- Radiation Oncology, Azienda Ospedaliero-Universitaria Careggi, University of Florence, Florence, Italy
Normal tissue complication probability (NTCP) models that were formulated in the Quantitative Analyses of Normal Tissue Effects in the Clinic (QUANTEC) are one of the pillars in support of everyday’s clinical radiation oncology. Because of steady therapeutic refinements and the availability of cutting-edge technical solutions, the ceiling of organs-at-risk-sparing has been reached for photon-based intensity modulated radiotherapy (IMRT). The possibility to capture heterogeneity of patients and tissues in the prediction of toxicity is still an unmet need in modern radiation therapy. Potentially, a major step towards a wider therapeutic index could be obtained from refined assessment of radiation-induced morbidity at an individual level. The rising integration of quantitative imaging and machine learning applications into radiation oncology workflow offers an unprecedented opportunity to further explore the biologic interplay underlying the normal tissue response to radiation. Based on these premises, in this review we focused on the current-state-of-the-art on the use of radiomics for the prediction of toxicity in the field of head and neck, lung, breast and prostate radiotherapy.
Introduction
The seminal QUANTEC collection (1) provided a comprehensive set of recommendations for the estimation of normal tissue complication probability (NTCP) that were largely based on empirical data, whereas the earlier influential paper by Emami (2) was mainly based on a consensus of experts. Leveraging the available published evidence into definitions of dose-volume relationships for most organs at risk epitomized the paradigm shift of QUANTEC.
However, its analyses relied on data from a time when predominantly 3D-conformal radiotherapy (3DCRT) was used with relatively uniform dose distributions. The advent of intensity modulated radiotherapy (IMRT) led to an unprecedented improvement in radiation ballistics (3), allowing for exquisite precision in dose distribution. Over the years, through constant optimization of IMRT techniques (4), the ceiling of organ-at-risk sparing has been reached: in the frame of photon therapy delivery, incremental advances in the reduction of radiation-induced toxicity are unlikely to occur, mainly due to limits dictated by physics. Ideally, further improvement must come from better shaping the dose distribution, which can only be personally optimized if precise knowledge (5) of dose-effect relationships is used. The current state-of-the-art of relying exclusively on NTCP models from QUANTEC has its own caveats (6): above all, the lack of integration of biologic heterogeneity and patients’ individual factors such as age, comorbidities, pre-existing organ dysfunction, and use of systemic agents represent the most limiting factors. In addition, an overarching issue is represented by the paucity of external validation studies (7, 8) for most NTCP models.
Overall, the absence of predictive biomarkers for radiation-related morbidity is a major unmet need in modern radiation therapy. Within the last 10 years (9, 10), the advent of radiomics has reshaped the approach to medical images, based on the hypothesis that they are inherently able to convey information on the underlying physiopathology. Standardization in image acquisition, high-throughput generation of objective descriptors and extensive data-mining characterized the transition from purely qualitative to quantitative imaging (11). As outlined in the pivotal CRUK-EORTC consensus review (12), distinct features can be envisaged in the re-thinking of imaging as a biomarker: non-invasiveness, serial assessment, comprehensive tumor mapping, repeatability, and cost-effectiveness. In the perspective of personalized oncology (13) as currently implemented in the clinic, the use of quantitative imaging may allow us to overcome the known limits associated with molecular profiling. Several applications of radiomics in the field of precision radiation oncology have been identified, providing insights in terms of stage discrimination (14, 15), molecular stratification (16–18), prognostic impact (19, 20), and prediction of response to treatment (21–23). With imaging, the possibility to capture intrinsic tumor and organ-specific heterogeneity could be leveraged to evaluate the individual predisposition to radiation-induced toxicity (24). Thus, radiomics-based analyses have the potential to enrich standard NTCP models for the definition of individualized risk profiling, ultimately aiming for a personalized patient management and optimized therapeutic ratio. At present, such efforts must still be considered investigational and not ready for prime time (25). The aim of our mini-review was to provide an overview on the evidence pertaining to the role of radiomics in the prediction of radiation-induced toxicity for parotid glands, lungs, heart, and rectum. Based on the aforementioned premises, in each of the following sections an introduction on the traditional QUANTEC-based NTCP models is followed by the description of the most relevant data thus far available on radiomics-analyses and their potential in improving the predictive ability of side effects.
Head and Neck Radiotherapy: Parotid Glands
Xerostomia represents a well known side-effect in head and neck cancer (HNC) radiotherapy (RT), accounting for significant impairment in patients’ quality of life due to its impact on taste, swallowing, and speech (26). The major determinant of xerostomia is radiation-induced damage of the parotid and submandibular glands, which globally release over 80% of saliva (27). The QUANTEC consortium (6, 28) identified a mean parotid gland dose of 26 Gy as a critical threshold for the preservation of salivary function. However, in IMRT clinical practice, it is often challenging to comply with this recommendation, since a detrimental impact on target coverage can’t be completely minimized (29). Furthermore, it has been demonstrated that a late recovery of salivary function is feasible, even in cases of overt xerostomia shortly after RT (30). These considerations led to the assumption that the dose-response relationship of parotid glands is more complex than initially hypothesized in QUANTEC, and that within this context, the use of quantitative imaging could lead to a better understanding of this issue. In an effort to better elucidate radiation-induced xerostomia pathogenesis, van Lujk et al. (31) postulated the existence of stem cell regions in the context of parotid glands involved in the regeneration of salivary function. As the distribution of stem cells within the parotid gland was shown to be inhomogeneous, with the highest concentration located near the dorsal edge of the mandible (where the first branching of the Stensen duct is located), it has been theorized that intentionally sparing these sub-regions would yield better results rather than attempting to spare the whole gland. The validity of this approach was further confirmed by a post hoc analysis of the PARSPORT trial performed by Buettner et al. (32). In fact, by taking into account the spatial information of dose distribution within parotid glands, the authors demonstrated that a significantly better prediction of patient-reported xerostomia could be obtained in respect to a model solely based on standard mean dose. Further efforts in unraveling the complex relationship between dose distribution within the parotid and NTCP led to the concept that different thresholds for xerostomia injury and recovery exist. Recently, Guo et al. (33) assessed the spatial radiation dose-based importance pattern in the major salivary glands in relation to late and acute xerostomia in a retrospective population of 146 HNC patients. The authors identified the superior portion of the two parotid glands (low dose region) as the most influential on xerostomia recovery, and demonstrated a different voxel hierarchy pattern for injury and recovery. In a retrospective analysis on 258 patients, Han and colleagues (34) showed an inverse correlation between the pattern of dose-volume histograms and clinical outcomes: a relatively high dose to small portions of a glandular sub-volume (between 10 and 40%) may be more harmful than a low-dose bath effect. Hence, in terms of function preservation, limiting the dose to specific sub-volumes such as the superior-posterior region of the ipsilateral parotid gland may be more useful (nested cross-validation area under the curve (AUC) – values of 0.78 and 0.70 for prediction of injury and recovery, respectively). In this perspective, the identification of quantitative imaging parameters correlated with both acute and late xerostomia is of paramount importance. Changes over time of radiomics features (delta-radiomics) have been extensively evaluated both in terms of acute and late xerostomia prediction (35–38). In an effort to better elucidate the relationship between parotid gland shrinkage after RT and late xerostomia, van Dijk et al. (37) recently demonstrated a correlation between delta radiomics surface changes in contralateral parotid gland and late xerostomia in 68 patients (AUC 0.93 in test cohort). This association was significant during the whole course of RT, but performed best for mid-treatment (week 3). This finding may have profound clinical implications, allowing an early identification of patients at risk for developing late side effects and prompting adaptive re-planning or even switching to other forms of radiation (e.g., proton therapy). A similar approach was performed by Rosen and colleagues (38), who retrospectively analyzed serial cone-beam CTs (CBCT) of 119 HNC cancer patients undergoing RT. The authors concluded that the rate of CBCT-measured parotid gland image feature changes improved NTCP modeling over dose alone for late xerostomia prediction (AUC 0.77). In the context of late xerostomia prediction, baseline evaluation of changes in magnetic resonance (MR) and 18F-fluorodeoxyglucose (FDG) positron emission tomography/computed tomography (PET-CT)-based parotid gland features was also shown to be a promising field of application (39–43). In particular, parotid glands with low metabolic activity and a low fat-to-functional parenchymal ratio were matched by more heterogeneous intensity and texture imaging features: overall, these hypothesis-generating studies showed that pre-treatment radiomics-based prediction outperformed conventional NTCP models. Finally, a machine-learning approach integrating dosiomics, radiomics, and morphological data in predicting both acute and late injury to salivary glands has recently shown promising results (44, 45). Interestingly, by applying a novel artificial intelligence methodology (“likelihood-fuzzy analysis”), Pota et al. (46) identified quantitative predictors of 12-month toxicity through a longitudinal assessment of parotid glands in a dual institution experience. Taking all data together, radiomics-based analyses proved to be reliable tools to assess the risk for xerostomia in HNC patients, warranting further validation in larger prospective cohorts.
Thoracic Radiotherapy: Lung
Radiation-induced lung injury (RILI) is at the same time a complex radiobiological entity with a multi-faceted physiopathology and a serious challenge for the clinician, representing an important source of morbidity in 15–40% (47, 48) of patients receiving radiation or chemoradiation as definitive treatment for non-small cell lung cancer (NSCLC). In the IMRT era, a stringent trade-off between dose delivery to locoregional disease and adequate sparing of healthy lung tissue is advocated. This assumption was corroborated by a secondary analysis of the controversial RTOG 0617 trial, suggesting that the lack of benefit of dose escalation may have resulted from an increase in cardio-pulmonary mortality in patients receiving more aggressive dose regimens (49, 50). It is well known that RILI is a dose-limiting toxicity in the management of esophageal cancer (51, 52) and lymphoma (53, 54) patients, as well. In view of the usually lower total dose delivered for these malignancies in current practice, the most compelling evidence on radiation-induced lung toxicity can be extrapolated from NSCLC. Hence, it is of primary importance to unravel the intricate network of technical, clinical, and treatment-related factors implicated in the onset of RILI in order to develop models that allow us to accurately predict the risk of serious adverse events. The use of dose estimates to the lung as a predictor of RILI risk is well established (55), while the role of other factors, in particular dose to the heart, is controversial (56–58). Currently, dose-volume parameters, namely the mean lung dose (MLD) and the volume of lungs receiving at least 20 Gy (V20Gy), have been integrated in the QUANTEC (59) as partially reliable surrogates for the risk of radiation pneumonitis. Taking into account the known low dose bath-effect of IMRT, lower dose-volume thresholds have also been suggested, such as V13Gy (60, 61) and V5Gy (54, 62) In comparison with the historical standard Lyman model, the development of the “generalized Lyman-model” (GLM) (63) led to the introduction of a new radiobiological parameter (the effective dose, or Deff, corresponding to the equivalent uniform dose, EUD), allowing for exposed volumes of the organ at risk to be weighted differently. However, dose-volume parameters do not ultimately allow us to take into account the functional heterogeneity within different lung regions and among individuals. On the other hand, data extraction from pre-treatment imaging may provide information for a tailored strategy. Thus far, a few reports are available on the potential added value of radiomics in the context of RILI prediction. In a single-center, retrospective experience on 96 patients who received curative RT for esophageal cancer, Anthony et al. (64) evaluated the correlation between the development of symptomatic radiation pneumonitis and pre-treatment analysis of FDG PET/CT and diagnostic CT scans. In a logistic regression model, the addition of the standard uptake value (SUV) standard deviation to 18 lung CT texture feature changes in the low-dose area (0–10 Gy) improved by 0.08 the mean AUC value in discriminating the diagnosis of RILI. In a larger experience on 192 patients treated for NSCLC in the same institution, Krafft et al. (65) extracted 6851 features from planning CT scans, as candidate predictors for RILI. Compared with standard clinical and dosimetric factors, at least absolute shrinkage and selection operator (LASSO) logistic regression, a final 449-feature set of the total lung volume yielded a higher average cross-validated AUC, demonstrating improved discrimination (0.51 and 0.68, respectively). The existence of a strict relationship between the dose distribution, a change of CT texture features before and after RT, and the risk of RILI development was firstly demonstrated by Cunliffe et al. (66). Recently, this dosiomic approach was replicated through a convolutional deep-neural network analysis (67, 68) in a cohort of 70 NSCLC patients treated with volumetric modulated arc therapy (VMAT), providing a high discriminative power (AUC of 0.84) over standard logistic regression models for the prediction of radiation pneumonitis. Taking into account the much less clinically relevant impact of radiation pneumonitis in the context of stereotactic body RT, limited data are available (69, 70) in this context in comparison to conventionally-fractionated regimens. Overall, in parallel to robust prognostic value in the context of stereotactic body RT (71) and chemoradiation (72), the reported data promisingly support the relevance of radiomics in the prediction of lung toxicity. However, to take into account the complexity of RILI, optimal models should integrate, in addition to dosimetric variables, other individual risk factors such as age (73), genetic polymorphysms (74), pre-existing functional impairment of the lung (48), chemotherapy regimens (75), and, curiously, a paradoxical protective effect of smoking as a possible result of functional exhaustion of the inflammatory microenvironment in current smokers (73). In summary, RILI is a multi-faceted phenomenon resulting from complex processes that depend on biologic, dosimetric, and treatment-related variables that need to be integrated in a comprehensive model (76, 77), beyond a mechanicistic dose-response relationship.
Breast Radiotherapy: Heart
Radiotherapy plays a crucial role in the curative management of non-metastatic breast cancer, with well-established benefits in terms of loco-regional control and survival for node-positive patients (78, 79). In 2005, the Early Breast Cancer Trialists’ Collaborative Group (EBCTCG) meta-analysis on individual patient data epitomized the known potential correlation of radiation and cardiac damage, showing a significant excess of non-breast cancer mortality from heart disease (rate ratio 1.27, SE 0.07, 2p = 0.0001) (80). Notably, the high cure rate of radiation for Hodgkin lymphoma (HL) has been historically offset by late heart dysfunction in long survivors (81). In the QUANTEC publication (82) it was recommended that the heart volume receiving up to 25 Gy (V25) should be below 10%. In current practice, the “ALARA” (“as low as reasonably achievable”) principle is usually applied to left-sided breast cancer patients, aiming for a mean heart dose (MHD) below 2 Gy whenever possible. However, the NTCP model does not take into account other dosimetric factors, such as the possible interaction between cardiac and lung dose-volume parameters (83), as suggested by Cella et al. in an institutional analysis on 90 HL patients (84). Abnormalities in myocardial perfusion and echocardiography have been reported (85) when larger than average heart volumes were inadvertently irradiated. In particular, a mean dose to the left ventricle of 9 + 4 Gy was significantly correlated with a reduced anterior wall strain (-16.8% at 14 months after RT), an early surrogate marker of myocardial function detectable with doppler echocardiographic imaging. Conversely, in patients with relatively low MHD (< 4 Gy), Bian et al. found no association between cardiac dosimetry and left ventricular ejection fraction (LVEF) (86). Multiple heart dose parameters have been associated with clinically relevant cardiotoxicity in breast cancer (87). At a median follow-up of 12 years, Correa et al. found an increased incidence of coronary artery disease and chronic heart failure (CHF) rates for increasing heart dose (85). Likewise, Saiki et al. found a significant association between MHD and the risk of heart failure with preserved ejection fraction (OR: 16.9, 95% CI: 3.9–73.7) (88). In a pivotal study, Darby et al. were able to demonstrate the existence of a linear relationship between the occurrence of major coronary events and MHD, with a 7.4% increase in the risk per Gy (95% CI: 2.9–14.5; p < 0.001). Nonetheless, a distinct dose threshold could not be identified (89). In a large cohort of 910 patients, Van den Bogaard et al. confirmed these findings, reporting a 16.5% increase per Gy in the cumulative incidence of acute coronary events (90), although they were not able to detect a correlation between RT dose and LVEF (91). Overall, the inter-individual heterogeneity in cardiac exposure to radiation has been an unresolved issue in cardiotoxicity studies. The inter-observer reproducibility in delineation of heart substructures and their dosimetric evaluation (82) are critical factors for a prospective, personalized risk assessment. Indeed, contouring standardization may have a significant role in minimizing differences in dose reporting (92–95). Patients enrolled in the prospective BACCARAT study (96) underwent a coronary computed tomography angiography (CCTA) before irradiation. By analyzing the dose distribution to the whole heart and its substructures in 89 left-sided subjects, the authors highlighted that MHD is a poor dosimetric surrogate parameter for the left ventricle and coronary arteries (in particular the left anterior descending artery). A machine learning approach based on CCTA-derived radiomics may have potential for a better prediction of atherosclerotic plaques over visual assessment (AUC of 0.73 vs 0.65, p = 0.04) (97). Taking all clinical observations together, no NTCP modeling provides conclusive evidence on late heart toxicity based on MHD analysis. To the best of our knowledge, no radiomics applications have been reported for the prediction of radiation-induced heart damage. Interestingly, Currie et al. (98) performed an explorative study based on automated feature extraction from single-photon emission computed tomography (SPECT) imaging in 22 non-cancer patients with cardiomyopathy to evaluate the most potent prognostic index for future cardiac events. With an artificial neural network approach, the authors showed that a 23iodine meta-iodobenzylguanidine (123I-mIBG) planar global washout higher than 30% was the best indicator for risk of cardiac events when accompanied by a decline in LVEF of more than 10%. In summary, in spite of technical capability of modern IMRT techniques to tightly refine the dose distribution within the thorax, the definition of dose-volume relationships and specific NTCP modeling for myocardial sub-volumes lags behind. Taking into account that the risk of future cardiac events after RT is strongly related to persistent smoking, age, prior cardiac events, and pre-existing cardiovascular risk factors, big data applications (99) may lend support to clinical decision making.
Prostate Radiotherapy: Rectum
Definitive RT represents one of the main treatment options for localized prostate cancer (100). Thanks to the availability of long-term data on clinical outcome and adverse events, radiation-induced lower gastro-intestinal toxicity remains one of the most relevant factors known to have a detrimental impact on patients’ quality of life (101). The relationship between increased late rectal toxicity and high radiation dose is well known for 3DCRT (102) and conventional fractionation up to 78 Gy, with increasing rates of bleeding with rectal volumes receiving 50, 60, 65, 70, and 75 Gy greater than 50, 35, 25, 20, and 15%, respectively (V50Gy > 50%, V60Gy > 35%, V65Gy > 25%, V70Gy > 20%, and V75Gy > 15%) (103, 104). When externally validated in patients treated with 3DCRT, the QUANTEC-based EUD model had relatively low predictive power (AUC 0.61) for late rectal bleeding (105). Further, the NTCP cross-applicability to IMRT for chronic gastrointestinal toxicity was assessed in a large single-institution cohort study (106). Indeed, debilitating symptoms such as fecal incontinence or rectal urgency were mostly reported when large volumes of the rectum were exposed to intermediate doses, as confirmed by the Medical Research Council RT01 randomized phase 3 trial (107) and the long-term follow-up of the AIROPROS 0102 study (108). In recent years, the implementation of moderate hypo-fractionated regimens in clinical practice prompted the development of dose-volume constraints adapted to different treatment schedules (109). Unlike what happens for moderate hypo-fractionated IMRT, high rather than low-dose regions in the rectum predict toxicity after an ultra hypo-fractionated regimen. Of note, V35Gy was shown to be a strong predictor of rectal bleeding (110) and a recent pooled analysis of patients treated within four different trials demonstrated that late toxicity and quality of life were significantly related to V38Gy after the delivery of 35-40 Gy in five fractions (111). Overall, prospectively defined dosimetric predictors of lower gastro-intestinal toxicity can be adapted according to different techniques and fractionations used in the context of definitive treatment for localized prostate cancer.
In view of the available spectrum of NTCP models and of the clinical variability of late rectal side effects, extracting mineable data from imaging would facilitate a personalized treatment prescription. Few radiomics analyses allow us to refine the toxicity prediction in the current scenario. In a single-center prospective study on 33 patients treated with moderately accelerated IMRT (70.2 Gy in 26 fractions), Abdollahi et al. (112) performed a machine learning approach on pre- and post-treatment T2-weighted MR scans of the rectal wall. Out of a total of 1096 features, a 37-set of descriptors extracted from baseline T2-weighted images was more accurate (mean AUC of 0.68) than post-treatment T2-weighted apparent diffusion coefficient (ADC) and delta values. Of note, a broad clinical endpoint was chosen by the authors (G1 rectal toxicity, occurring in 54% of the cohort). Similar pilot analyses from the same group focused on the bladder wall (113) and femoral head changes (114). In a secondary analysis of the multi-institutional randomized HYPRO trial, Rossi et al. (115) evaluated the correlation of late gastrointestinal and genitourinary toxicity with non-treatment related characteristics (age, baseline PSA, Gleason score, comorbidities), DVH parameters, and radiomics features. Of the 820 patients with intermediate and high risk prostate cancer enrolled in the trial, 351 had dose distributions to rectum and bladder available for 3D texture analysis. For both rectal bleeding and fecal incontinence, logistic NTCP models showed that the addition of texture features led to a statistically significant improvement in the predictive ability (AUC of 0.73 for both; p < 0.04), higher than what was obtained with clinical and DVH parameters. In a smaller prospective study on 64 patients, Mostafaei et al. came to similar results by analyzing baseline CT markers with a stacking regression algorithm (116). Interestingly, an explorative approach focused on four patients irradiated on a 1.5 Tesla MR-Linear Accelerator within a prospective observational trial. Delta-radiomics assessed with a longitudinal T2-weighted intensity histogram of prostate and surrounding organs at risk showed early significant variations of the rectal wall, with change in mean, median, and standard deviation metrics values at the second week of treatment. A longitudinal radiomic data acquisition process was deemed feasible on the hybrid machine (117). To summarize, in the modern context of prostate RT, the prediction of gastrointestinal toxicity based only on NTCP models may be misleading, given the current trend for dose-escalated IMRT and the establishment of hypo-fractionated and ultra hypo-fractionated regimens as standards of care. Early prospective data on the integration of radiomics analyses are available. Potentially, these features may represent a valuable tool for clinical decision in the future. Further refinement could be provided by applying machine learning methods and bioinformatics tools to genome-wide data to identify patients with a greater congenital risk of toxicity before treatment (118).
Assessing the Quality of Radiomics Investigations: A Word of Caution
In the previous sections, the potential of radiomics for the prediction of radiation-induced toxicity for parotid glands, lung, heart, and rectum was highlighted. Promisingly, quantitative imaging represents an area of active research under the light of precision oncology (25). Nonetheless, when evaluating the investigations thus far published on radiomics, some caveats need to be taken into account. In view of the complexity of the radiomic workflow, Lambin and colleagues (11) introduced a radiomics quality score (RQS) tool. Based on a set of 16 well-defined criteria addressing several aspects such as image protocol quality, segmentation method, feature reduction, presence of biologic correlates, and extent of validation, the authors proposed to define an objective ranking of quality for radiomics studies. In particular, a score of 36 corresponds to the highest value achievable, whereby the prospective validation of a radiomics signature in a registered trial confers the largest contribution (7 points). Through a systematic review of the literature focusing on the link between radiomic biomarkers and tumor biology, Sanduleanu et al. (119) applied the RQS in 41 studies. Unsurprisingly, most studies (30/41) were of poor quality, with an average score of 30% or less, mainly because of a lack of robust segmentation, external validation, and discrimination based on cut-off values. In addition, interobserver variability among authors in terms of scoring was significant, suggesting that the proposed scale requires further refinement. When applying the RQS to evaluate the methodological quality of the most relevant radiomics analyses thus far published for the prediction of xerostomia, RILI, and late rectal toxicity, the overall outlook (Tables 1, 2) is unsatisfactory. Although all studies performed well in terms of describing feature reduction methods (all used measures to decrease the risk of overfitting), multivariable analyses with non-radiomic factors, and reporting cut-off analyses, the weaknesses are represented by the limited validation (typically, on a dataset from the same institution), the retrospective study design, the infrequent discussion of biological correlates, and the lack of cost-effectiveness. A notable exception is represented by the work of Rossi et al. (112) with a RQS of 80.5% (29/36): the high score can be justified due to the fact that the radiomics signature in this study was prospectively validated in a large, multi-institutional randomized trial with a resulting potential direct clinical utility. In view of the suboptimal methodological quality frequently observed in the radiomics studies we evaluated, caution is advised in the interpretation of the reported findings. Another relevant limit to bear in mind is the lack of standardization in regards to imaging features definition and interpretation. In this perspective, the recently published Image Biomarker Standardization Initiative (IBSI) position paper (120) should be viewed as a relevant step ahead, fostering homogeneity in radiomics analyses across different research platforms.
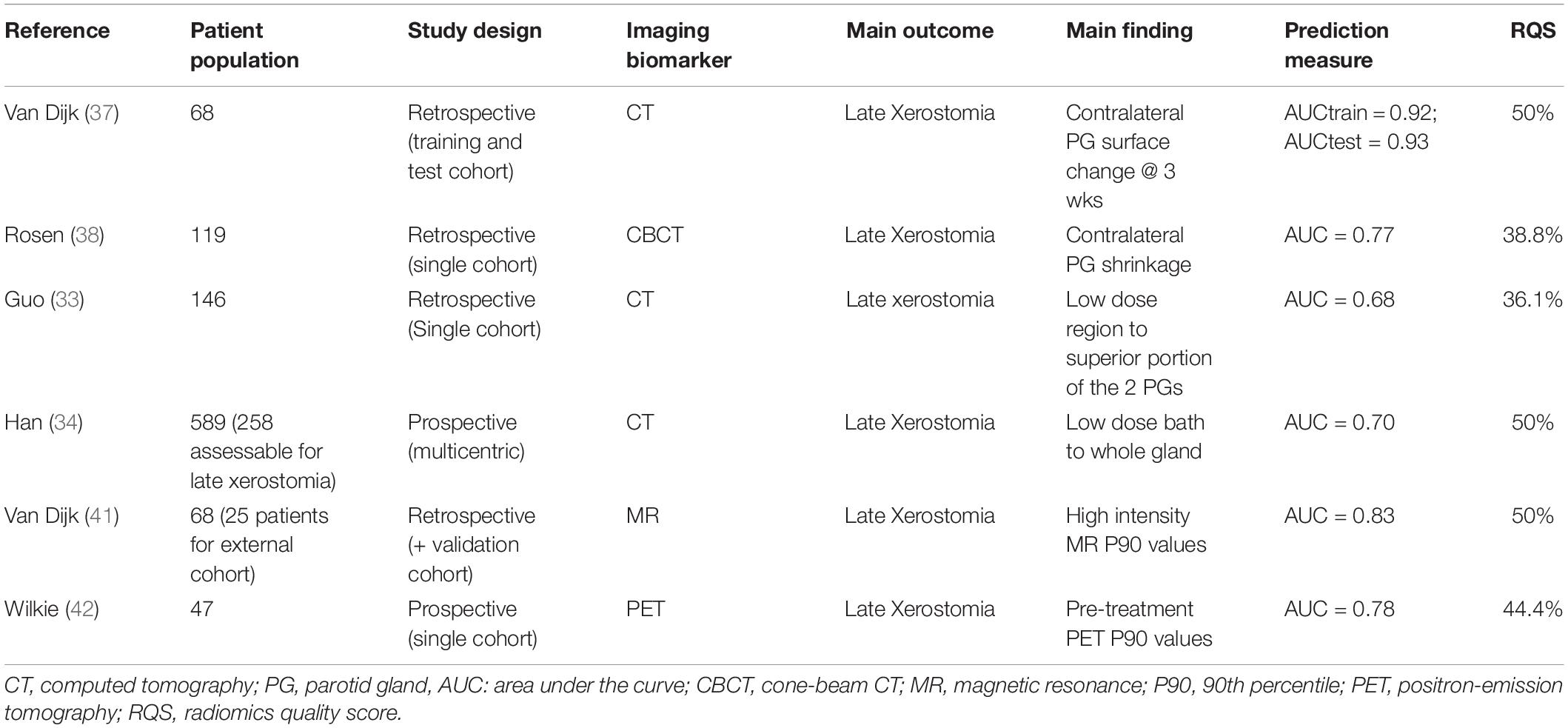
Table 1. Most consolidated data in radiomics-based approaches in predicting radiotherapy-induced xerostomia.
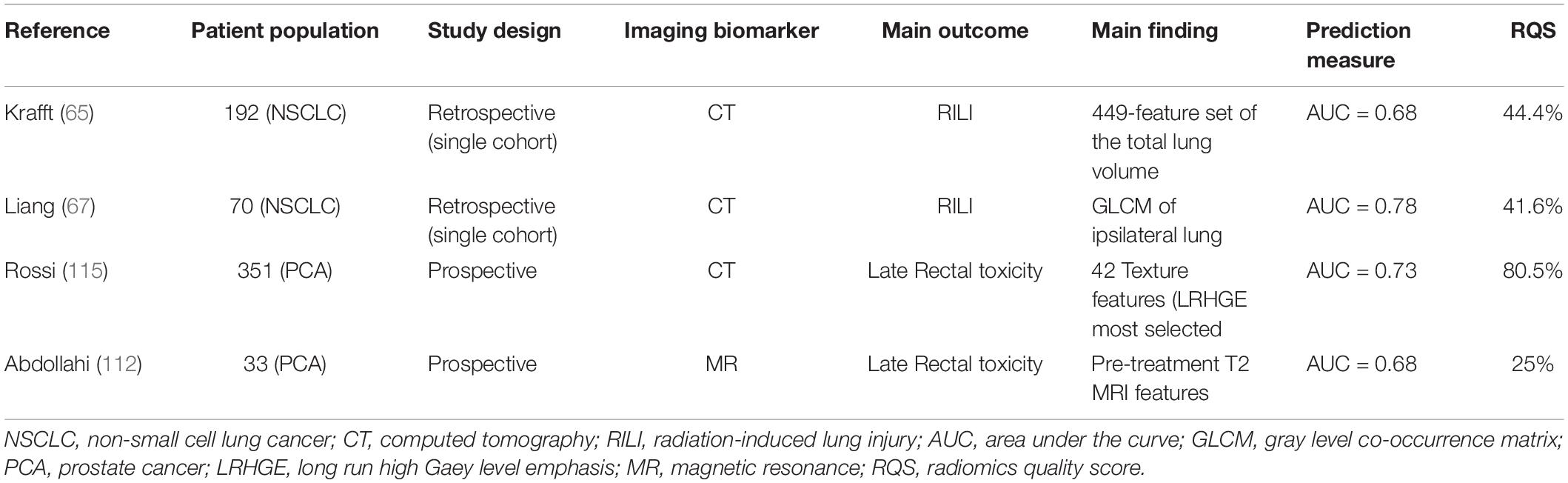
Table 2. Most promising data in radiomics-based approaches in predicting radiotherapy-induced toxicity in the treatment of solid tumors.
Conclusion
In comparison to efficacy outcomes, the current state-of-the-art on radiomics prediction of radiation-induced toxicity is still relatively limited, with the notable exception of xerostomia prognostication (Tables 1, 2). Taking all data together, the vast majority of reviewed studies suggested that indeed radiomics applications may increase the predictive ability of organ-specific side effects over standard clinical and dosimetric factors. For further progress, four major areas of improvement can be envisaged. Firstly, the need for standardization is a critical, well-recognized major step for further development (120, 121). Secondly, in view of the frequent single-center retrospective design and the generally low number of enrolled patients and of clinical endpoints (i.e., side effects), the robustness of data is questionable for most studies (122). In this perspective, the lack of or very limited external validation in independent datasets is a point of weakness for both conventional NTCP models (123) and radiomics applications (119). Thirdly, progress in the field of radio-genomics is eagerly awaited (124), in order to improve the understanding of underlying biological processes, such as intrinsic radio-sensitivity. Lastly, controlled randomized clinical trials testing radiomics-based interventions in adequately powered studies are still yet to be published. At present, no single radiomics finding is readily applicable to patient management in clinical practice. Nonetheless, the available body of evidence is encouraging and warrants further investigation, given the size of benefit demonstrated in terms of high predictive ability of common toxicities. In conclusion, building on established NTCP models, the so far available hypothesis-generating data underline the potential of radiomics for improved clinical decision making in precision radiation oncology.
Author Contributions
All authors listed have made a substantial, direct and intellectual contribution to the work, and approved it for publication.
Conflict of Interest
The authors declare that the research was conducted in the absence of any commercial or financial relationships that could be construed as a potential conflict of interest.
References
1. Marks LB, Ten Haken RK, Martel MK. Guest editor’s introduction to QUANTEC: a users guide. Int J Radiat Oncol Biol Phys. (2010) 76(3 Suppl.):S1–2. doi: 10.1016/j.ijrobp.2009.08.075
2. Emami B, Lyman J, Brown A, Coia L, Goitein M, Munzenrider JE, et al. Tolerance of normal tissue to therapeutic irradiation. Int J Radiat Oncol Biol Phys. (1991) 21:109–22.
3. Ling CC, Yorke E, Fuks Z. From IMRT to IGRT: frontierland or neverland? Radiother Oncol. (2006) 78:119–22. doi: 10.1016/j.radonc.2005.12.005
4. Bentzen SM. Theragnostic imaging for radiation oncology: dose-painting by numbers. Lancet Oncol. (2005) 6:112–7. doi: 10.1016/S1470-2045(05)01737-7
5. Baumann M, Krause M, Overgaard J, Debus J, Bentzen SM, Daartz J, et al. Radiation oncology in the era of precision medicine. Nat Rev Cancer. (2016) 16:234–49. doi: 10.1038/nrc.2016.18
6. Bentzen SM, Constine LS, Deasy JO, Eisbruch A, Jackson A, Marks LB, et al. Quantitative analyses of normal tissue effects in the clinic(QUANTEC): an introduction to the scientific issues. Int J Radiat Oncol Biol Phys. (2010) 76(3 Suppl.):S3–9. doi: 10.1016/j.ijrobp.2009.09.040
7. Collins GS, De Groot JA, Dutton S, Omar O, Shanyinde M, Tajar A, et al. External validation of multivariable prediction models: a systematic review of methodological conduct and reporting. BMC Med Res Methodol. (2014) 14:40. doi: 10.1186/1471-2288-14-40
8. Collins GS, Reitsma JB, Altman DG, Moons KG. Transparent reporting of a multivariable prediction model for individual prognosis or diagnosis (TRIPOD): the TRIPOD statement. Br J Cancer. (2015) 112:251–9. doi: 10.1038/bjc.2014.639
9. Lambin P, Rios-Velazquez E, Leijenaar R, Carvalho S, van Stiphout RG, Granton P, et al. Radiomics: extracting more information from medical images using advanced feature analysis. Eur J Cancer. (2012) 48:441–6. doi: 10.1016/j.ejca.2011.11.036
10. Gillies RJ, Kinahan PE, Hricak H. Radiomics: images are more than pictures, they are data. Radiology. (2016) 278:563–77. doi: 10.1148/radiol.2015151169
11. Lambin P, Leijenaar RTH, Deist TM, Peerlings J, de Jong EEC, van Timmeren J, et al. Radiomics: the bridge between medical imaging and personalized medicine. Nat Rev Clin Oncol. (2017) 14:749–62. doi: 10.10138/nrclinonc.2017.141
12. O’Connor JP, Aboagye EO, Adams JE, Aerts HJWL, Barrington SF, Beer AJ, et al. Imaging biomarker roadmap for cancer studies. Nat Rev Clin Oncol. (2017) 14:169–86. doi: 10.1038/nrclinonc.2016.162
13. Tannock IF, Hickman JA. Limits to personalized cancer medicine. N Engl J Med. (2016) 375:1289–94. doi: 10.1056/NEJMsb1607705
14. Liang C, Huang Y, He L, Chen X, Ma X, Dong D, et al. The development and validation of a CT-based radiomics signature for the preoperative discrimination of stage I-II and stage III-IV colorectal cancer. Oncotarget. (2016) 7:31401–12. doi: 10.18632/oncotarget.8919
15. Ren J, Tian J, Yuan Y, Dong D, Li X, Shi Y, et al. Magnetic resonance imaging based radiomics signature for the preoperative discrimination of stage I-II and III-IV head and neck squamous cell carcinoma. Eur J Radiol. (2018) 106:1–6. doi: 10.1016/j.ejrad.2018.07.002
16. Kniep HC, Madesta F, Schneider T, Hanning U, Schoenfeld MH, Schoen G, et al. Radiomics of brain MRI: utility in prediction of metastatic tumor type. Radiology. (2019) 290:479–87. doi: 10.1148/radiol.2018180946
17. Peeken JC, Bernhofer M, Spraker MB, Pfeiffer D, Devecka M, Thamer A, et al. CT-based radiomic features predict tumor grading and have prognostic value in patients with soft tissue sarcomas treated with neoadjuvant radiation therapy. Radiother Oncol. (2019) 135:187–96. doi: 10.1016/j.radonc.2019.01.004
18. Bogowicz M, Jochems A, Deist TM, Tanadini-Lang S, Huang SH, Chan B, et al. Privacy-preserving distributed learning of radiomics to predict overall survival and HPV status in head and neck cancer. Sci Rep. (2020) 10:4542. doi: 10.1038/s41598020-61297-4
19. Aerts HJ, Velazquez ER, Leijenaar RT, Parmar C, Grossmann P, Carvalho S, et al. Decoding tumour phenotype by noninvasive imaging using a quantitative radiomics approach. Nat Commun. (2014) 5:4006. doi: 10.1038/ncomms5006
20. Krarup MMK, Nygård L, Vogelius IR, Andersen FL, Cook G, Goh V, et al. Heterogeneity in tumours: validating the use of radiomic features on 18F-FDG PET/CT scans of lung cancer patients as a prognostic tool. Radiother Oncol. (2020) 144:72–8. doi: 10.1016/j.radonc.2019.10.012
21. Sun R, Limkin EJ, Vakalopoulou M, Dercle L, Champiat S, Han SR, et al. A radiomics approach to assess tumour-infiltrating CD8 cells and response to anti-PD-1 or anti-PD-L1 immunotherapy: an imaging biomarker, retrospective multicohort study. Lancet Oncol. (2018) 19:1180–91. doi: 10.1016/S1470-2045(18)30413-3
22. Bulens P, Couwenberg A, Intven M, Debucquoy A, Vandecaveye V, Van Cutsem E, et al. Predicting the tumor response to chemoradiotherapy for rectal cancer: model development and external validation using MRI radiomics. Radiother Oncol. (2020) 142:246–52. doi: 10.1016/j.radonc.2019.07.033
23. Cao Q, Li Y, Li Z, An D, Lin Q. Development and validation of a radiomics signature on differentially expressed features of 18F-FDG PET to predict treatment response of concurrent chemoradiotherapy in thoracic esophagus squamous cell carcinoma. Radiother Oncol. (2020) 146:9–15. doi: 10.1016/j.radonc.2020.01.027
24. Gardin I, Gregoire V, Gibon D, Kirisli H, Pasquier D, Thariat J, et al. Radiomics: principles and radiotherapy applications. Crit Rev Oncol Hematol. (2019) 138:44–50. doi: 10.1016/j.critrevonc.2019.03.015
25. Jaffray DA, Das S, Jacobs PM, Jerai R, Lambin P. How advances in imaging will affect precision radiation oncology. Int J Radiat Oncol Biol Phys. (2018) 101:292–8. doi: 10.1016/j.ijrobp.2018.01.047
26. Jellema AP, Slotman BJ, Doornaert P, Leemans CR, Langendijk JA. Impact of radiation-induced xerostomia on quality of life after primary radiotherapy among patients with head and neck cancer. Int J Radiat Oncol Biol Phys. (2007) 69:751–60.
27. Ortholan C, Benezery K, Bensadoun RJ. Normal tissue tolerance to external beam radiation therapy: salivary glands. Cancer Radiother. (2010) 14:290–4. doi: 10.1016/j.canrad.2010.03.007
28. Deasy JO, Moiseenko V, Marks L, Chao KS, Nam J, Eisbruch A. Radiotherapy dose-volume effects on salivary gland function. Int J Radiat Oncol Biol Phys. (2010) 76(3 Suppl.):S58–63. doi: 10.1016/j.ijrobp.2009.06.090
29. Kreps S, Berges O, Belin L, Zefkili S, Petras S, Giraud P. Salivary gland-sparing helical tomotherapy for head and neck cancer: preserved salivary function on quantitative salivary gland scintigraphy after tomotherapy. Eur Ann Otorhinolaryngol Head Neck Dis. (2016) 133:257–62. doi: 10.1016/j.anorl.2016.05.003
30. Owosho AA, Thor M, Oh JH, Riaz N, Tsai CJ, Rosenberg H, et al. The role of parotid gland irradiation in the development of severe hyposalivation (xerostomia) after intensity-modulated radiation therapy for head and neck cancer: temporal patterns, risk factors, and testing the QUANTEC guidelines. J Craniomaxillofac Surg. (2017) 45:595–600. doi: 10.1016/j.jcms.2017.01.020
31. van Luijk P, Pringle S, Deasy JO, Moiseenko VV, Faber H, Hovan A, et al. Sparing the region of the salivary gland containing stem cells preserves saliva production after radiotherapy for head and neck cancer. Sci Transl Med. (2015) 7:305ra147. doi: 10.1126/scitranslmed.aac4441
32. Buettner F, Miah AB, Gulliford SL, Hall M, Harrington KJ, Webb S, et al. Novel approaches to improve the therapeutic index of head and neck radiotherapy: an analysis of data from the PARSPORT randomised phase III trial. Radiother Oncol. (2012) 103:82–7. doi: 10.1016/j.radonc.2012.02.006
33. Guo Y, Jiang W, Lakshminarayanan P, Han P, Cheng Z, Bowers M, et al. Spatial radiation dose influence on xerostomia recovery and its comparison to acute incidence in patients with head and neck cancer. Adv Radiat Oncol. (2019) 5:221–30. doi: 10.1016/j.adro.2019.08.009
34. Han P, Lakshminarayanan P, Jiang W, Shpitser I, Hui X, Lee SH, et al. Dose/Volume histogram patterns in salivary gland subvolumes influence xerostomia injury and recovery. Sci Rep. (2019) 9:3616. doi: 10.1038/s41598-019-40228-y
35. Wu H, Chen X, Yang X, Tao Y, Xia Y, Deng X, et al. Early prediction of acute xerostomia during radiation therapy for head and neck cancer based on texture analysis of daily CT. Int J Radiat Oncol Biol Phys. (2018) 102:1308–18. doi: 10.1016/j.ijrobp.2018.04.059
36. Liu Y, Shi H, Huang S, Chen X, Zhou H, Chang H, et al. Early prediction of acute xerostomia during radiation therapy for nasopharyngeal cancer based on delta radiomics from CT images. Quant Imaging Med Surg. (2019) 9:1288–302. doi: 10.21037/qims.2019.07.08
37. van Dijk LV, Langendijk JA, Zhai TT, Vedelaar TA, Noordzij W, Steenbakkers RJHM, et al. Delta-radiomics features during radiotherapy improve the prediction of late xerostomia. Sci Rep. (2019) 9:12483. doi: 10.1038/s41598-019-48184-3
38. Rosen BS, Hawkins PG, Polan DF, Balter JM, Brock KK, Kamp JD, et al. Early changes in serial CBCT-measured parotid gland biomarkers predict chronic xerostomia after head and neck radiation therapy. Int J Radiat Oncol Biol Phys. (2018) 102:1319–29. doi: 10.1016/j.ijrobp.2018.06.048
39. Sheikh K, Lee SH, Cheng Z, Lakshminarayanan P, Peng L, Han P, et al. Predicting acute radiation induced xerostomia in head and neck Cancer using MR and CT radiomics of parotid and submandibular glands. Radiat Oncol. (2019) 14:131. doi: 10.1186/s13014-019-1339-4
40. Van Dijk LV, Noordzij W, Brouwer CL, Boellard R, Burgerhof JGM, Langendijk JA, et al. 18F-FDG PET image biomarkers improve prediction of late radiation-induced xerostomia. Radiother Oncol. (2018) 126:89–95. doi: 10.1016/j.radonc.2017.08.024
41. van Dijk LV, Thor M, Steenbakkers RJHM, Apte A, Zhai TT, Borra R, et al. Parotid gland fat related magnetic resonance image biomarkers improve prediction of late radiation-induced xerostomia. Radiother Oncol. (2018) 128:459–66. doi: 10.1016/j.radonc.2018.06.012
42. Wilkie JR, Mierzwa ML, Casper KA, Mayo CS, Schipper MJ, Eisbruch A, et al. Predicting late radiation-induced xerostomia with parotid gland PET biomarkers and dose metrics. Radiother Oncol. (2020) 148:30–7. doi: 10.1016/j.radonc.2020.03.037
43. Klein Nulent TJW, Valstar MH, de Keizer B, Willems S, Smit LA, Al-Mamgani A, et al. Physiologic distribution of PSMA-ligand in salivary glands and seromucous glands of the head and neck on PET/CT. Oral Surg Oral Med Oral Pathol Oral Radiol. (2018) 125:478–86. doi: 10.1016/j.oooo.2018.01.011
44. Gabryś HS, Buettner F, Sterzing F, Hauswald H, Bangert M. Design and selection of machine learning methods using radiomics and dosiomics for normal tissue complication probability modeling of xerostomia. Front Oncol. (2018) 8:35. doi: 10.3389/fonc.2018.00035
45. Jiang W, Lakshminarayanan P, Hui X, Han P, Cheng Z, Bowers M, et al. Machine learning methods uncover radiomorphologic dose patterns in salivary glands that predict xerostomia in patients with head and neck cancer. Adv Radiat Oncol. (2018) 4:401–12. doi: 10.1016/j.adro.2018.11.008
46. Pota M, Scalco E, Sanguineti G, Farneti A, Cattaneo GM, Rizzo G, et al. Early prediciton of radiotherapy-induced parotid shrinkage and toxicity based on CT radiomics and fuzzy classification. Artif Intell Med. (2017) 81:41–53. doi: 10.1016/j.artmed.2017.03.004
47. Madani I, De Ruyck K, Goeminne H, De Neve W, Thierens H, Van Meerbeeck J. Predicting risk of radiation-induced lung injury. J Thorac Oncol. (2007) 2:864–74. doi: 10.1097/JTO.0b013e318145b2c6
48. Palma DA, Senan S, Tsujino K, Barriger RB, Rengan R, Moreno M, et al. Predicting radiation pneumonitis after chemoradiation therapy for lung cancer: an international individual patient data meta-analysis. Int J Radiat Oncol Biol Phys. (2013) 85:444–50. doi: 10.1016/j.ijrobp.2012.04.043
49. Hong JC, Salama JK. Dose escalation for unresectable locally advanced non-small cell lung cancer: end of the line? Transl Lung Cancer Res. (2016) 5:126–33. doi: 10.3978/j.issn.2218-6751.2016.01.07
50. Chun SG, Hu C, Choy H, Komaki RU, Timmerman RD, Schild SE, et al. Impact of intensity-modulated radiation therapy technique for locally advanced non-small-cell lung cancer: a secondary analysis of the NRG Oncology RTOG 0617 randomized clinical trial. J Clin Oncol. (2017) 35:56–62. doi: 10.1200/JCO.2016.69.1378
51. Nguyen NP, Krafft SP, Vin-Hung V, Vos P, Almeida F, Jang S, et al. Feasibility of tomotherapy to reduce normal lung and cardiac toxicity for distal esophageal cancer compared to three-dimensional radiotherapy. Radiother Oncol. (2011) 101:438–42. doi: 10.1016/j.radonc.2011.07.015
52. Niezink AGH, de Jong RA, Muijs CT, Langendijk JA, Widder J. Pulmonary function changes after radiotherapy for lung or esophageal cancer: a systematic review focusing on dose-volume parameters. Oncologist. (2017) 22:1257–64. doi: 10.1634/theoncologist.2016.0324
53. Koh ES, Sun A, Tran TH, Tsang R, Pintilie M, Hodgson DC, et al. Clinical dose-volume histogram analysis in predicting radiation pneumonitis in Hodgkin’s lymphoma. Int J Radiat Oncol Biol Phys. (2006) 66:223–8.
54. Pinnix CC, Smith GL, Milgrom S, Osborne EM, Reddy JP, Akhtari M, et al. Predictors of radiation pneumonitis in patients receiving intensity modulated radiation therapy for Hodgkin and non-Hodgkin lymphoma. Int J Radiat Oncol Biol Phys. (2015) 92:175–82. doi: 10.1016/j.ijrobp.2015.02.010
55. Barriger RB, Fakiris AJ, Hanna N, Yu M, Mantravadi P, McGarry RC. Dose-volume analysis of radiation pneumonitis in non-small-cell lung cancer patients treated with concurrent cisplatinum and etoposide with or without consolidation paclitaxel. Int J Radiat Oncol Biol Phys. (2010) 78:1381–6. doi: 10.1016/j.ijrobp.2009.09.030
56. Huang EX, Hope AJ, Lindsay PE, Trovo M, El Naqa I, Deasy O, et al. Heart irradiation as a risk factor for radiation pneumonitis. Acta Oncol. (2011) 50:51–60. doi: 10.3109/0284186X.2010.521192
57. Tucker SL, Liao Z, Dinh J, Bian SX, Mohan R, Martel MK, et al. Is there an impact of heart exposure on the incidence of radiation pneumonitis? Analysis of data from a large clinical cohort. Acta Oncol. (2014) 53:590–6. doi: 10.3109/0284186X.2013.831185
58. Wijsman R, Dankers FJWM, Troost EGC, Hoffmann A, van der Heijden EHFM, de Geus-Oei LF, et al. Inclusion of incidental radiation dose to the cardiac atria and ventricles does not improve the prediction of radiation pneumonitis in advanced-stage non-small cell lung cancer patients treated with intensity modulated radiation therapy. Int J Radiat Oncol Biol Phys. (2017) 99:434–41. doi: 10.1016/j.ijrobp.2017.04.011
59. Marks LB, Bentzen SM, Deasy JO, Spring Kong FM, Bradley JD, Vogelius IS, et al. Radiation dose-volume effects in the lung. Int J Radiat Oncol Biol Phys. (2010) 76(3 Suppl.):S70–6. doi: 10.1016/j.ijrobp.2009.06.091
60. Seppenwoolde Y, Lebesque JV, de Jaeger K, Belderbos JSA, Boersma LJ, Schilstra C, et al. Comparing different NTCP models that predict the incidence of radiation pneumonitis. Normal tissue complication probability. Int J Radiat Oncol Biol Phys. (2003) 55:724–35.
61. Schallenkamp JM, Miller RC, Brinkmann DH, Foote T, Garces Y. Incidence of radiation pneumonitis after thoracic irradiation: dose-volume correlates. Int J Radiat Oncol Biol Phys. (2007) 1:724–35.
62. Wang D, Li B, Wang Z, Zhu J, Sun H, Zhang J, et al. Functional dose-volume histograms for predicting radiation pneumonitis in locally advanced non-small cell lung cancer treated with late-course accelerated hyperfractionated radiotherapy. Exp Ther Med. (2011) 2:1017–22.
63. Tucker SL, Liu HH, Liao Z, Wei X, Wang S, Jin H, et al. Analysis of radiation pneumonitis risk using a generalized Lyman model [published correction appears in Int J Radiat Oncol Biol Phys. 2010 Sep 1;78(1):316-7. Dosage error in article text]. Int J Radiat Oncol Biol Phys. (2008) 72:568–74. doi: 10.1016/j.ijrobp.2008.04.053
64. Anthony GJ, Cunliffe A, Castillo R, Pham N, Guerrero T, Armato SG, et al. Incorporation of pre-therapy 18 F-FDG uptake data with CT texture features into a radiomics model for radiation pneumonitis diagnosis. Med Phys. (2017) 44:3686–94. doi: 10.1002/mp.12282
65. Krafft SP, Rao A, Stingo F, Briere TM, Court LE, Liao Z, et al. The utility of quantitative CT radiomics features for improved prediction of radiation pneumonitis. Med Phys. (2018) 45:5317–24. doi: 10.1002/mp.13150
66. Cunliffe A, Armato SG, Castillo R, Pham N, Guerrero T, Al-Hallaq HA. Lung texture in serial thoracic computed tomography scans: correlation of radiomics-based features with radiation therapy dose and radiation pneumonitis development. Int J Radiat Oncol Biol Phys. (2015) 91:1048–56. doi: 10.1016/j.ijrobp.2014.11.030
67. Liang B, Yan H, Tian Y, Chen X, Yan L, Zhang T, et al. Dosiomics: extracting 3D spatial features from dose distribution to predict incidence of radiation pneumonitis. Front Oncol. (2019) 9:269. doi: 10.3389/fonc.2019.00269
68. Liang B, Tian Y, Chen X, Yan H, Yan L, Zhang T, et al. Prediction of radiation pneumonitis with dose distribution: a convolutional neural network (CNN) based model. Front Oncol. (2020) 9:1500. doi: 10.3389/fonc.2019.01500
69. Moran A, Daly ME, Yip SF, Yamamoto T. Radiomics-based assessment of radiation-induced lung injury after stereotactic body radiotherapy. Clin Lung Cancer. (2017) 18:e425–31. doi: 10.1016/j.cllc.2017.05.014
70. Bousabarah K, Temming S, Hoevels M, Borggrefe J, Baus WW, Ruess D, et al. Radiomic analysis of planning computed tomograms for predicting radiation-induced lung injury and outcome in lung cancer patients treated with robotic stereotactic body radiation therapy. Strahlenther Onkol. (2019) 195:830–42. doi: 10.1007/s00066-019-01452-7
71. Starkov P, Aguilera TA, Golden DI, Shultz DB, Trakul N, Maxim PG, et al. The use of texture-based radiomics CT analysis to predict outcomes in early-stage non-small cell lung cancer treated with stereotactic ablative radiotherapy. Br J Radiol. (2019) 92:20180228. doi: 10.1259/bjr.20180228
72. van Timmeren JE, van Elmpt W, Leijenaar RTH, Reymen B, Monshouwer R, Bussink J, et al. Longitudinal radiomics of cone-beam CT images from non-small cell lung cancer patients: Evaluation of the added prognostic value for overall survival and locoregional recurrence. Radiother Oncol. (2019) 136:78–85. doi: 10.1016/j.radonc.2019.03.032
73. Vogelius IR, Bentzen SM. A literature-based meta-analysis of clinical risk factors for development of radiation induced pneumonitis. Acta Oncol. (2012) 51:975–83. doi: 10.3109/0284186X.2012.718093
74. De Ruysscher D, Jin J, Lautenschlaeger T, She JX, Liao Z, Kong FS. Blood-based biomarkers for precision medicine in lung cancer: precision radiation therapy. Transl Lung Cancer Res. (2017) 6:661–9. doi: 10.21037/tlcr.2017.09.12
75. Liu T, He Z, Dang J, Li G. Comparative efficacy and safety for different chemotherapy regimens used concurrently with thoracic radiation for locally advanced non-small cell lung cancer: a systematic review and network meta-analysis. Radiat Oncol. (2019) 14:55. doi: 10.1186/s13014-019-1239-7
76. Lee S, Ybarra N, Jeyaseelan K, Faria S, Kopek N, Brisebois P, et al. Bayesan network ensemble as a multivariate strategy to predict radiation pneumonitis risk. Med Phys. (2015) 42:2421–30. doi: 10.1118/1.4915284
77. Luna JM, Chao HH, Diffenderfer ES, Valdes G, Chinniah C, Ma G, et al. Predicting radiation pneumonitis in locally advanced stage II-III non-small cell lung cancer using machine learning. Radiother Oncol. (2019) 133:106–12. doi: 10.1016/j.radonc.2019.01.003
78. Clarke M, Collins R, Darby S, Davies C, Elphinstone P, Evans V, et al. Effects of radiotherapy and of differences in the extent of surgery for early breast cancer on local recurrence and 15-year survival: an overview of the randomised trials. Lancet. (2005) 366:2087–106. doi: 10.1016/S0140-6736(05)67887-7
79. Cserni G. Effects of radiotherapy and surgery for early breast cancer. Lancet. (2006) 367:1652–3. doi: 10.1016/S0140-6736(06)68724-2
80. EBCTCG (Early Breast Cancer Trialists’ Collaborative Group), McGale P, Taylor C, Correa C, Cutter D, Duane F, et al. Effect of radiotherapy after mastectomy and axillary surgery on 10-year recurrence and 20-year breast cancer mortality: meta-analysis of individual patient data for 8135 women in 22 randomised trials. Lancet. (2014) 383:2127–35. doi: 10.1016/S0140-6736(14)60488-8
81. Galper SL, Yu JB, Mauch PM, Strasser JF, Silver B, Lacasce A, et al. Clinically significant cardiac disease in patients with Hodgkin lymphoma treated with mediastinal irradiation. Blood. (2011) 117:412–8. doi: 10.1182/blood-2010-06-291328
82. Gagliardi G, Constine LS, Moiseenko V, Correa C, Pierce LJ, Allen AM, et al. Radiation dose-volume effects in the heart. Int J Radiat Oncol Biol Phys. (2010) 76(3 Suppl.):S77–85. doi: 10.1016/j.ijrobp.2009.04.093
83. Ghobadi G, van der Veen S, Bartelds B, de Boer RA, Dickinson MG, de Jong JR, et al. Physiological interaction of heart and lung in thoracic irradiation. Int J Radiat Oncol Biol Phys. (2012) 84:e639–46. doi: 10.1016/j.ijrobp.2012.07.2362
84. Cella L, Palma G, Deasy JO, Oh JH, Liuzzi R, D’Avino V, et al. Complication probability models for radiation-induced heart valvular dysfunction: do heart-lung interactions play a role? PLoS One. (2014) 9:e111753. doi: 10.1371/journal.pone.0111753
85. Correa CR, Das IJ, Litt HI, Ferrari V, Hwang WT, Solin LJ, et al. Association between tangential beam treatment parameters and cardiac abnormalities after definitive radiation treatment for left-sided breast cancer. Int J Radiat Oncol Biol Phys. (2008) 72:508–16. doi: 10.1016/j.ijrobp.2007.12.037
86. Bian SX, Korah MP, Whitaker TR, Ji L, Groshen S, Chung E. No acute changes in LVEF observed with concurrent trastuzumab and breast radiation with low heart doses. Clin Breast Cancer. (2017) 17:510–5. doi: 10.1016/j.clbc.2017.03.011
87. Cao L, Cai G, Chang C, Yang ZZ, Feng Y, Yu XL, et al. Early cardiac toxicity following adjuvant radiotherapy of left-sided breast cancer with or without concurrent trastuzumab. Oncotarget. (2016) 7:1042–54. doi: 10.18632/oncotarget.6053
88. Saiki H, Petersen IA, Scott CG, Bailey KR, Dunlay SM, Finley RR, et al. Risk of heart failure with preserved ejection fraction in older women after contemporary radiotherapy for breast cancer. Circulation. (2017) 135:1388–96. doi: 10.1161/CIRCULATIONAHA.116.025434
89. Darby SC, Ewertz M, McGale P, Bennet AM, Goldman-Blom U, Brønnum D, et al. Risk of ischemic heart disease in women after radiotherapy for breast cancer. N Engl J Med. (2013) 368:987–98. doi: 10.1056/NEJMoa1209825
90. van den Bogaard VA, Ta BD, van der Schaaf A, Bouma AB, Middag AMH, Bantema-Joppe EJ, et al. Validation and modification of a prediction model for acute cardiac events in patients with breast cancer treated with radiotherapy based on three-dimensional dose distributions to cardiac substructures. J Clin Oncol. (2017) 35:1171–8. doi: 10.1200/JCO.2016.69.8480
91. van den Bogaard VAB, van Luijk P, Hummel YM, van der Meer P, Schuit E, Boerman LM, et al. Cardiac function after radiation therapy for breast cancer. Int J Radiat Oncol Biol Phys. (2019) 104:392–400. doi: 10.1016/j.ijrobp.2019.02.003
92. Lorenzen EL, Taylor CW, Maraldo M, Nielsen MH, Offersen BV, Andersen MR, et al. Inter-observer variation in delineation of the heart and left anterior descending coronary artery in radiotherapy for breast cancer: a multi-centre study from Denmark and the UK. Radiother Oncol. (2013) 108:254–8. doi: 10.1016/j.radonc.2013.06.025
93. Feng M, Moran JM, Koelling T, Chugtai A, Chan JL, Freedman L, et al. Development and validation of a heart atlas to study cardiac exposure to radiation following treatment for breast cancer. Int J Radiat Oncol Biol Phys. (2011) 79:10–8. doi: 10.1016/j.ijrobp.2009.10.058
94. Lee J, Hua KL, Hsu SM, Lin JB, Lee CH, Lu KW, et al. Development of delineation for the left anterior descending coronary artery region in left breast cancer radiotherapy: an optimized organ at risk. Radiother Oncol. (2017) 122:423–30. doi: 10.106/j.radonc.2016.12.029
95. Francolini G, Desideri I, Meattini I, Becherini C, Terziani F, Olmetto E, et al. Assessment of a guideline-based heart substructures delineation in left-sided breast cancer patients undergoing adjuvant radiotherapy : Quality assessment within a randomized phase III trial testing a cardioprotective treatment strategy (SAFE-2014). Strahlenther Onkol. (2019) 195:43–51. doi: 10.1007/s00066-018-1388-x
96. Jacob S, Camilleri J, Derreumaux S, Walker V, Lairez O, Lapeyre M, et al. Is mean heart dose a relevant surrogate parameter of left ventricle and coronary arteries exposure during breast cancer radiotherapy: a dosimetric evaluation based on individually-determined radiation dose (BACCARAT study). Radiat Oncol. (2019) 14:29. doi: 10.1186/s13014-019-1234-z
97. Kolossváry M, Karády J, Kikuchi Y, Ivanov A, Schlett CL, Lu MT, et al. Radiomics versus visual and histogram-based assessment to identify atheromatous lesions at coronary CT angiography: an ex vivo study. Radiology. (2019) 293:89–96. doi: 10.1148/radiol.2019190407
98. Currie G, Iqbal B, Kiat H. Intelligent imaging: radiomics and artificial neural networks in heart failure. J Med Imaging Radiat Sci. (2019) 50:571–4. doi: 10.1016/j.jmir.2019.08.006
99. Lustberg T, van Soest J, Jochems A, Deist T, van Wijk Y, Walsh S, et al. Big data in radiation therapy: challenges and opportunities. Br J Radiol. (2017) 90:20160689. doi: 10.1259/bjr.20160689
100. Mottet N, Bellmunt J, Bolla M, Briers E, Cumberbatch MG, De Santis M, et al. EAU-ESTRO-SIOG guidelines on prostate cancer. part 1: screening, diagnosis, and local treatment with curative intent. Eur Urol. (2017) 71:618–29. doi: 10.1016/j.eururo.2016.08.003
101. Davis KM, Kelly SP, Luta G, Tomko C, Miller AB, Taylor KL. The association of long-term treatment-related side effects with cancer-specific and general quality of life among prostate cancer survivors. Urology. (2014) 84:300–6. doi: 10.1016/j.urology.2014.04.036
102. Viani GA, Stefano EJ, Afonso SL. Higher-than-conventional radiation doses in localized prostate cancer treatment: a meta-analysis of randomized, controlled trials. Int J Radiat Oncol Biol Phys. (2009) 74:1405–18. doi: 10.1016/j.ijrobp.2008.10.091
103. Fiorino C, Fellin G, Rancati T, Vavassori V, Bianchi C, Borca Casanova V, et al. Clinical and dosimetric predictors of late rectal syndrome after 3D-CRT for localized prostate cancer: preliminary results of a multicenter prospective study. Int J Radiat Oncol Biol Phys. (2008) 70:1130–7. doi: 10.1016/j.ijrobp.2007.07.2354
104. Michalski JM, Yan Y, Watkins-Bruner D, Bosch WR, Winter K, Galvin JM, et al. Preliminary toxicity analysis of 3-dimensional conformal radiation therapy versus intensity modulated radiation therapy on the high-dose arm of the Radiation Therapy Oncology Group 0126 prostate cancer trial. Int J Radiat Oncol Biol Phys. (2013) 87:932–8. doi: 10.1016/j.ijrobp.2013.07.041
105. Liu M, Moiseenko V, Agranovich A, Karvat A, Kwan W, Saleh ZH, et al. Normal tissue complication probability (NTCP) modeling of late rectal bleeding following external beam radiotherapy for prostate cancer: a test of the QUANTEC-recommended NTCP model. Acta Oncol. (2010) 49:1040–4. doi: 10.3109/0284186X.2010.509736
106. Troeller A, Yan D, Marina O, Schulze D, Alber M, Parodi K, et al. Comparison and limitations of DVH-based NTCP models derived from 3D-CRT and IMRT data for prediction of gastrointestinal toxicities in prostate cancer patients by using propensity score matched pair analysis. Int J Radiat Oncol Biol Phys. (2015) 91:435–43. doi: 10.1016/j.ijrobp.2014.09.046
107. Gulliford SL, Foo K, Morgan RC, Aird EG, Bidmead AM, Critchley HC, et al. Dose-volume constraints to reduce rectal side effects from prostate radiotherapy: evidence from MRC RT01 Trial ISRCTN 47772397. Int J Radiat Oncol Biol Phys. (2010) 76:747–54. doi: 10.1016/j.ijrobp.2009.02.025
108. Fellin G, Rancati T, Fiorino C, Vavassori V, Antognoni P, Baccolini M, et al. Long term rectal function after high-dose prostatecancer radiotherapy: results from a prospective cohort study. Radiother Oncol. (2014) 110:272–7. doi: 10.1016/j.radonc.2013.09.028
109. Morgan SC, Hoffman K, Loblaw DA, Buyyounouski MK, Patton C, Barocas D, et al. Hypofractionated radiation therapy for localized prostate cancer: an ASTRO, ASCO, and AUA evidence-based guideline. J Clin Oncol. (2018) 36:JCO1801097. doi: 10.1200/JCO.18.01097
110. Musunuru HB, Davidson M, Cheung P, Vesprini D, Liu S, Chung H, et al. Predictive parameters of symptomatic hematochezia following 5-fraction gantry-based SABR in prostate cancer. Int J Radiat Oncol Biol Phys. (2016) 94:1043–51. doi: 10.1016/j.ijrobp.2015.12.010
111. Alayed Y, Davidson M, Quon H, Cheung P, Chu W, Chung HT, et al. Dosimetric predictors of toxicity and quality of life following prostate stereotactic ablative radiotherapy. Radiother Oncol. (2019) 144:135–40. doi: 10.1016/j.radonc.2019.11.017
112. Abdollahi H, Mahdavi SR, Mofid B, Bakhshandeh M, Razzaghdoust A, Saadipoor A, et al. Rectal wall MRI radiomics in prostate cancer patients: prediction of and correlation with early rectal toxicity. Int J Radiat Biol. (2018) 94:829–37. doi: 10.1080/09553002.2018
113. Abdollahi H, Tanha K, Mofid B, Razzaghdoust A, Saadipoor A, Khalafi L, et al. MRI radiomic analysis of IMRT-induced bladder wall changes in prostate cancer patients: a relationship with radiation dose and toxicity. J Med Imaging Radiat Sci. (2019) 50:252–60. doi: 10.1016/j.jmir.2018.12.002
114. Abdollahi H, Mahdavi SR, Shiri I, Mofid B, Bakhshandeh M, Rahmani K. Magnetic resonance imaging radiomic feature analysis of radiation-induced femoral head changes in prostate cancer radiotherapy. J Cancer Res Ther. (2019) 15(Supplement):S11–9. doi: 10.4103/jcrt.JCRT_172_18
115. Rossi L, Bijman R, Schillemans W, Aluwini S, Cavedon C, Witte M, et al. Texture analysis of 3D dose distribution for predictive modelling of toxicity rates in radiotherapy. Radiother Oncol. (2018) 129:548–53. doi: 10.1016/j.radonc.2018.07.027
116. Mostafaei S, Abdollahi H, Kazempour Dehkordi S, Shiri I, Razzaghdoust A, Moghaddam SHZ, et al. CT imaging markers to improve radiation toxicity prediction in prostate cancer radiotherapy by stacking regression algorithm. Radiol Med. (2020) 125:87–97. doi: 10.1007/s11547-019-01082-0
117. Lorenz JW, Schott D, Rein L, Mostafaei F, Noid G, Lawton C, et al. Serial T2-weighted magnetic resonance images acquired on a 1.5 tesla magnetic resonance linear accelerator reveal radiomic feature variation in organs at risk: an exploratory analysis of novel metrics of tissue response in prostate cancer. Cureus. (2019) 11:e4510. doi: 10.7759/cureus.4510
118. Lee S, Kerns S, Ostrer H, Rosenstein B, Deasy JO, Oh JH. Machine learning on a genome-wide association study to predict late genitourinary toxicity after prostate radiation therapy. Int J Radiat Oncol Biol Phys. (2018) 101:128–35. doi: 10.1016/j.ijrobp.2018.01.054
119. Sanduleanu S, Woodruff HC, de Jong EEC, van Timmeren JE, Jochems A, Dubois L, et al. Tracking tumor biology with radiomics: a systematic review utilizing a radiomics quality score. Radiother Oncol. (2018) 127:349–60. doi: 10.1016/j.radonc.2018.03.033
120. Zwanenburg A, Vallieres M, Abdalah MA, Aerts HJWL, Andrearczyk V, Apte A, et al. The image biomarker standardization initiative: standardized quantitative radiomics for high-throughput image-based phenotyping. Radiology. (2020) 295:328–38. doi: 10.1148/radiol.2020191145
121. Traverso A, Wee L, Dekker A, Gillies R. Repeatability and reproducibility of radiomic features: a systematic review. Int J Radiat Oncol Biol Phys. (2018) 102:1143–58. doi: 10.1016/j.ijrobp.2018.05.053
122. Welch ML, McIntosh C, Haibe-Kains B, Milosevic MF, Wee L, Dekker A, et al. Vulnerabilities of radiomic signature development: the need of safeguards. Radiother Oncol. (2019) 130:2–9. doi: 10.1016/j.radonc.2018.10.027
123. Sharabiani M, Clementel E, Andratschke N, Hurkmans C. Generalizability assessment of head and neck cancer NTCP models based on the TRIPOD criteria. Radiother Oncol. (2020) 146:143–50. doi: 10.1016/j.radonc.2020.02.013
Keywords: Radiomics, Intensity modulated radiotherapy, xerostomia, radiation induced lung injury, cardiac toxicity, lower gastro-intestinal toxicity
Citation: Desideri I, Loi M, Francolini G, Becherini C, Livi L and Bonomo P (2020) Application of Radiomics for the Prediction of Radiation-Induced Toxicity in the IMRT Era: Current State-of-the-Art. Front. Oncol. 10:1708. doi: 10.3389/fonc.2020.01708
Received: 13 February 2020; Accepted: 30 July 2020;
Published: 06 October 2020.
Edited by:
Tiziana Rancati, Istituto Nazionale dei Tumori (IRCCS), ItalyReviewed by:
Arjen Van Der Schaaf, University Medical Center Groningen, NetherlandsElisa Scalco, Italian National Research Council, Italy
Copyright © 2020 Desideri, Loi, Francolini, Becherini, Livi and Bonomo. This is an open-access article distributed under the terms of the Creative Commons Attribution License (CC BY). The use, distribution or reproduction in other forums is permitted, provided the original author(s) and the copyright owner(s) are credited and that the original publication in this journal is cited, in accordance with accepted academic practice. No use, distribution or reproduction is permitted which does not comply with these terms.
*Correspondence: Pierluigi Bonomo, bonomop@aou-careggi.toscana.it; orcid.org/0000-0001-8999-5208