2020) 11(1):6367. doi: 10.1038/s41467-020-20030-5
67. Jackson HW, Fischer JR, Zanotelli VRT, Ali HR, Mechera R, Soysal SD, et al. The Single-Cell Pathology Landscape of Breast Cancer. Nature (2020) 578(7796):615–20. doi: 10.1038/s41586-019-1876-x
68. Stahl PL, Salmen F, Vickovic S, Lundmark A, Navarro JF, Magnusson J, et al. Visualization and Analysis of Gene Expression in Tissue Sections by Spatial Transcriptomics. Science (2016) 353(6294):78–82. doi: 10.1126/science.aaf2403
69. Alon S, Goodwin DR, Sinha A, Wassie AT, Chen F, Daugharthy ER, et al. Expansion Sequencing: Spatially Precise In Situ Transcriptomics in Intact Biological Systems. Science (2021) 371(6528):eaax2656. doi: 10.1101/2020.05.13.094268
70. He B, Bergenstrahle L, Stenbeck L, Abid A, Andersson A, Borg A, et al. Integrating Spatial Gene Expression and Breast Tumour Morphology via Deep Learning. Nat BioMed Eng (2020) 4(8):827–34. doi: 10.1038/s41551-020-0578-x
71. Linde N, Casanova-Acebes M, Sosa MS, Mortha A, Rahman A, Farias E, et al. Macrophages Orchestrate Breast Cancer Early Dissemination and Metastasis. Nat Commun (2018) 9(1):21. doi: 10.1038/s41467-017-02481-5
72. Wang X, Tokheim C, Gu SS, Wang B, Tang Q, Li Y, et al. In Vivo CRISPR Screens Identify the E3 Ligase Cop1 as a Modulator of Macrophage Infiltration and Cancer Immunotherapy Target. Cell (2021) 184(21):5357–74.e22. doi: 10.1158/1535-7163.TARG-21-P108
73. Lawson KA, Sousa CM, Zhang X, Kim E, Akthar R, Caumanns JJ, et al. Functional Genomic Landscape of Cancer-Intrinsic Evasion of Killing by T Cells. Nature (2020) 586(7827):120–6. doi: 10.1038/s41586-020-2746-2
74. Reinfeld BI, Madden MZ, Wolf MM, Chytil A, Bader JE, Patterson AR, et al. Cell-Programmed Nutrient Partitioning in the Tumour Microenvironment. Nature (2021) 593(7858):282–8. doi: 10.1038/s41586-021-03442-1
75. Damaghi M, West J, Robertson-Tessi M, Xu L, Ferrall-Fairbanks MC, Stewart PA, et al. The Harsh Microenvironment in Early Breast Cancer Selects for a Warburg Phenotype. Proc Natl Acad Sci USA (2021) 118(3):e2011342118. doi: 10.1073/pnas.2011342118
76. Sun C, Li T, Song X, Huang L, Zang Q, Xu J, et al. Spatially Resolved Metabolomics to Discover Tumor-Associated Metabolic Alterations. Proc Natl Acad Sci USA (2019) 116(1):52–7. doi: 10.1073/pnas.1808950116
77. Yuan Z, Zhou Q, Cai L, Pan L, Sun W, Qumu S, et al. SEAM Is a Spatial Single Nuclear Metabolomics Method for Dissecting Tissue Microenvironment. Nat Methods (2021) 18(10):1223–32. doi: 10.1038/s41592-021-01276-3
78. Vistain LF, Tay S. Single-Cell Proteomics. Trends Biochem Sci (2021) 46(8):661–72. doi: 10.1016/j.tibs.2021.01.013
79. Schoof EM, Furtwangler B, Uresin N, Rapin N, Savickas S, Gentil C, et al. Quantitative Single-Cell Proteomics as a Tool to Characterize Cellular Hierarchies. Nat Commun (2021) 12(1):3341. doi: 10.1038/s41467-021-23667-y
80. Specht H, Emmott E, Petelski AA, Huffman RG, Perlman DH, Serra M, et al. Single-Cell Proteomic and Transcriptomic Analysis of Macrophage Heterogeneity Using Scope2. Genome Biol (2021) 22(1):50. doi: 10.1186/s13059-021-02267-5
81. Budnik B, Levy E, Harmange G, Slavov N. SCoPE-MS: Mass Spectrometry of Single Mammalian Cells Quantifies Proteome Heterogeneity During Cell Differentiation. Genome Biol (2018) 19(1):161. doi: 10.1186/s13059-018-1547-5
82. Marx V. A Dream of Single-Cell Proteomics. Nat Methods (2019) 16(9):809–12. doi: 10.1038/s41592-019-0540-6
83. Lundberg E, Borner GHH. Spatial Proteomics: A Powerful Discovery Tool for Cell Biology. Nat Rev Mol Cell Biol (2019) 20(5):285–302. doi: 10.1038/s41580-018-0094-y
Keywords: breast cancer, microenvironment, single-cell RNA sequencing, single-cell omics, heterogeneity
Citation: Tan Z, Kan C, Sun M, Yang F, Wong M, Wang S and Zheng H (2022) Mapping Breast Cancer Microenvironment Through Single-Cell Omics. Front. Immunol. 13:868813. doi: 10.3389/fimmu.2022.868813
Received: 03 February 2022; Accepted: 11 March 2022;
Published: 20 April 2022.
Edited by:
Xia Liu, University of Kentucky, United StatesReviewed by:
Shanhu Li, Beijing Institute of Biotechnology, ChinaBidesh Mahata, University of Cambridge, United Kingdom
Copyright © 2022 Tan, Kan, Sun, Yang, Wong, Wang and Zheng. This is an open-access article distributed under the terms of the Creative Commons Attribution License (CC BY). The use, distribution or reproduction in other forums is permitted, provided the original author(s) and the copyright owner(s) are credited and that the original publication in this journal is cited, in accordance with accepted academic practice. No use, distribution or reproduction is permitted which does not comply with these terms.
*Correspondence: Hong Zheng, zhenghong@ahmu.edu.cn; Siying Wang, sywang@ahmu.edu.cn