- 1Department of Animal Science, College of Agriculture and Natural Resources, University of Tehran, Karaj, Iran
- 2Biomedical Center for Systems Biology Science Munich, Ludwig-Maximilians-University, Munich, Germany
- 3Department of Animal and Poultry Science, College of Aburaihan, University of Tehran, Tehran, Iran
- 4Department of Physical Education and Sports Science, School of Psychology and Educational Sciences, Yazd University, Yazd, Iran
- 5Biotechnology Research Center, Karaj Branch, Islamic Azad University, Karaj, Iran
- 6Department of Medical Mycology, School of Medical Science, Tarbiat Modares University, Tehran, Iran
- 7Department of Production Animal Health, Faculty of Veterinary Medicine, University of Calgary, Calgary, AB, Canada
Background: The recent emergence of COVID-19, rapid worldwide spread, and incomplete knowledge of molecular mechanisms underlying SARS-CoV-2 infection have limited development of therapeutic strategies. Our objective was to systematically investigate molecular regulatory mechanisms of COVID-19, using a combination of high throughput RNA-sequencing-based transcriptomics and systems biology approaches.
Methods: RNA-Seq data from peripheral blood mononuclear cells (PBMCs) of healthy persons, mild and severe 17 COVID-19 patients were analyzed to generate a gene expression matrix. Weighted gene co-expression network analysis (WGCNA) was used to identify co-expression modules in healthy samples as a reference set. For differential co-expression network analysis, module preservation and module-trait relationships approaches were used to identify key modules. Then, protein-protein interaction (PPI) networks, based on co-expressed hub genes, were constructed to identify hub genes/TFs with the highest information transfer (hub-high traffic genes) within candidate modules.
Results: Based on differential co-expression network analysis, connectivity patterns and network density, 72% (15 of 21) of modules identified in healthy samples were altered by SARS-CoV-2 infection. Therefore, SARS-CoV-2 caused systemic perturbations in host biological gene networks. In functional enrichment analysis, among 15 non-preserved modules and two significant highly-correlated modules (identified by MTRs), 9 modules were directly related to the host immune response and COVID-19 immunopathogenesis. Intriguingly, systemic investigation of SARS-CoV-2 infection identified signaling pathways and key genes/proteins associated with COVID-19’s main hallmarks, e.g., cytokine storm, respiratory distress syndrome (ARDS), acute lung injury (ALI), lymphopenia, coagulation disorders, thrombosis, and pregnancy complications, as well as comorbidities associated with COVID-19, e.g., asthma, diabetic complications, cardiovascular diseases (CVDs), liver disorders and acute kidney injury (AKI). Topological analysis with betweenness centrality (BC) identified 290 hub-high traffic genes, central in both co-expression and PPI networks. We also identified several transcriptional regulatory factors, including NFKB1, HIF1A, AHR, and TP53, with important immunoregulatory roles in SARS-CoV-2 infection. Moreover, several hub-high traffic genes, including IL6, IL1B, IL10, TNF, SOCS1, SOCS3, ICAM1, PTEN, RHOA, GDI2, SUMO1, CASP1, IRAK3, HSPA5, ADRB2, PRF1, GZMB, OASL, CCL5, HSP90AA1, HSPD1, IFNG, MAPK1, RAB5A, and TNFRSF1A had the highest rates of information transfer in 9 candidate modules and central roles in COVID-19 immunopathogenesis.
Conclusion: This study provides comprehensive information on molecular mechanisms of SARS-CoV-2-host interactions and identifies several hub-high traffic genes as promising therapeutic targets for the COVID-19 pandemic.
Introduction
Coronavirus disease 2019 (COVID-19) is an infectious disease that was first reported in Wuhan, China in December 2019 (1). This viral pneumonia spread rapidly, with the World Health Organization (WHO) declaring a global pandemic on March 11, 2020 (2). COVID-19, caused by a single-stranded RNA beta coronavirus called severe acute respiratory syndrome coronavirus 2 (SARS-CoV-2), affects the lower respiratory tract in humans (3). As of 1 October 2021, SARS-CoV-2 has infected 233,503,524 people and caused 4,777,503 deaths worldwide (https://covid19.who.int/table). Primary symptoms of SARS-CoV-2 infection include fever, cough, shortness of breath, and pneumonia (4, 5). The recent emergence of COVID-19 and inadequate knowledge of infection progress, molecular mechanisms involved in the disease, interactions between SARS-CoV-2 and the host, and their relationship to disease outcomes limit our ability to develop effective treatments for infected patients. Therefore, understanding molecular/immunological mechanisms underlying the various clinical symptoms of COVID-19 are deemed critical in development of potential therapeutic strategies (6, 7).
Detecting changes in gene expression in relevant tissues during SARS-CoV-2 infection through various functional genomic methods (e.g., microarrays and RNA-sequencing-based transcriptomics) can increase understanding of molecular mechanisms involved in COVID-19, plus host-pathogen interactions (8–10). Transcriptomic studies in COVID-19 patients used lung epithelial cells, nasopharyngeal swabs, bronchoalveolar lavage fluid (9, 11–14), or peripheral blood mononuclear cells (PBMCs) (6, 8, 15). However, differential gene expression analysis focuses more on individual effects of genes (16), whereas genes interact in complex biological gene networks (17). Therefore, investigation of gene or protein interactions at the systems level should elucidate dynamics of SARS-CoV-2 infection and molecular mechanisms responsible for COVID-19.
Recent computational methods of systems biology, including network analysis and machine learning, are suitable for analyzing omics techniques such as high throughput RNA-sequencing (RNA-seq) at the systemic level (18). Weighted gene co-expression network analysis (WGCNA) is a systems biology method to identify clusters (modules) of highly correlated genes, candidate biomarkers, and therapeutic targets (19); it has been used in various human infectious diseases, e.g., Influenza (20), Tuberculosis (21, 22), Hepatitis B,C (23–25), HIV (26), and various cancers (27–30). Additionally, in some studies using the module-trait relationships approach of WGCNA, modules related to clinical traits of COVID-19 were identified at various stages (31–33). WGCNA has a unique network-based approach called module preservation analysis (34), based on topological changes across conditions, e.g., comparison of samples from healthy versus diseased persons. Simply stated, differences in connectivity patterns and network density between healthy and disease samples as reference and test sets, respectively, indicate a disease-induced perturbation of the network (34). Therefore, non-preserved modules between healthy and disease samples are important candidates for biological investigation of a disease such as COVID-19. The module preservation approach of WGCNA is valuable for differential network analysis (17, 34) and has been successfully used for several human (35–37) and animal (16, 38) diseases.
Combining high-throughput technologies with computational systems biology methods such as WGCNA provides new opportunities to better understand molecular mechanisms responsible for diseases such as COVID-19 (39). To the best of our knowledge, this is the first differential co-expression network analysis on COVID-19, with the following purposes (1): Combining RNA-seq data and WGCNA to identify co-expression modules in healthy samples as the reference set (2); Module preservation analysis to detect non-preserved modules between healthy and COVID-19 (as test set) samples (3); Module-trait relationships analysis to identify significant highly-correlated modules with disease severity (4); Functional enrichment analysis of non-preserved modules for biological assessment and understanding molecular regulatory mechanisms behind COVID-19 (5); Identification of hub genes and potential transcription factors (TFs) in non-preserved modules; and (6) Extraction of protein-protein interaction (PPI) networks, based on hub genes of candidate modules for topological analysis, using betweenness centrality (BC) to identify hub genes with the highest BC score (hub-high traffic genes) as vital bridges for information transfer inside modules.
Materials and Methods
Gene Expression Datasets
RNA-sequencing (RNA-seq) raw reads of COVID-19 patients and healthy persons were obtained from the Gene Expression Omnibus (GEO) database at the National Center for Biotechnology Information (NCBI; accession number GSE152418) and the European Genome-phenome Archive (EGA; accession number EGAS00001004571). These datasets included 87 samples of PBMCs from healthy (n=17), mild (n=33) and severe (n=37) groups. An Illumina NovaSeq 6000 platform was used to generate 101-bp single-end reads, as detailed in the original reports (6, 7).
RNA-Seq Data Analysis
FastQCsoftware version 0.11.9 (https://www.bioinformatics.babraham.ac.uk/projects/fastqc/) was used for quality control of raw reads. Next, adapter sequences, low-quality reads, and bases were trimmed by Trimmomatic software (Version 0.39) (40) using the following criteria: ILLUMINACLIP: Adapter.fa:2:30:10, LEADING:20, SLIDINGWINDOW:6:20, TRAILING:20, and MINLEN:50. After obtaining clean reads, their quality was verified with FastQC software to confirm improvements. Then, clean reads were aligned to the latest human reference genome (GRCh38) using Hisat2 software version 2.2.1 with default parameters (41). Python script HTSeq-count (42) version 0.13.5 was used to count uniquely mapped reads to annotated genes, based on the ENSEMBL human GTF file (release 104). Finally, all count files were merged into a table and a raw expression matrix created and normalized to log-counts per million (log-CPM) using the voom function of the limma R package (Version 3.46.0) (43, 44). To prevent sampling noise caused by low-expressed or low-variance genes, only genes with expression ≥ 1 count per million reads (CPM) in at least 5 samples and standard deviation > 0.25 were selected for downstream analyses.
Weighted Gene Co-Expression Network Analysis (WGCNA)
Based on the assumption that non-preserved modules between healthy controls and COVID-19 patients may explain biological behavior of COVID-19 and increase understanding of molecular mechanisms responsible for SARS-CoV-2 infection, healthy samples were selected as a reference set for construction of a weighted gene co-expression network and modules detection. In this study, a signed weighted gene co-expression network was constructed according to the standard procedure of WGCNA R package Version 1.70 (19). In general, signed networks provide a better understanding of molecular regulatory mechanisms at the systemic level, facilitating better separation of modules in terms of biological performance (19, 45). Initially, as WGCNA is very sensitive to outliers, the adjacency function of the WGCNA R package was used to construct distance-based adjacency metrics of samples; those with a standardized connectivity score < −2.5 were considered an outlier and removed. To ensure a scale-free network, pickSoftThreshold function of the WGCNA R package was used to identify the soft thresholding power β value. Moreover, since a Pearson correlation is susceptible to outliers, bi-weight mid-correlation were used to calculate pairwise correlations between genes, as it is more robust to outliers (46, 47). Therefore, a weighted adjacency matrix was constructed using the bi-weight mid-correlation coefficient at β = 12 as a soft thresholding power and subsequently transformed into a topological overlap matrix (TOM). Next, an average linkage hierarchical clustering analysis was performed based on the TOM dissimilarity (1-TOM) and modules were detected through a dynamic hybrid tree cutting algorithm. Finally, modules with very similar expression profiles or highly correlated eigengenes were merged. All of the above steps were performed by the 1-step network construction and module detection blockwiseModules function of the WGCNA R package with the following options: power = 12, networkType = “signed”, TOMType = “signed”, maxBlockSize = 15000, corType = “bicor”, reassignThreshold = 0, mergeCutHeight = 0.25, and minModuleSize = 30.
Module Preservation Analysis
To investigate whether the topological structure of modules changed between healthy (reference set) and COVID-19 (test set) samples, the modulePreservation function of the WGCNA R package was used for preservation analysis. In this step, 2 composite preservation statistics, Zsummary and medianRank, were investigated based on 200 random permutations. Modules with a higher value of Zsummary represent high preservation across conditions. However, Zsummary is highly dependent on module size, and as it increases, Zsummary also increases, whereas medianRank is independent of module size and unlike Zsummary, modules with low medianRank values indicate strong preservation across various conditions (34). Modules with values Zsummary > 10 and medianRank < 8 indicates high preservation between groups (34). Therefore, in this study, modules with values of Zsummary ≤ 10 or medianRank ≥ 8 were considered non-preserved modules.
Module-Trait Relationships Analysis
To identify significant highly-correlated modules with disease severity of COVID-19, 25 mild and 29 severe COVID-19 patients were used for module-trait relationships (MTRs) analysis. Because the gene co-expression analysis is very sensitive to outliers, the distance-based adjacency metrics of samples was calculated and samples with a standardized connectivity < −2.5 were removed, considered as an outlier. In addition, samples and genes with > 50% missing entries and genes with zero variance were identified and excluded from WGCNA analysis (35). Briefly, a correlation matrix of expression values was constructed using pairwise bi-weight mid-correlation coefficients between all pairs of genes across the selected samples. In other words, the genes in a module share strong interconnectedness (36). Finally, average linkage hierarchical clustering analysis was performed by the topological overlap-based dissimilarity matrix (1-TOM) as input, and modules were identified by dynamic hybrid tree cutting algorithm. Then, the modules with the highly correlated eigengenes were merged. The above steps were performed using automatic, one-step network construction and module detection function “blockwiseModules” of the WGCNA R package. Next, in order to identify the COVID-19-related modules, the correlation between the disease severity of COVID-19 and module eigengenes was taken using Pearson correlation coefficient.
Functional Enrichment Analysis of the Non-Preserved Modules
Enrichr online web tool (48) was used to analyze Gene Ontology (GO) and Kyoto Encyclopedia of Genes and Genomes (KEGG) pathways for non-preserved modules. Thus, all genes in each non-preserved module were investigated for functional characterization; only terms with a threshold of adj p value < 0.05 were deemed significant (p values corrected by the Benjamini-Hochberg method).
Hub Genes and TFs Detection in Non-Preserved and Correlated Modules
In complex biological networks, intramodular genes with the highest degree of connections (hub genes) have more biological relevance in association with a disease (49–52). Module memberships (MM) or eigengene-based connectivity kME, a criterion indicating the relationship of a gene with the relevant module, is calculated as the correlation between gene expression profile and module eigengenes (the first principal component of the expression profile for a module) by the WGCNA R package. Genes with higher MM values have a more significant relationship with biological performance of the respective module and act as central genes in that module (19, 53, 54). Therefore, genes with kME ≥ 0.7 were selected as intramodular hub genes in modules. Next, for construction of protein-protein interaction (PPI) networks, identified hub genes in each module were subjected to the search tool for retrieval of interacting genes (STRING) database (55). Additionally, transcriptional regulatory factors within modules were identified using a set of human transcription factors extracted from the HumanTFDB database (56).
Identification of Hub-High Traffic Genes and Network Visualization
PPI networks derived from hub genes of non-preserved modules from the previous step were used for topological analyses with the betweenness centrality (BC) measure. In connected networks, BC is a general measure of centrality, based on the number of shortest paths between every 2 other genes that pass through a certain gene, indicating influence over information transfer between genes in modules (57–61). Genes with the highest BC scores, termed high traffic genes, have a central role in association with the biological behavior of the respective module (36, 62). In this study, we used 2 methods to calculate BC scores in the hub gene-base PPI networks from modules. The first method was to calculate BC scores through cytoHubba (a cytoscape plugin) Version 0.1, using the Betweenness algorithm (63), whereas the second method was to calculate BC scores through Igraph (Version 1.2.6) R package (https://cran.r-project.org/web/packages/igraph/). Finally, overlapped genes with the highest BC score between these 2 methods were selected and the top 50 hub genes in modules with a size of ≥ 450 and the top 10 hub genes in modules with a size of ≤ 180 in terms of BC score were considered hub high-traffic genes. Additionally, COVID-19 biologically related modules were visualized using Cytoscape Version 3.8.2 (64).
Results
RNA-Seq Data Analysis
The stringent step-by-step workflow of the differential co-expression network analysis used in this study is shown (Figure 1). Briefly, RNA-seq data contained 87 samples (17 healthy and 70 COVID-19 patients) of PBMCs, with an average of 23 million reads per sample. Details regarding RNA-seq data analysis and preprocessing are in Supplementary Table 1. After normalization and filtering of low expressed and low variance genes, 11663 genes remained for weighted gene co-expression network construction. The normalized and filtered gene expression matrix of healthy controls and COVID-19 patients is in Supplementary Table 2.
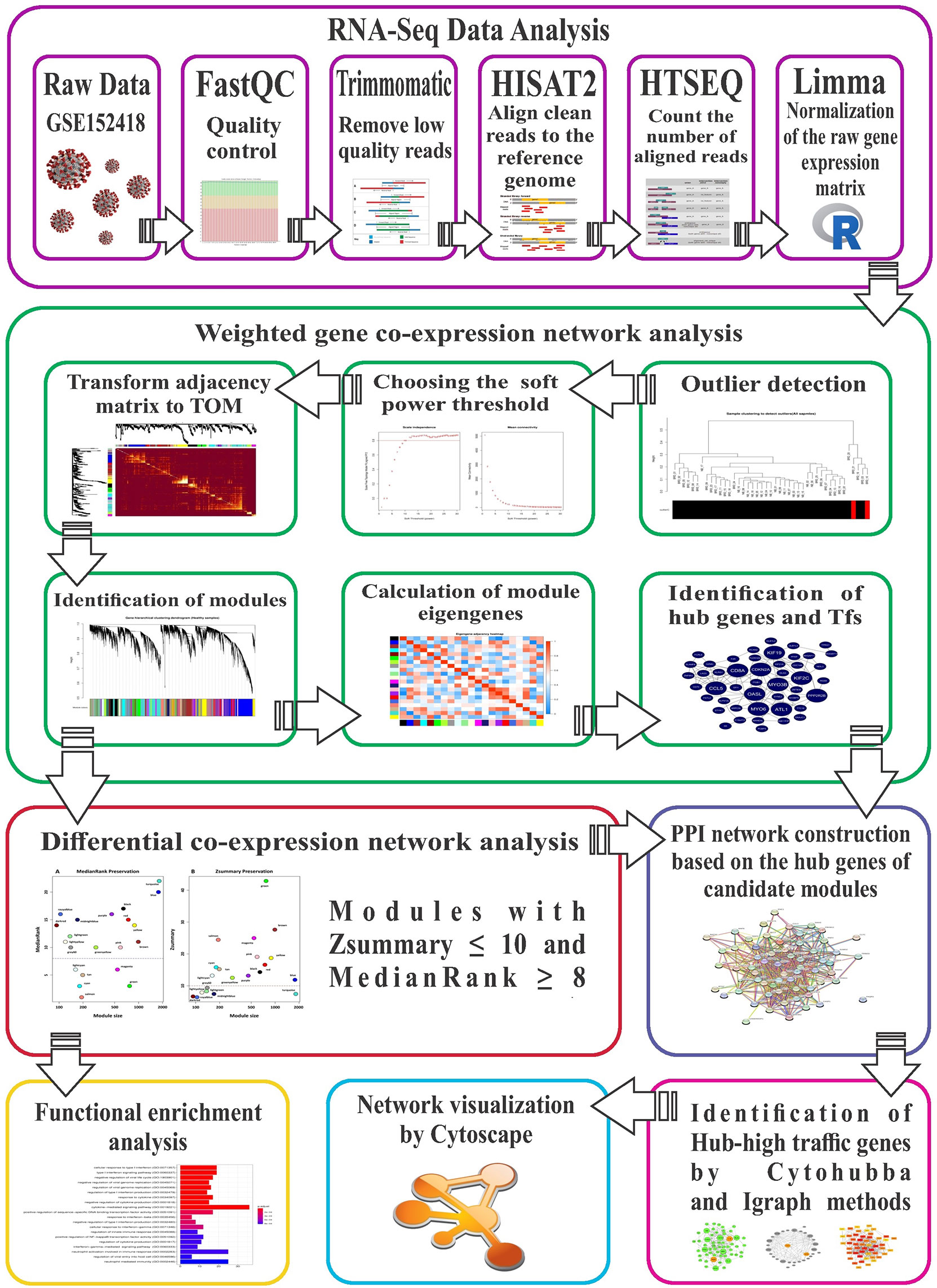
Figure 1 The stringent step-by-step pipeline of the RNA-seq data analysis and differential co-expression network approach in this study.
Weighted Gene Co-Expression Network Construction
Before co-expression network construction and module detection, distance-based adjacency metrics of samples were constructed to identify outliers and prevent their negative influence on network analysis. All samples had a standardized connectivity score > −2.5, with no outliers (Figure 2A). Weighted gene co-expression network was constructed at β = 12, representing a scale free topology fitting index (R2) ≥ 0.80. In total, 21 co-expression modules (average of 525 genes) and 647 background genes (grey module) were identified in healthy samples (reference set) through hierarchical clustering analysis and dynamic hybrid tree cutting algorithm. Moreover, each module as a branch of the hierarchical clustering dendrogram was labeled with a specific color by the WGCNA R package (Figure 2B). The turquoise module with a size of 1818 and the darkred module with 93 genes were the largest and smallest modules, respectively. Complete module information is provided in Supplementary Table 3.
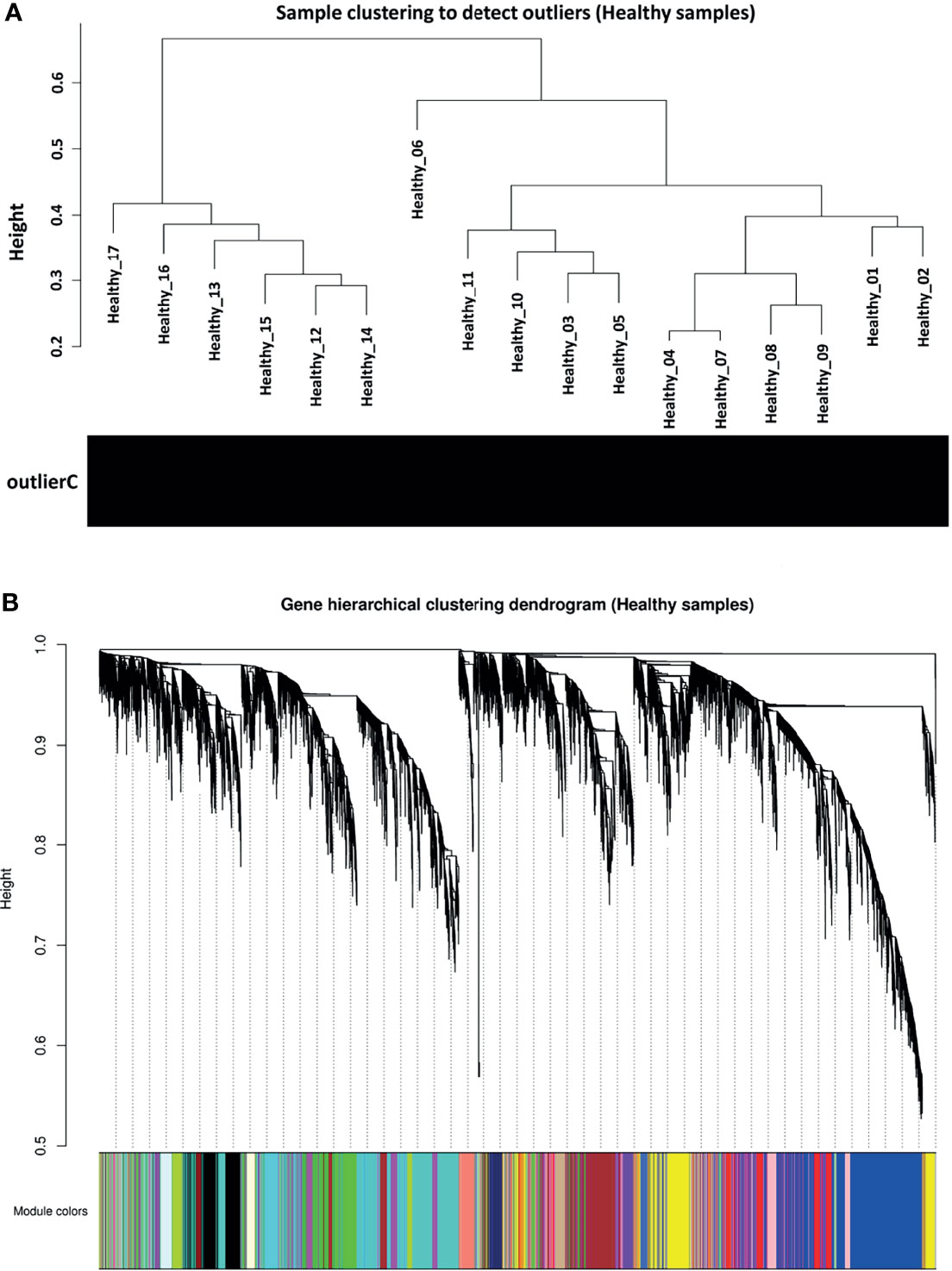
Figure 2 Preprocessing of weighted gene co-expression network analysis. (A) Sample clustering to detect outliers in healthy samples as reference set. All samples had a standardized connectivity score > −2.5. (B) Gene hierarchical clustering dendrogram of 21 detected modules based on a dissimilarity (1-TOM) measure. Branches represent modules that are marked with a specific color. The y-axis represents the co-expression distance, the x-axis represents genes and the grey module represents background genes.
Module Preservation Analysis
Based on module preservation analysis, among identified modules, the topological structure of 6 modules including salmon (medianRank = 1; Zsummary = 24), cyan (medianRank = 3; Zsummary = 16), green (medianRank = 3; Zsummary = 43), tan (medianRank = 5; Zsummary = 15), lightcyan (medianRank = 6; Zsummary = 13), and magenta (medianRank = 6; Zsummary = 25) was highly-preserved between healthy and COVID-19 samples (Figure 3). Moreover, according to our assumption, connectivity patterns and network densities of the other 15 modules were changed under COVID-19 conditions (Figure 3). Among the 15 non-preserved modules, the turquoise module (medianRank = 22; Zsummary = 7.4) had the highest degree of change in the topological structure affected by SARS-CoV-2 infection. More information regarding preservation status of all modules is available in Supplementary Table 4.
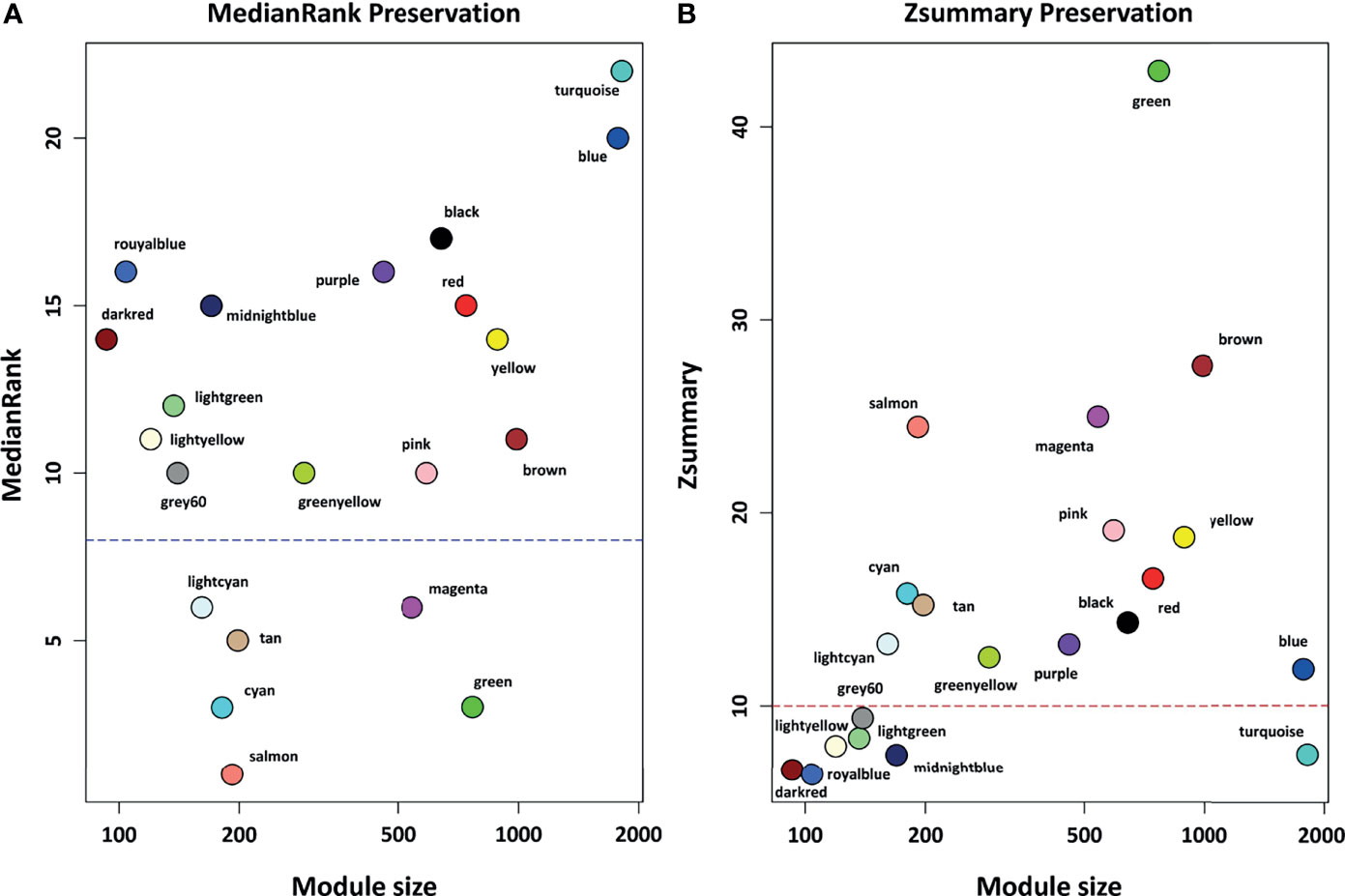
Figure 3 The preservation status of the respective modules. (A) MedianRank preservation results. The y axis represents medianRank values and x axis represent module size. Each point with a specific color represents the corresponding module. (B) Zsummary preservation results. The y axis represents Zsummary values and x axis represents the module size. Each point with a specific color represents the corresponding module. Modules with medianRank ≥ 8 (the blue dashed line) or Zsummary ≤ 10 (the red dashed line) were considered non-preserved between healthy controls and COVID-19 samples.
Functional Enrichment Analysis of the Non-Preserved Modules
To identify biological processes associated with non-preserved modules, GO analysis was performed and a total of 320 biological processes were significantly enriched in the 13 non-preserved modules including blue, brown, grey60, lightgreen, midnightblue, pink, purple, turquoise, black, darkred, greenyellow, red, and yellow. In the other 2 non-preserved modules, including lightyellow and royalblue, no biological process was significantly enriched. Conversely, KEGG pathways analysis identified 97 significant terms in 10 non-preserved modules (blue, brown, grey60, lightgreen, lightyellow, pink, purple, greenyellow, red, and yellow) as well as no enriched term in the other 5 non-preserved modules (midnightblue, turquoise, black, darkred, and royalblue). Additionally, among the non-preserved modules, the blue module had the most significant enriched terms with 148 and 47 biological processes and KEGG pathways, respectively. Complete information regarding functional enrichment analysis is provided in Supplementary Table 5. Based on functional enrichment analysis, 9 non-preserved modules including blue, brown, grey60, lightgreen, lightyellow, midnightblue, pink, purple, and turquoise were related to the host immune response and SARS-CoV-2 infection. Among these 9 candidate non-preserved modules, the blue module had the most biological associations with the immunopathogenesis of COVID-19. The top biological processes of non-preserved modules are shown in Figure 4.
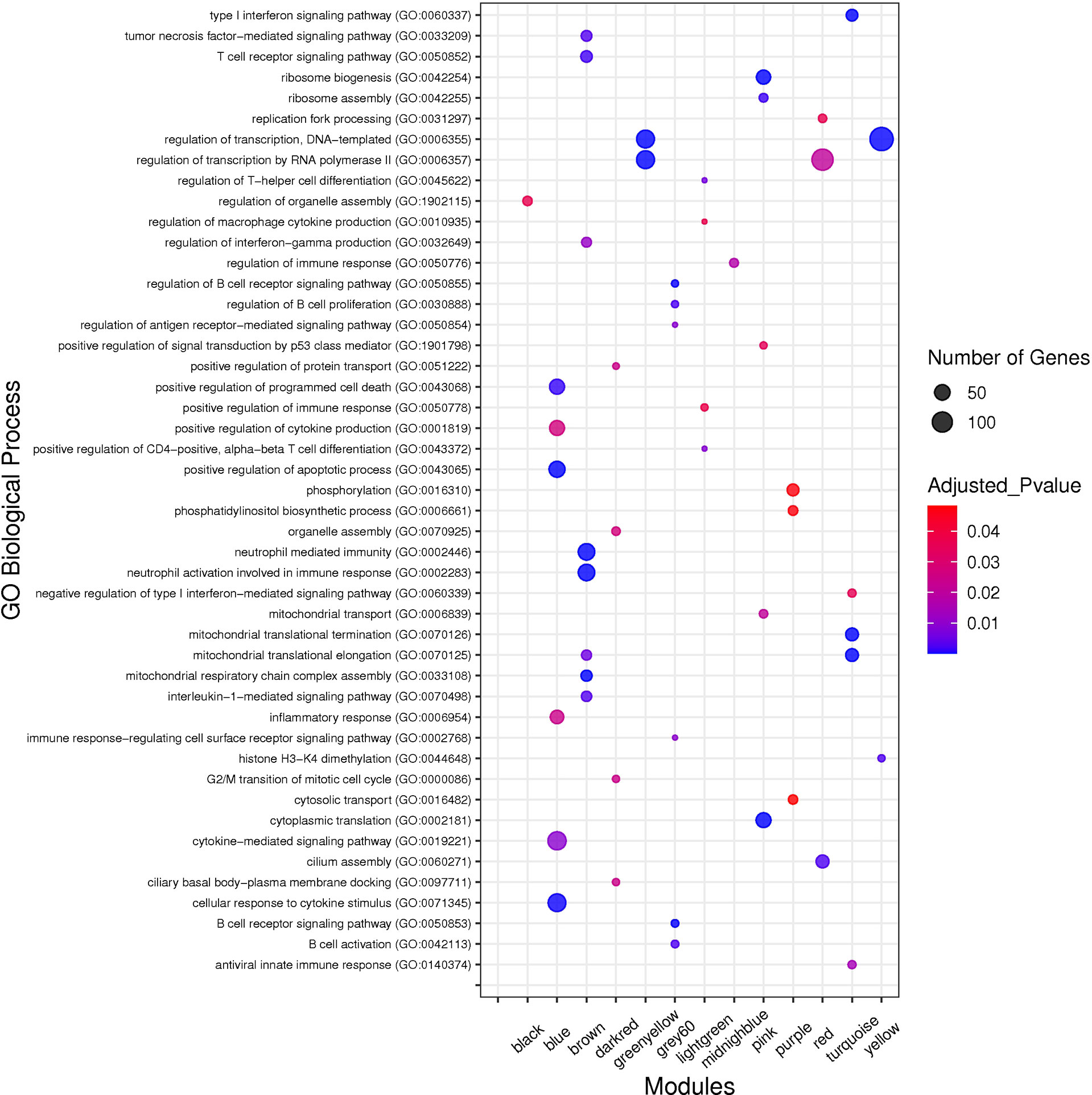
Figure 4 The top GO biological processes of the non-preserved modules. The y axis and the x axis represent significant enriched GO terms and module name, respectively. Color and size of each point represent adjusted p value and number of genes for each term, respectively.
TFs, Hub TFs, Hub Genes, and Hub-High Traffic Genes Identification in Non-Preserved Modules
Here, the differential co-expression network approach and functional enrichment analysis revealed 9 candidate non-preserved modules with a: 1) change in topological structure due to COVID-19; and 2) biological relation to SARS-CoV-2 infection. Using the MM criterion to assess these modules, a total of 881, 369, 85, 62, 59, 64, 251, 253, and 555 intramodular hub genes were identified in the blue, brown, grey60, lightgreen, lightyellow, midnightblue, pink, purple, and turquoise modules, respectively. The complete list of the hub genes of the non-preserved modules is provided in Supplementary Table 6. In addition, based on co-expressed hub genes, the PPI network of the blue module is shown in Figure 5. Additionally, based on human transcriptional regulatory factors extracted from the HumanTFDB database, a total of 183, 33, 12, 14, 5, 10, 34, 18, and 131 TFs were detected in the blue, brown, grey60, lightgreen, lightyellow, midnightblue, pink, purple, and turquoise modules, respectively. Complete information of TFs detected in non-preserved modules are in Supplementary Table 7. Furthermore, among detected hub genes, we identified 110, 5, 8, 8, 2, 5, 16, 11, and 49 TFs (hub TFs) in the blue, brown, grey60, lightgreen, lightyellow, midnightblue, pink, purple, and turquoise modules, respectively (Supplementary Table 8). Moreover, the identified hub-high traffic genes in the 9 candidate non-preserved modules along with their BC scores obtained by both cytoHubba and Igraph methods are presented in Table 1 and Supplementary Table 9. These genes were highly connected intramodular hubs in the 9 candidate co-expression modules and they were also central genes for information transfer within the hub-gene based PPI networks of their respective modules. Therefore, hub-high traffic genes are considered important candidates for prognostic and therapeutic targets for COVID-19. Additionally, PPI networks of the 9 candidate non-preserved modules are in Supplementary Figure 1.
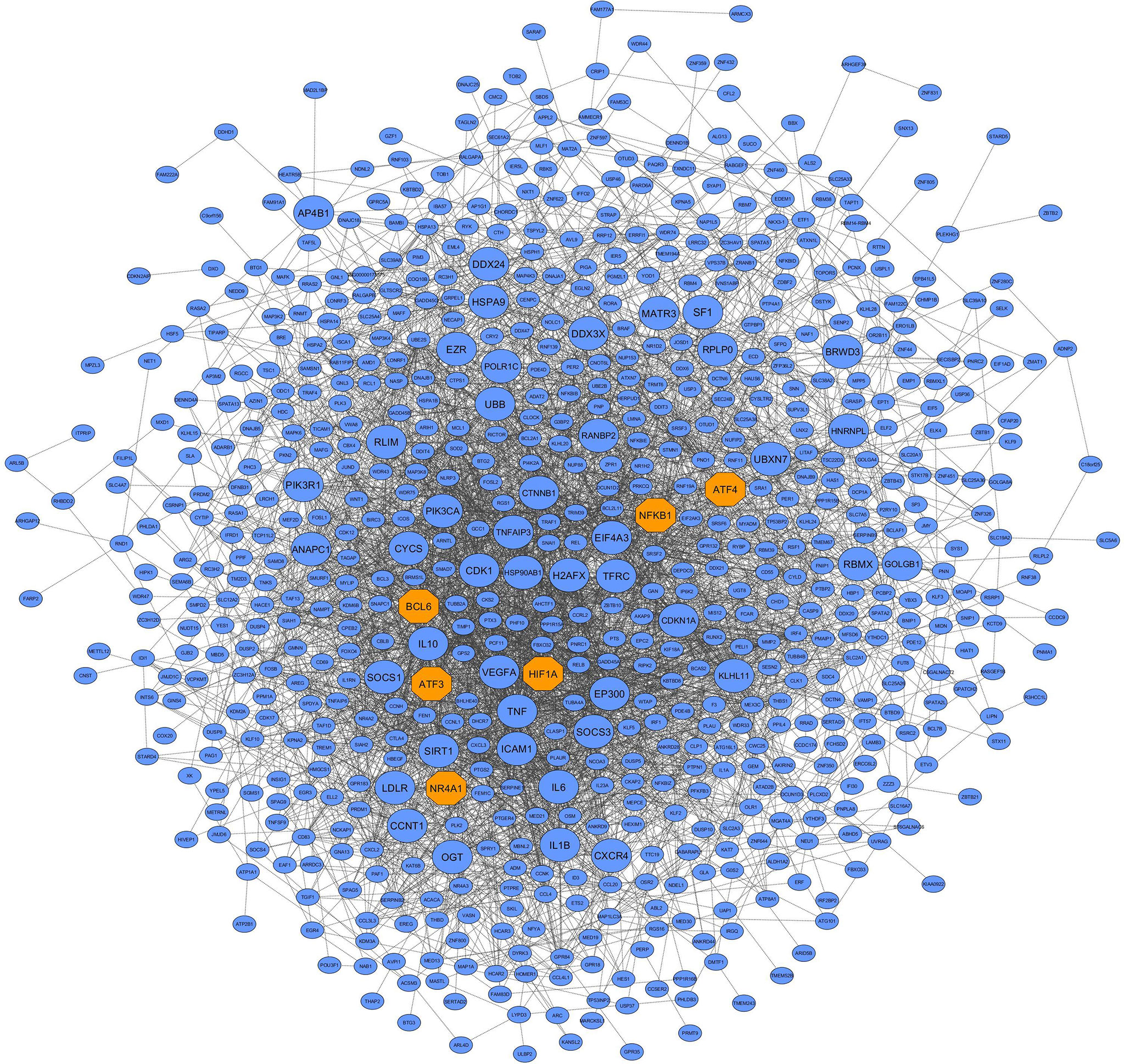
Figure 5 PPI network based on the co-expressed hub genes of the blue module. This module had the most biological associations with the immunopathogenesis of COVID-19. Large circles and orange octagons represent hub-high traffic genes and TFs, respectively.
MTRs Analysis
The weighted adjacency matrix was constructed at β = 10 whose scale-free topology fitting index (R2) was ≥ 0.80. After network construction, 12 co-expression modules (excluding grey module with 690 uncorrelated genes) were identified through hierarchical clustering and dynamic hybrid tree cutting with an average size of 784 genes. Disease severity related to COVID-19 that were used in MTRs included clinical signs measurements of COVID-19 such as flu-like illness (FLI), mild COVID-19, severe COVID-19. Among the significant modules: purple (R = −0.63, P = 1e−05), blue (R = −0.71, P = 2e−07), brown (R = −0.55, P = 2e−04), and turquoise (R = 0.7, P = 3e−07) modules were significant highly-correlated and yellow (R = −0.41, P = 0.008), tan (R = −0.32, P = 0.04), greenyellow (R = 0.37, P = 0.02), and pink (R = −0.38, P = 0.01) modules were significant moderately-correlated with severe COVID-19, respectively (Figure 6A). Also, purple (R = −0.64, P = 8e−06), blue (R = −0.75, P = 1e−08), brown (R = −0.55, P = 2e−04), and turquoise (R = 0.72, P = 1e−07) modules were significant highly-correlated and yellow (R = −0.42, P = 0.006), tan (R = −0.31, P = 0.04), black (R = 0.33, P = 0.04), and pink (R = −0.34, P = 0.03) modules were significant moderately-correlated with mild COVID-19, respectively (Figure 6B). Then, the significant highly-correlated modules were selected for downstream analysis. Briefly, the turquoise, blue, brown, and purple modules with module sizes of 2592, 1691, 1214 and 141 genes, respectively, were identified as significant highly-correlated modules with disease severity (Figure 6A). All of the detected modules were also identified with MP method. It is noteworthy that both WGCNA methods showed a similar ability to identify candidate modules during COVID-19, confirming each other results.
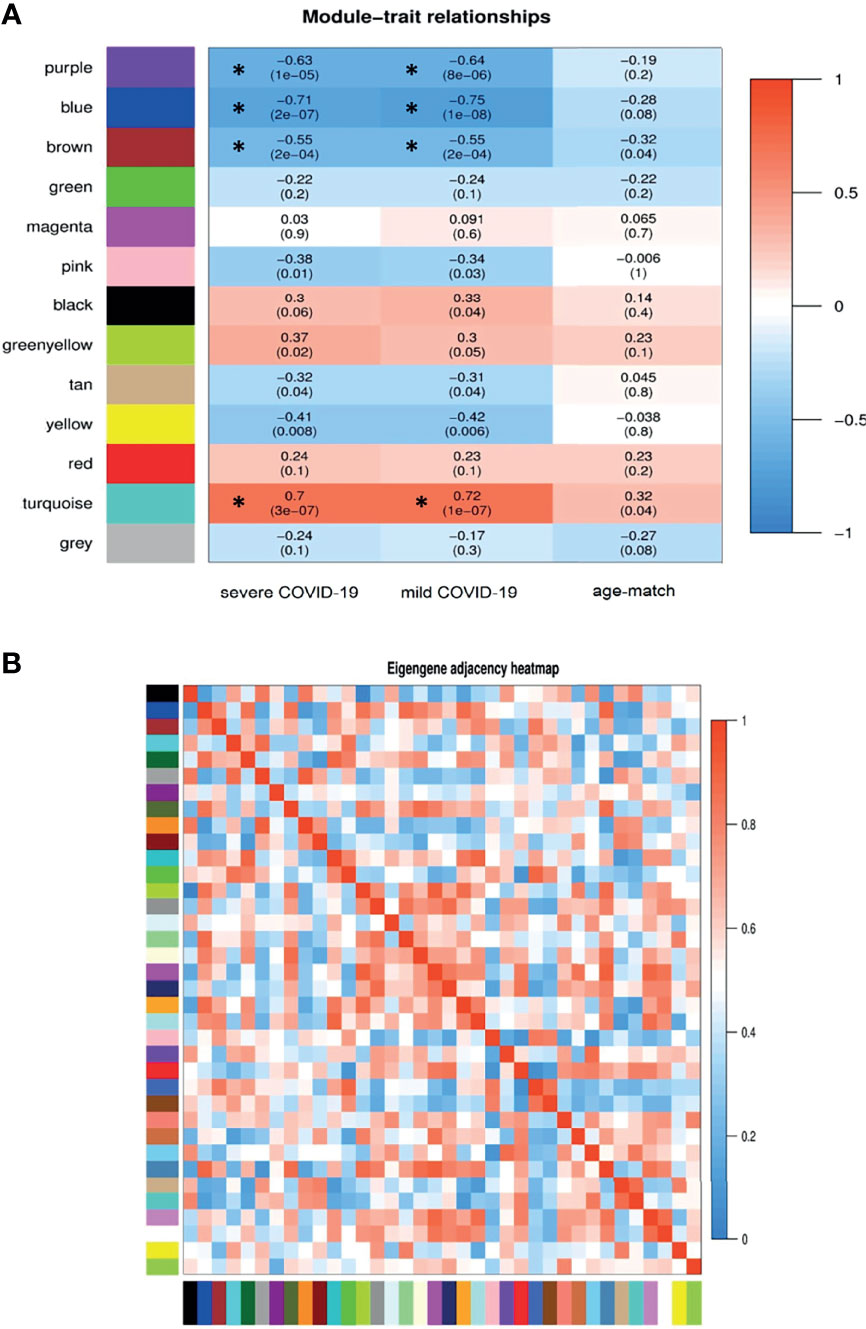
Figure 6 Module-trait relationships analysis. (A) Module-trait relationships (MTRs) between detected modules and disease severity of COVID-19. Module-trait relationships MTRs are obtained by calculating the correlation between the traits and the module eigengenes. The red and blue colors indicate strong positive correlation and strong negative correlation, respectively. Rows represent module eigengene (ME) and columns indicate disease severity of COVID-19. Asterisks corresponds significant highly-correlated values. (B) Eigengene adjacency heatmap indicate relationship among all the modules.
Functional Enrichment Analysis of Highly-Correlated Modules
In order to understand the biological performance of significant highly-correlated modules with disease severity of COVID-19, functional enrichment analysis was performed and a total of 342 biological process and 93 KEGG pathways were significantly enriched in the respective modules. The turquoise module had the highest number of enriched terms and pathways, including 295 biological processes and 82 KEGG pathways. The most significant GO term and KEGG pathway in the turquoise module were “mitochondrial translational elongation (GO:0070125)”, “translational termination (GO:0006415)”, and “negative regulation of type I interferon-mediated signaling pathway (GO:0060339)”. On the other hand, 13 biological processes and 8 KEGG pathways were significantly enriched in the purple module. The most significant GO term and KEGG pathway in the purple module were “Fc gamma R-mediated phagocytosis” (GO:0006968, Adjusted P value = 2.27E-09) and “Autophagy” (Adjusted P value = 2.09E-06), respectively. Based on the functional enrichment analysis, among the significant highly-correlated modules with disease severity of COVID-19, blue, brown, lightgreen, grey60, lightyellow, pink, midnightblue, purple, and turquoise were associated with COVID-19 mechanisms. The identified modules in the COVID-19 samples with different colors as a heatmap and the relationship between them are shown presented in Figure 6B. Figure 7 was constructed by ClueGO plugin and shows GO (describes our knowledge of the biological domain with respect to three aspects: Molecular Function, Cellular Component, and Biological Process (Supplementary Table 10).
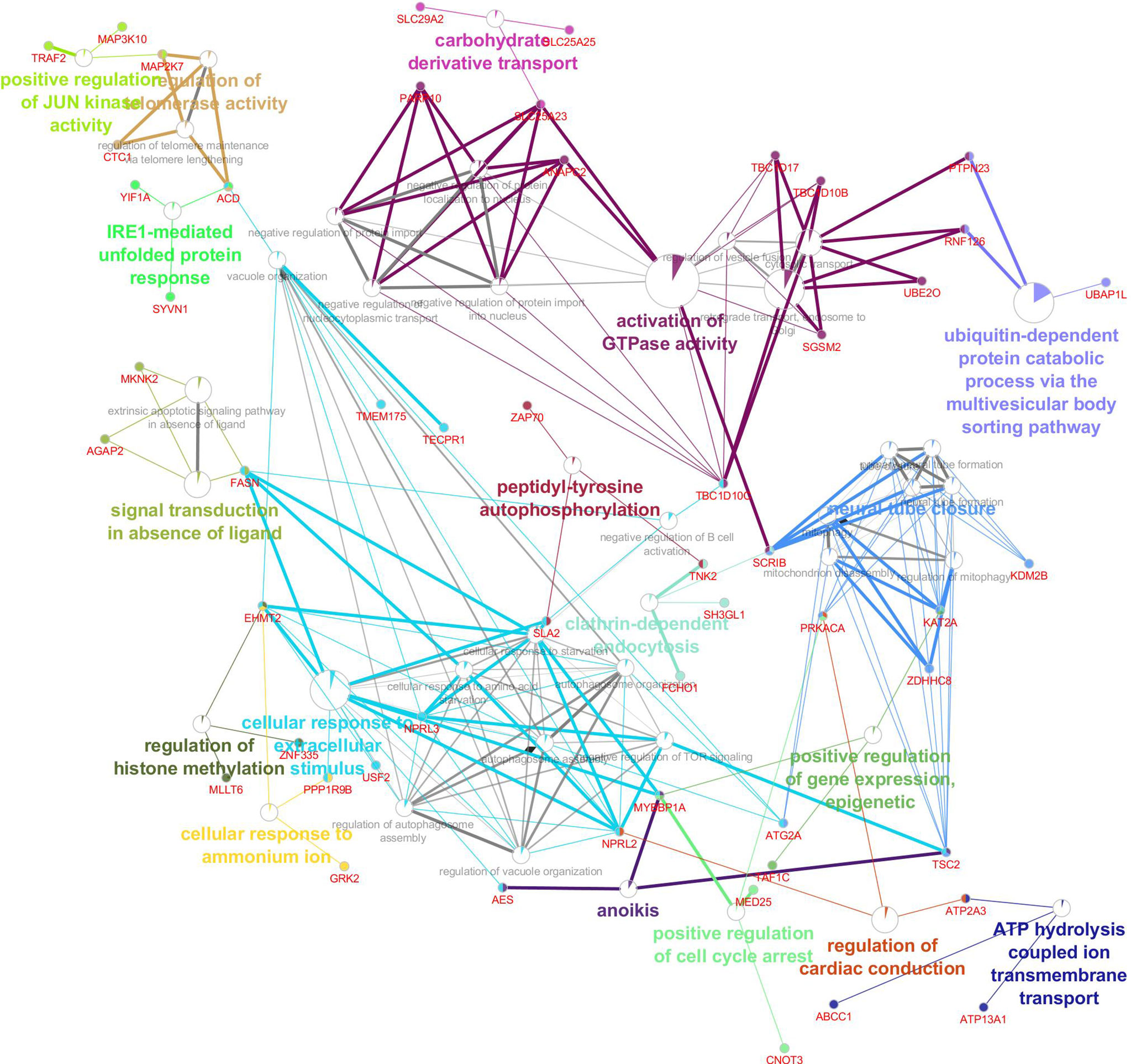
Figure 7 The Gene, Gene Ontology and pathway, related modules involved in the disease severity of COVID-19.
Discussion
COVID-19, a global pandemic caused by SARS-CoV-2, has caused severe pulmonary conditions and many deaths (65–67). The prevalence of SARS-CoV-2 infection is rapidly increasing, and despite several vaccines, no cure is available (68–70). Development of potential therapies requires an understanding of molecular mechanisms of the disease and patient-pathogen interactions (6, 7). In this study, bioinformatics and systems biology approaches were used to elucidate molecular regulatory mechanisms responsible for COVID-19. Since the main aim of the study were to find key and hub high-traffic genes affecting the disease at the systems level, we used the Module Preservation approach. As well, all the modules and genes identified by the Module-trait Relationships approach were covered by the main study approach. Although the second approach requires a sufficient number of samples for each attribute, it can also be used as a validation of the MP method. It should also be noted that more studies are needed to increase the accuracy of the MTR analysis. As well, the main purpose of the study is to systematically study the COVID-19 in terms of the static aspect of biological processes. Based on differential co-expression network analyses, among the 21 modules identified in healthy controls, network connectivity patterns of 15 modules (72%) changed due to SARS-CoV-2 infection. That 15 of 21 modules were affected indicated the ability of SARS-CoV-2 to cause systemic disturbances in gene networks of healthy individuals. Investigating the function of these non-preserved modules should provide insights into molecular mechanisms and host-pathogen interactions, as well as identifying key genes, representing an important step for development of effective treatments. Moreover, in functional enrichment analyses, among the 15 non-preserved modules between healthy and COVID-19 samples, 9 modules including blue, brown, grey60, lightgreen, lightyellow, midnightblue, pink, purple, and turquoise had direct biological relationships with host immune responses and COVID-19 development.
Blue Module
Based on GO and KEGG pathway analyses, the blue module was clearly closely related to SARS-CoV-2 pathogenesis. Co-expressed genes of this module were significantly enriched in KEGG pathways related to host inflammatory responses, including: Toll-like receptor signaling pathway; C-type lectin receptor signaling pathway; MAPK signaling pathway; NF-kappa B signaling pathway; and IL-17 signaling pathway.
Toll-like receptors (TLRs) are the most important pattern recognition receptors (PRRs); they recognize pathogen-associated molecular patterns (PAMPs), activate the innate immune system and regulate secretion of proinflammatory cytokines and the host inflammatory response (71, 72). Activation of the Toll-like receptor signaling pathway during SARS-CoV-2 infection leads to activation of a cascade of downstream pathways, thereby activating the MAPK and NF-kappa B signaling pathways, increasing production of type I interferons and proinflammatory cytokines such as IL-1β, IL6, and TNF-α (73–77). C-type lectin receptors, another type of PRRs, are involved in the proinflammatory response; their role has been investigated during Middle East respiratory syndrome coronavirus (MERS-CoV) and SARS-CoV-2 infections (78, 79). Moreover, SARS-CoV-2 ORF8 increased the expression level of proinflammatory cytokines by activating the IL-17 signaling pathway (80). Additionally, in the blue module, the biological processes associated with the cytokines included: positive regulation of cytokine production (GO:0001819); cellular response to cytokine stimulus (GO:0071345); cytokine-mediated signaling pathway (GO:0019221); inflammatory response (GO:0006954); and positive regulation of T cell cytokine production (GO:0002726). The vast cytokine release from the immune system in response to SARS-CoV-2 infection often exacerbates the proinflammatory response, with a cytokine storm in the lung that causes acute respiratory distress syndrome (ARDS), acute lung injury (ALI), decreased lung function, and host death (81, 82). Therefore, development of effective strategies for controlling or preventing excessive inflammation through these key pathways in the blue module, which regulate cytokine production, could enhance survival of COVID-19 patients (80, 83–85).
In the blue module, other important KEGG pathways and biological processes associated with the immune system included: TNF signaling pathway; Apoptosis; p53 signaling pathway; Influenza A; HIF-1 signaling pathway; Viral protein interaction with cytokine and cytokine receptor; and positive regulation of programmed cell death (GO:0043068).
The TNF signaling pathway is another key pathway responsible for the cytokine storm and lung injury during SARS-CoV-2 infection. Blocking the NFkB1 inhibitory protein (IkB) induces the NFkB1 transcription factor, which increases transcription of cytokine genes (86–88). This pathway has been considered an attractive therapeutic target for COVID-19-induced lung injury (86, 89).
The SARS-CoV-2 and MERS-Cov infections reduce percentages of peripheral blood mononuclear cells (PBMCs), e.g., T cells, by inducing apoptosis and contributing to disease pathogenesis (90, 91). In addition, induction of apoptosis in PBMCs during COVID-19 is more marked in severe clinical cases (90, 92). Moreover, severe COVID-19 is associated with numerous changes in PBMCs, e.g., lymphopenia (93) and lymphocyte apoptosis (especially T cells), with lymphopenia associated with severe COVID-19 (94, 95). The SARS-CoV-2-induced cytokine storm can exacerbate apoptosis in T cells, reducing their numbers in COVID-19 patients (96). More research is needed to mitigate lymphocyte apoptosis and lymphopenia in COVID-19 patients (94). Furthermore, in agreement with our research, in a transcriptomic study, in addition to apoptosis, the p53 signaling pathway was highly enriched in PBMCs of the COVID-19 group and may be associated with lymphopenia in COVID-19 patients (97).
The exacerbated inflammatory response due to severe/critical COVID-19 leads to ARDS, which can lead to multi-organ failure (MOF) and death (98). This imbalanced inflammatory response (cytokine storm) increases disease severity and is associated with high concentrations of circulating proinflammatory cytokines, e.g., IL6, TNF, and IL1B in lung tissue (93, 99–102). Interestingly, in this study, IL6, TNF, and IL1B were key hub-high traffic genes in the blue module. Interleukin-6 (IL6), a proinflammatory cytokine that enables virus to enter host cells and proliferate, is an important prognostic biomarker and a powerful predictor of COVID-19 mortality (103, 104); it is significantly increased in ICU patients, and was highly significantly correlated with COVID-19 mortality (105–107). Additionally, in an extensive proteomic study, IL6 was an important candidate associated with disease severity (108). Given the key role of IL6 in SARS-CoV-2 pathogenesis, blocking IL6 signaling may prevent hyperinflammation and increase survival in COVID-19 patients (109–111). For example, tocilizumab (a monoclonal antibody against IL6) and convalescent plasma therapy (CPT) reduced IL6 concentrations, controlled and relieved inflammation, and managed the cytokine storm in COVID-19 patients (112–115). Moreover, clinical trials are underway to investigate effects of IL6 receptor and IL1B signaling blockade on COVID-19 treatment (100).
Tumor necrosis factor (TNF) is another key proinflammatory cytokine involved in COVID-19 hyperinflammation, as COVID-19 patients have increased TNF concentrations (116). TNF has a special nature and biological features that make it a promising target for treatment of COVID-19 (117). Anti-TNF therapies can manage inflammation in many human inflammatory diseases, e.g., chronic kidney disease (CKD), rheumatoid arthritis (RA) (118, 119), Crohn’s disease (120), psoriasis, psoriatic arthritis (121), and sepsis (122). There is ample evidence that anti-TNF therapies, in addition to reducing TNF concentrations and preventing inflammation, have additional therapeutic effects, including: suppressing formation of new blood vessels by modulating angiogenic vascular endothelial growth factor (VEGF) in patients with RA (123); reducing synovial expression of chemokines such as interleukin-8 (IL8) and monocyte chemotactic protein-1 (MCP-1) in RA patients (124); downregulating production of interleukin-18 (IL18) in RA patients (125); and reducing expression of adhesion molecules (126). Anti-TNF therapies reduce secretion of proinflammatory cytokines to normal (IL1β) or sub-normal (IL6 and IFN-γ) in RA patients (127). Therefore, anti-TNF therapies may reduce many pathogenic proinflammatory cytokines during SARS-CoV-2 infection and be an effective immunomodulatory approach for treatment of COVID-19 patients (128, 129). In agreement with this study, these findings indicated the importance of IL6, TNF and IL1B hub-high traffic genes, considered potential targets (individually or in combination) for development of effective therapeutic immunomodulation strategies to manage COVID-19 hyperinflammation.
Identified hub-high traffic genes in the blue module included several important transcriptional regulatory factors, i.e., NFKB1and HIF1A. The nuclear factor-kappa b subunit-1 (NFKB1), an important transcription factor belonging to the NF-κB family, stimulates transcription of proinflammatory cytokines such as IL6, TNF‐α, and IL1 as well as chemokines such as IL8 (CXCL8), all with major roles in causing a COVID-19-induced cytokine storm (86, 87, 130, 131). The inflammation regulator NFKB1 was significantly upregulated in response to SARS-CoV-2 infection; transcriptomic studies revealed its key role in regulation of differentially expressed genes (DEGs) between healthy individuals and patients with mild or severe COVID-19 (7, 10, 132–136). Furthermore, by targeting NFKB1, miR-9 regulates inflammatory pathways related to COVID-19 pathogenesis (137). Moreover, by binding to NFKB1, miR-27a-3p suppressed NF-κB activation and reduced acute lung injury in an animal model (138). These results demonstrated the vital role of the NFKB1 hub-high traffic TF in the inflammatory response during COVID-19, consistent with targeting NFKB1 as a potential treatment strategy for severe COVID-19 cases (85, 139). Fluoxetine, tiotropium, and andrographolide targeted NFKB1, suppressed inflammation, and reduced the cytokine storm in COVID-19 patients (86, 140, 141). Additionally, vitamin D blocked the TNF-induced NFkB1 signaling pathway and blocked the cytokine storm in severe COVID-19 infections.
In severe cases of COVID-19, a hypoxic microenvironment activates Hypoxia Inducible Factor 1 Alpha (HIF1A), a master regulator that activates, recruits, and stabilizes immune cells such as macrophages and neutrophils at the site of inflammation; furthermore, these cells secrete inflammatory cytokines, causing a cytokine storm (142). Activation of HIF1A upregulates VEGF, which increases vascular leakage and destroys alveolar-interstitial-endothelial epithelial complex barriers (142). Conversely, activation of HIF1A, induces autophagy, a pathway through which SARS-CoV-2 increases its proliferation and progression in host cells (142, 143). Moreover, in agreement with our results, in a transcriptomic study, HIF1A had the highest connections in the GO, KEGG, and PPI networks of various clinical stages of COVID-19 (33). Thus, HIF1A inhibitors may interfere with processes that promote COVID-19 pathogenesis (144, 145).
Other critical hub-high traffic genes in the blue module included EP300 (146, 147), CDK1 (4, 148), VEGFA (149–151), CTNNB1 (151, 152), IL10 (9, 153–155), SOCS1, SOCS3 (156), SIRT1 (157, 158), TFRC (159–161), HSP90AB1 (162), CYCS (163), EZR (164), TNFAIP3, ICAM1 (10), and PIK3R1 (139) as well as TFs such as ATF4 (165), ATF3 (166, 167), and BCL6 (152) which have important roles in pathogenesis of SARS-CoV-2, and some of which are potential therapeutic targets. For instance, SOCS1/3 antagonists may be prophylactic or therapeutic against the COVID-19 pandemic (156). Moreover, a significant increase in expression of endothelial cell adhesion molecules e.g., intercellular adhesion molecule 1 (ICAM1) in patients with severe COVID-19, is associated with COVID-19 severity and may cause coagulation disorders (168). Additionally, increased expression of ICAM1 in COVID-19 patients contributes to replication of SARS-CoV-2 and provides a favorable environment for its survival in humans (135). Therefore, ICAM1 protein can be considered a key target for treatment of COVID-19 patients (169, 170).
Brown Module
The brown module was significantly enriched in immune-related pathways, including “NOD-like receptor signaling pathway”, “Necroptosis”, “tumor necrosis factor-mediated signaling pathway (GO:0033209)”, “interferon-gamma-mediated signaling pathway (GO:0060333)”, “T cell receptor signaling pathway (GO:0050852)”, “neutrophil mediated immunity (GO:0002446)”, and “neutrophil activation involved in immune response (GO:0002283)”.
NOD-like receptors (NLRs) are a specialized group of cytoplasmic PRRs with an important role in pathogenesis of a variety of inflammatory human diseases by regulating nuclear factor–kappa B (NF-κB) signaling, proinflammatory cytokines such as IL1B, and cell death (171). Moreover, in recent COVID-19 studies, the NOD-like receptor signaling pathway was among active pathways in response to the SARS-CoV-2 infection, implying this pathway may be a central mediator of severe COVID-19 (172, 173). Interestingly, this pathway and some of its members were reported to be important targets for reducing the cytokine storm in patients with severe COVID-19 (174–176).
Necroptosis or inflammatory cell death is immunogenic cell death in response to viral infection; it involves various processes, including clearance of virus-infected cells, inflammation, metabolic abnormalities, and embryonic development (177, 178). SARS-CoV-2 induces necroptosis through some of its viral proteins (179). Despite the immunological role of necroptosis, this pathway also contributes to pathogenesis of COVID-19. For example, synergism of interferon-gamma and tumor necrosis factor-mediated signaling can perpetuate a COVID-19-induced cytokine storm by stimulating programmed cell death (apoptosis and necroptosis) and increasing mortality (180). Moreover, increased secretion of mature IL1B through the necroptosis pathway can exacerbate the inflammatory response during SARS-CoV-2 infection (181). In addition, SARS-CoV-2 internalization by platelets induced them to undergo necroptosis and apoptosis, which can contribute to thrombosis (182). Furthermore, there is a major role of necroptosis in lung damage of COVID-19 patients (181). Therefore, blocking inflammatory cell death such as necroptosis may benefit COVID-19 patients by limiting tissue inflammation/injury (180). In this regard, Necrostatin-1 (Nec-1), an inhibitor of necroptosis, protected against complications of COVID-19 (183).
T-cells, lymphocytes in the cell-mediated adaptive immune response, are involved in viral clearance and long-term antiviral immunity (184). However, SARS-CoV-2 counteracts T-cell activity through specific immune escape mechanisms, e.g., T-cell apoptosis (90) or direct interactions with T-cell activator molecules (185). Moreover, severe COVID-19 is associated with dysregulation of T-cells, causing lymphopenia and exhaustion of CD4+ and CD8+ T cells (186–188). Conversely, activation of the T cell receptor signaling pathway in response to candidate drug treatment in COVID-19 patients indicates a key role of T-cells for limiting of SARS-CoV-2 infection during recovery (189). Despite their critical immunological role, T-cells may contribute to the pathogenesis of SARS-CoV-2. For instance, the inflammatory response and activation of T cells (especially T-helper 17 cells) can synergistically exacerbate the disease and prolong SARS-CoV-2 infection (15). Treatment strategies to activate/block T-cells in COVID-19 patients have been reviewed (190).
A major contributor of SARS-CoV-2 pathogenesis in the lungs of COVID-19 patients is excessive activation of neutrophils (191). Formation of neutrophil extracellular traps (NETs) by SARS-CoV-2-activated neutrophils can exacerbate inflammation-associated lung damage and cause severe pulmonary fibrosis in COVID-19 patients (192, 193). Moreover, high levels of NETs caused lung epithelial cell death in vitro, implying increased risk of mortality in COVID-19 patients (194). Conversely, formation of NETs by neutrophils in response to SARS-CoV-2 infection caused rapid vascular occlusion, altered microcirculation (195), and induced thrombosis (196). Therefore, NET-targeting approaches such as the use of NET-inhibitory factor (nNIF), could be a novel strategy to reduce lung damage and thrombotic responses during COVID-19 disease (197).
We also identified several significant terms related to mitochondrial activity in the brown module. These terms included “Oxidative phosphorylation”, “mitochondrial translational elongation (GO:0070125)”, “mitochondrial translational termination (GO:0070126)”, “mitochondrial ATP synthesis coupled electron transport (GO:0042775)” and “mitochondrial respiratory chain complex I assembly (GO:0032981)”. Mitochondria have a major role in maintaining cellular immunity, homeostasis and cell survival (198). Interestingly, there is emerging evidence that SARS-CoV-2 highjacks mitochondria in immune cells, manipulates mitochondrial activity to its advantage, and provides favorable conditions for viral replication within the mitochondrial structure (199). After entering the cell through the angiotensin-converting enzyme-2 (ACE-2) host receptor, the SARS-CoV-2 sends its genomic RNAs toward the mitochondria and, after manipulating it, affects various processes, including stimulation of cytokine production, mitophagy, iron storage, and platelet coagulation (200). Furthermore, SARS-CoV-2 may use mitochondrial-derived bilayer vesicles to hide within the cell (201). Additionally, host mitochondrial manipulation by SARS-CoV-2 ORF-9b released mitochondrial DNA (mt-DNA) into the cytoplasm, which activated an mtDNA-induced inflammasome, thereby suppressing innate and adaptive immune responses (201). Moreover, SARS-CoV-2 targets the oxidative phosphorylation pathway, causing a massive increase in reactive oxygen species (ROS) which increases TNF and IL1B, followed by hyperinflammation (202–205). Another mechanism of mitochondrial dysfunction by SARS-CoV-2 is excessive ferritin (hyperferritinemia) in mitochondria, causing oxidative stress (206). Furthermore, mitochondrial oxidative stress can impair glucose tolerance of cells and cause complications in diabetic patients (200). Given the importance of mitochondria in the pathogenesis of COVID-19, a clear understanding of biological interactions between mitochondria and the virus would be important to develop anti-SARS-CoV-2 strategies.
Previous COVID-19 studies reported that hub-high traffic genes in the brown module, such as RBX1 (207–210), PSMA3 (211), PSMA6 (212), PTEN (213), VPS29 (214, 215), SNRPE (216), PSMA4 (217), TXN (218), PTGES3 (219, 220), RHOA (57, 221, 222), CANX (209), B2M (223), GDI2 (57, 224, 225), TXNL1 (226), SUMO1 (147, 227, 228), PSMC2 (229), REEP5 (230), DECR1 (108), RAB10 (224), PRDX3 (231, 232), ACTR2 (215), TNFSF13B (192, 233, 234), and ARPC3 (215), have a central role in host-SARS-COV-2 interactions. For example, ARDS and ALI in patients with severe COVID-19 were directly associated with increased expression of the PTEN and RHOA hub-high traffic genes, respectively (213, 222). Additionally, a recent multi-omics study suggested the GDI2 hub-high traffic gene as a potential biomarker for segregating COVID-19 positive cases (225). Moreover, SARS-CoV-2 disrupts PPAR-γ activity by suppressing SUMO1, resulting in a hyperinflammatory response in severe COVID-19 patients (147).
We identified the caspase-1 (CASP1) hub-high traffic gene in the brown module; it has a key role in the pathogenesis of SARS-CoV-2 and is associated with the severity of COVID-19 (235). Significant increases in the CASP1 expression in patients with acute COVID-19 were reported in previous transcriptomic studies (236, 237). Indeed, activation of CASP1 by activating inflammasomes in response to the SARS-CoV-2 infection activates IL1B and IL18 and contributes to hypercytokinemia in COVID-19 patients (238–240). Besides, activation of CASP1 promotes pyroptosis (a highly inflammatory and Caspase-1-dependent form of programmed cell death), which can lead to secretion of a wide range of inflammatory mediators (241, 242). Melatonin suppressed the lung cytokine storm in COVID-19 patients by reducing CASP1 expression (243).
Grey60 Module
Significant functional terms such as “B cell receptor signaling pathway”, “B cell activation (GO:0042113)”, and “regulation of B cell proliferation (GO:0030888)” demonstrated that the grey60 module was closely related to the humoral adaptive immunity. In agreement with our results, similar transcriptomic and systemic studies of COVID-19 reported enrichment of B cell receptor signaling pathway, B cell activation, and B cell proliferation pathways during SARS-CoV-2 infection (7, 244–246). Furthermore, B cell depleted patients infected with SARS-CoV-2 had prolonged disease (247). Remarkably, in 1 study, IgM memory B cells were commonly depleted in COVID-19 patients, which increased mortality (248). Moreover, given the relationship between B cell subset frequencies and clinical/laboratory parameters, these cells may be potential biomarkers for predicting the clinical outcome of COVID-19 (249). These findings supported the special importance of B cells and cell pathways during the humoral immune response in COVID-19 patients. Interestingly, we identified several hub-high traffic genes involved in regulating activity/development of B cells such as CD19 (250), IGLL5 (251, 252), CD79B (253), CD79A (254), CD22 (255), PNOC (256), and POU2AF1 (257), with a central role in information transfer within the grey60 module during humoral immunity in response to SARS-CoV-2 infection.
Lightgreen Module
Investigation of KEGG pathways and biological processes of the lightgreen module revealed that co-regulated genes of this module were highly enriched in pathways such as “Th17 cell differentiation” and “Antigen processing and presentation”, as well as biological process such as “regulation of macrophage cytokine production (GO:0010935)”. As discussed in the “brown module” section, T cells can have dual actions; despite a key role in the immune response, they may also contribute to disease pathogenesis. Release of a wide range of cytokines (especially IL6) in response to SARS-CoV-2 infection enhanced differentiation of naive T cells into the T-helper 17 (TH17) phenotype (258–261). TH17 cells have a major role in inducing the proinflammatory effects of cytokines (G-CSF, IL1B, IL6, and TNF) and chemokines (KC, MIP2A, IL8, IP10, MIP3A) through secretion of inerleukin-17 (IL17), thus contributing to the cytokine storm and subsequent ARDS in COVID-19 patients (262–264). Moreover, an increase in the percentage of TH17 cells in response to the SARS-CoV-2 infection caused pregnancy complications, e.g., miscarriage, preterm labor, and preeclampsia in pregnant women with COVID-19 (265). Conversely, aberrant Th17 cell differentiation in COVID-19 patients increased the risk of autoimmune disorders, e.g., Guillain-Barré syndrome, multiple sclerosis, and rheumatoid arthritis (173, 266). Modulating compounds aimed at reducing the percentage of TH17 cells may suppress inflammation in COVID-19 patients (267).
Additionally, some lightgreen module hub-high traffic genes, including GLUL (268), ACSL1 (269), TRIB1 (236), TUBA1B (270), PLEK (271, 272), HSPA5 (273, 274), and IRAK3 (275) may contribute to the pathogenesis of COVID-19. For example, SARS-CoV-2 spikes can drive the infection process of host cells by binding to cell-surface heat shock protein A5 (HSPA5) receptor (273, 276). However, targeting this receptor using natural compounds, e.g., phytoestrogens or estrogens, can prevent SARS-CoV-2 from binding to stressed cells and thus limit infection (277). Conversely, the IRAK3 hub-high traffic gene is an important inflammatory mediator associated with asthma susceptibility (278, 279) and SARS-CoV-2 increases risk of asthma in COVID-19 patients by increasing expression of this gene (275). Additionally, a network-based study recently suggested the IRAK3 hub-high traffic gene as a potential therapeutic target against COVID-19 (280). Moreover, we identified aryl hydrocarbon receptor (AHR) hub-high traffic TF among the central genes of the lightgreen module. Increased expression of this transcriptional regulatory factor in response to the SARS-CoV-2 infection interfered with antiviral immunity in the host, as AHR suppressed production of type I interferons in the COVID-19 patients and increased SARS-CoV-2 replication in host cells (281). Therefore, pharmacologic AHR blockade approaches may be effective to enhance host antiviral immunity and reduce viral replication in COVID-19 patients (281).
Lightyellow Module
Functional examination of the lightyellow module suggested that this module was only enriched in the “Natural killer cell mediated cytotoxicity” KEGG pathway. Additionally, some important hub-high traffic genes involved in the cytotoxic activity of natural killer cells during COVID-19 were NCAM1 (252, 282), GZMA, NKG7 (283, 284), KLRD1 (285, 286), GZMB (150, 287, 288), and PRF1 (289–291). Natural killer (NK) cells are key innate immune cells with a central role in killing virus-infected cells (292). Decreased NK cells during SARS and SARS-CoV-2 infections, followed by an increase during the recovery period indicates an inverse relationship between number of NK cells and disease severity (188, 293, 294), with a decrease in their number early in the disease process promoting progression of coronavirus infection (294).
Among the hub-high traffic genes in the lightyellow module, GZMB and PRF1 had the most important role in NK cells cytotoxicity. Perforin glycoprotein, expressed by the PRF1 gene, has an important role in the cytolytic activity of cytotoxic T cells and NK cells and causes pores in the membrane of target cells (295, 296). Granzyme B is a key serine protease expressed in cytotoxic T cells and NK cells by the GZMB gene; it enters the target cells through membrane pores created by perforin and induces apoptosis, eliminating infected cells (297, 298). Interestingly, decreased expression of both PRF1 and GZMB hub-high traffic genes in severe COVID-19 patients lead to dysfunction of NK cells cytotoxicity, indicating that both genes are essential for NK cells activity (155, 299). Conversely, genetic mutations in the PRF1 gene impaired NK cells cytotoxicity, implicating this gene in the antiviral activity of NK cells (300–302). Given the importance of NK cells and their genes/proteins in antiviral activity, as well as their reduction in COVID-19 patients, development of treatment strategies to restore NK cells may control infection in the early stages of COVID-19 (303). Moreover, other genes in the lightyellow module, e.g., ERBB2 (151) and ADRB2 (275, 304), as well as TBX21 (305–307) hub-high traffic TF, have critical roles during SARS-CoV-2 infection. For instance, in addition to the IRAK3 hub-high traffic gene (described in the lightgreen module section), increased ADRB2 hub-high traffic gene expression during SARS-CoV-2 infection also increased the risk of asthma in COVID-19 patients (275).
Midnightblue Module
The only significant enriched term in midnightblue was “regulation of immune response (GO:0050776)”. In this module, we also identified several hub-high traffic genes that were crucial in regulating the host immune response during COVID-19. These hub-high traffic genes included CDKN2A (308, 309), OASL (282, 310), CCL5 (311–316), KIF2C (4), and CD8A (317). Among them, the CCL5 hub-high traffic gene has the most important role in regulating the inflammatory response and the host immune system; however, its excessive expression can amplify inflammatory responses toward immunopathology (e.g., cytokine storm) (311, 314). C-C motif ligand 5 (CCL5) also known as RANTES, is a chemotactic molecule in the CC family of inflammatory chemokines, expressed by virus-infected airway epithelial cells and macrophages, which promotes trafficking of proinflammatory leukocytes to the site of infection and stimulates cytokine production (314, 318–320). A massive increase in secretion of pro-inflammatory molecules, including chemokines such as CCL5 and other cytokines in response to SARS-CoV-2 infection, cause a cytokine storm (84, 321–323). Multiple-fold increases in CCL5 expression in severe COVID-19 patients compared to healthy individuals are well documented (311, 312, 315, 324). Additionally, there was a significant increase in CCL5 expression in non-surviving COVID-19 patients compared to severe, mild and healthy groups (313). Furthermore, high levels of CCL5 cause liver disorders and acute kidney injury (AKI) in COVID-19 patients (314, 318, 325). Therefore, early intervention to prevent overexpression of CCL5 can restore immune balance in COVID-19 patients and prevent disease progression (311, 314). Moreover, 2′–5′‐oligoadenylate synthetase‐like (OASL), the other hub-high traffic gene of midnightblue module, is an interferon-stimulating gene with a key role in antiviral defense mechanisms (326) and with potential as a diagnostic biomarker for COVID-19 (327).
Pink Module
In the pink module, genes were observed in functional enriched terms such as “Coronavirus disease”, “Ribosome”, “ribosome assembly (GO:0042255)”, “mitochondrial transport (GO:0006839)”, and “translation (GO:0006412)”. Ribosomes are complex molecular machines that synthesize proteins by translating mRNA into polypeptides (328). Interestingly, in the infection stage, nonstructural protein 1 (Nsp1) from SARS-CoV-2 bound to the host ribosome and hijacked it to (1) disrupt the mechanism of host cellular translation and protein production (2), inhibiting all cellular antiviral defense mechanisms (3), initiating translation of viral mRNAs and subsequently viral protein production, and (4) increasing viral replication efficiency (329–335). Therefore, inhibitory mechanisms to target ribosome-SARS-CoV-2 interactions may be used to treat COVID-19 (331). Additionally, some pink module hub-high traffic genes, such as RPL9, RPS27A, RPL26, RPS12 (336), RPS6 (228), RPL5, RPL7 (337), RPGR (173), and RPS20 (338) were highly enriched in the ribosome pathway and involved in SARS-CoV-2-ribosome interactions. For example, the increase in ribosomal protein L9 (RPL9) expression was attributed to SARS-CoV-2 hijacking the host translation machine (339).
We identified the heat shock protein 90 alpha family class A member 1 (HSP90AA1) hub-high traffic gene in the pink module, which has an important role in development of SARS-CoV-2 infection (340) and has a significant increase in expression in severe COVID-19 patients compared to the non-severe group (341, 342). Furthermore, this hub-high traffic gene is also involved in comorbidities associated with COVID-19, e.g., cardiovascular diseases (CVDs) and has been suggested as a potential therapeutic target for these complications (139, 343, 344). We also identified mitochondrial 60-kDa heat shock protein (HSPD1) hub-high traffic gene in the pink module. High concentrations of circulatory HSPD1 in severe COVID-19 patients are associated with cardiac failure and are a potential clinical biomarker for heart disorders in COVID-19 patients (345).
Other hub-high traffic genes in this module, such as BTAF1 (216), EZH2 (346), NPM1 (347), TPT1 (151, 269, 348), LDHA (217, 268), PTPRC (189), SOS1 (349, 350), DDX5 (351, 352), BCL10 (162, 353), EEF1B2 (354), CALM1 (344), EIF4A2, EIF4B (336, 355, 356), EEF1A1 (269, 354), HNRNPA1 (228, 347), and IFNG (8, 357, 358), have important roles in development/inhibition of COVID-19. Overexpression of SARS-CoV-2 ORF6 caused HNRNPA1 nuclear accumulation, subverting the host mRNA export system and reducing nucleus size (359). Moreover, IFNG hub-high traffic gene neutralizers have been predicted as antagonists of COVID-19 biology (8, 357).
Purple Module
The immune-related enriched pathways in the purple module included “Fc gamma R-mediated phagocytosis” and “Autophagy”; these 2 pathways were significantly enriched in previous COVID-19 studies (189, 360, 361). Autophagy is an evolutionarily conserved mechanism to selectively eliminate damaged organelles, misfolded proteins, and intracellular pathogens (362). Components are enclosed in an autophagosome, which fuses with a lysosome to form an autolysosome that breaks down its contents (363). However, there is growing evidence that coronaviruses, such as SARS-CoV-1, MERS-CoV, and SARS-CoV-2, manipulate/hijack the autophagy pathway to accelerate their replication and complete their life cycle (364–368). Accordingly, inhibitory mechanisms and blockade of the autophagy pathway may be a novel therapeutic strategy (369–373).
Additionally, some of the purple module hub-high traffic genes, such as MAPK1 (189, 374), CUL2 (210), CMTM6 (162), TXNRD1 (375), RAB1A (376), DICER1 (377), RAB5A (209), HSP90B1 (343), MAGT1 (378), ADAM10 (379, 380), SNX2 (89), OLA1 (381), SPTLC1 (382), SH3GLB1 (383), TIMM10B (384), and CREB1 (385) hub-high traffic TF, which are central for information exchange in this module, are potential targets for development of COVID-19 therapeutic strategies. Among these, the mitogen-activated protein kinase 1 (MAPK1) hub-high traffic gene is a potential core target for many anti-COVID-19 therapeutic strategies (189, 374, 386–390). Perhaps targeting the MAPK1 hub-high traffic gene with guanfacine or desipramine may be effective for treating COVID-19 associated comorbidities, such as diabetes, CVDs, and chronic kidney diseases (CKDs) (139). Interestingly, the ras-related protein Rab-5A (RAB5A) is involved in various autophagy processes such as autophagosomal maturation and early autolysosome formation, and the SARS-CoV-2 enhances autophagy by increasing expression of this gene to accelerate viral replication (391). Furthermore, RAB5A is a key host protein for interaction with SARS-CoV-2 and may be an important target for inhibiting COVID-19 progression (209).
Turquoise Module
Based on the functional terms of the turquoise module such as “mitochondrial translational elongation (GO:0070125)”, “translational termination (GO:0006415)”, and “negative regulation of type I interferon-mediated signaling pathway (GO:0060339)”, this module may have an important role in pathogenesis and development of SARS-CoV-2. In agreement with our results, COVID-19 suppressed type I interferon signaling and impaired their responses (6, 392, 393). Additionally, some SARS-CoV-1, MERS-CoV, and SARS-CoV-2 proteins decreased production and impaired type I interferon signaling (394). Accordingly, disruption of the type I interferon signaling, key antiviral mediators, contributed to the pathogenesis of COVID-19 (395). Indeed, impaired type I interferon signaling and exacerbation of the inflammatory response are hallmarks of severe/critical COVID-19 (234, 396). Therefore, type I interferons may be an intervention for SARS-CoV-2 infection (397).
Additionally, in terms of individual genes in the turquoise module, we identified hub-high traffic genes such as UTP14A (398), RUVBL2 (139), PRKCD (280, 399), KEAP1 (400–402), CEBPA (TF) (403), RPL26L1 (404), PTPN6 (255), PPARA (TF) (405), TNFRSF1A (406–408), IKBKB (275, 409), TFEB (TF) (410, 411), PSMD4 (412), and ARHGAP1 (413), important components in immunopathogenesis of SARS-CoV-2. For example, the TNF receptor superfamily member 1A (TNFRSF1A) hub-high traffic gene may be a biomarker of mortality in severe COVID-19 patients, as increased expression of this gene was significantly correlated with mortalities (414). We also identified tumor protein p53 (TP53) hub-high traffic TF in the turquoise module, with an important role in the pathogenesis of COVID-19 (415). Moreover, in an animal model of COVID-19, ALI in the infected group was associated with an increase in TP53 expression (416). Besides, 1 study reported that an increase in the expression of TP53 led to the induction of apoptosis in PBMCs and thus reduced their frequencies in COVID-19 patients (97). Furthermore, this TF is associated with some human inflammatory diseases such as RA (417). In agreement with us, this TF was identified as a hub gene in several network-based COVID-19 studies (347, 418–421). Therefore, this TF may have an immunoregulatory role during SARS-CoV-2 infection and be a potential therapeutic target.
As well as, different proteomic databases were explored. More data bases were related to SARS-CoV-2 structure and different situations and tissues. Therefore COVID-19 Immune Atlas (https://covid19cellatlas.com/#/) was selected. Hospitalized COVID-19 patients and age-matched healthy controls were recruited. No differences in age, sex, body mass index (BMI), viremia, or time from symptom debut until hospital admission were present between moderate and severe patients. Based on different cells in the Atlas below results were achieved:
Both moderate and severe COVID-19 patients were characterized by the progressive expansion of CD16 neutrophils, which also expressed lower levels of CD177, CD11b, and CD62L and higher levels of CD66b and LOX-1, a phenotype compatible with neutrophil immaturity. Intriguingly, a positive correlation between type 1 inflammatory mediators (e.g., IFNγ and CXCL10) and eosinophil activation was observed, suggesting that, particularly in moderately affected patients, part of the granulocyte compartment could be actively participating in the efficient viral clearance, similarly to what can occur upon influenza infection.
CXCR4 is one of the most highly expressed basophil receptors in COVID-19 patients and might be implicated in basophil transendothelial migration. CD63 expression on basophils can be induced by cross-linking of CD62L and CD11b, among other stimuli. Therefore, the up-regulation of CD62L, CD63, CD11b, and CXCR4 on basophils observed during the acute phase of COVID-19 and their normalization after viral clearance might imply a role of this phenotype in COVID-19 pathophysiology.
We found increased levels of CXCR4 in both moderate and severe patients. Other recent studies have also observed increased levels of CXCR4 with levels higher in severe than in non-severe COVID-19 patients. We also observed a characteristic increase in ASC expansion during acute COVID-19 in both severe and moderate patients; however, no difference was observed between the two patient groups. Expansion of ASCs during viral infections has been shown to be a good predictor of the development of neutralising antibodies and B cell memory. Additionally, during viral infections, ASCs can produce large amounts of antibodies as long as viral shedding occurs, suggesting that ASCs play an active role in infection clearance. We found that the majority of patients had neutralizing antibody titres during the acute phase, perhaps originating from the expanded ASC population. Taken together, the observed ASC expansion during acute COVID-19 may play an important role in SARS-CoV-2 clearance and disease control. We highlight the strength of analyzing secretion of multiple cytokines at once, as this approach provides information on cytokine co-expression patterns and identification of polyfunctional T cells, which are superior in their cytokine secretion capacity and therefore may be important in antiviral defence upon re-exposure to SARS-CoV-2.
Innate lymphoid cells were largely depleted from the circulation of COVID-19 patients compared with healthy controls. Remaining circulating ILCs revealed decreased frequencies of ILC2 in severe COVID-19, with a concomitant decrease of ILC precursors (ILCp) in all patients, compared with controls. ILC2 and ILCp showed an activated phenotype with increased CD69 expression, whereas expression levels of the chemokine receptors CXCR4 and CCR4 were significantly altered in ILC2 and ILCp, and ILC1, respectively. The activated ILC profile of COVID-19 patients was associated with soluble inflammatory markers, while frequencies of ILC subsets were correlated with laboratory parameters that reflect the disease severity.
Unsupervised analysis of peripheral blood NK cells furthermore identified distinct NK cell immunotypes that were linked to disease severity. Hallmarks of these immunotypes were high expression of perforin, NKG2C, and Ksp37, reflecting increased presence of adaptive NK cells in circulation of patients with severe disease. Last, arming of CD56 NK cells was observed across COVID-19 disease states, driven by a defined protein-protein interaction network of inflammatory.
We found that T cell activation, characterized by expression of CD38, was a hallmark of acute COVID-19. Many of these T cells also expressed HLA-DR, Ki-67, and PD-1, indicating a combined activation/cycling phenotype, which correlated with early SARS-CoV-2-specific IgG levels and, to a lesser extent, plasma levels of various inflammatory markers. Our data also showed that many activated/cycling T cells in the acute phase were functionally replete and specific for SARS-CoV-2.
The present findings provided new knowledge regarding molecular mechanisms responsible for COVID-19 as well as host-virus interactions. The current study emphasized the ability of SARS-CoV-2 to cause systemic perturbation in host biological-immunologic gene networks and then manipulate them to accelerate viral replication and progression of COVID-19. Building on these findings, further research is needed to more fully characterize molecular fingerprints underlying SARS-CoV-2 infection for development of drugs and vaccines against COVID-19.
Conclusion
COVID-19 is a global pandemic that has affected many and despite much effort, no effective treatment is yet available. A systems biology approach was used to investigate molecular regulatory mechanisms responsible for COVID-19 at the systemic level. It is noteworthy that both WGCNA methods showed a similar ability to identify candidate modules during COVID-19, confirming each other results. Differential co-expression network analysis revealed that the topological structure of 72% (15 of 21) of the modules were affected due to the SARS-CoV-2 infection. Moreover, based on functional enrichment analysis, among the 15 non-preserved modules between healthy controls and COVID-19 patients, 9 modules were directly involved in the host immune response and COVID-19 immunopathogenesis. Integration of co-expression networks based on the hub genes with PPI networks identified 290 hub-high traffic genes. Indeed, these genes have the highest degree of connection in co-expression networks and the highest BC score in co-expressed hub gene-based PPI networks and can be considered promising therapeutic targets for development of treatment strategies against COVID-19. Interestingly, most of these hub-high traffic genes had a central role in immunopathogenesis of COVID-19 and were directly related to disease severity and mortality. Future research is needed to validate hub-high traffic genes reported in this study, especially those whose role in the pathogenesis of SARS-CoV-2 is yet unclear.
Data Availability Statement
RNA-seq raw reads of COVID-19 patients and healthy individuals were obtained from the Gene Expression Omnibus (GEO) database at the National Center for Biotechnology Information (NCBI) under the accession number of GSE152418.
Author Contributions
AH and AB conceived the ideas. AH and AB designed the study. AH, AB, and NS analyzed the data. AH, AB, BA, BH, and FF interpreted the data. AH wrote the main manuscript. HG, GJ, BH, BA, and MR helped to write manuscript. AB, JK, and HB reviewed and edited the manuscript. All authors contributed to the article and approved the submitted version.
Conflict of Interest
The authors declare that the research was conducted in the absence of any commercial or financial relationships that could be construed as a potential conflict of interest.
Publisher’s Note
All claims expressed in this article are solely those of the authors and do not necessarily represent those of their affiliated organizations, or those of the publisher, the editors and the reviewers. Any product that may be evaluated in this article, or claim that may be made by its manufacturer, is not guaranteed or endorsed by the publisher.
Acknowledgments
The authors thank workers of the branch of systems biology for the assistance in data sets collection. The authors thank all the teams who worked on the contributions and who provided technical assistance during this study. We also thank the editor and reviewers whose critical comments improved the manuscript.
Supplementary Material
The Supplementary Material for this article can be found online at: https://www.frontiersin.org/articles/10.3389/fimmu.2021.789317/full#supplementary-material
Abbreviations
AKI, acute kidney injury; ALI, acute lung injury; ARDS, acute respiratory distress syndrome; BC, betweenness centrality; COVID-19, corona virus disease 2019; CVD, cardiovascular disease; CPM, count per million; GO, gene ontology; KEGG, Kyoto encyclopedia of genes and genomes; MERS-CoV, middle east respiratory syndrome coronavirus; MM, module membership; MOF, multi organ failure; PBMC, peripheral blood mononuclear cell; PPI, protein-protein interaction; RA, rheumatoid arthritis; RNA-seq, RNA-sequencing; SARS-CoV-2, severe acute respiratory syndrome corona virus 2; TF, transcription factor; TOM, topological overlap matrix; WGCNA, weighted gene co-expression network analysis.
References
1. Guan W-J, Ni Z-Y, Hu Y, Liang W-H, Ou C-Q, He J-X, et al. Clinical Characteristics of Coronavirus Disease 2019 in China. N Engl J Med (2020) 382(18):1708–20. doi: 10.1056/NEJMoa2002032
2. Cucinotta D, Vanelli M. WHO Declares COVID-19 a Pandemic. Acta BioMed (2020) 91(1):157–60. doi: 10.23750/abm.v91i1.9397
3. Sohrabi C, Alsafi Z, O'Neill N, Khan M, Kerwan A, Al-Jabir A, et al. World Health Organization Declares Global Emergency: A Review of the 2019 Novel Coronavirus (COVID-19). Int J Surg (2020) 76:71–6. doi: 10.1016/j.ijsu.2020.02.034
4. Auwul MR, Rahman MR, Gov E, Shahjaman M, Moni MA. Bioinformatics and Machine Learning Approach Identifies Potential Drug Targets and Pathways in COVID-19. Briefings Bioinf (2021). doi: 10.1093/bib/bbab120
5. Chen N, Zhou M, Dong X, Qu J, Gong F, Han Y, et al. Epidemiological and Clinical Characteristics of 99 Cases of 2019 Novel Coronavirus Pneumonia in Wuhan, China: A Descriptive Study. Lancet (2020) 395(10223):507–13. doi: 10.1016/S0140-6736(20)30211-7
6. Arunachalam PS, Wimmers F, Mok CKP, Perera RAPM, Scott M, Hagan T, et al. Systems Biological Assessment of Immunity to Mild Versus Severe COVID-19 Infection in Humans. Science (2020) 369(6508):1210–20. doi: 10.1126/science.abc6261
7. Schulte-Schrepping J, Reusch N, Paclik D, Baßler K, Schlickeiser S, Zhang B, et al. Severe COVID-19 Is Marked by a Dysregulated Myeloid Cell Compartment. Cell (2020) 182(6):1419–40. doi: 10.1038/s41598-021-86002-x
8. Daamen AR, Bachali P, Owen KA, Kingsmore KM, Hubbard EL, Labonte AC, et al. Comprehensive transcriptomic analysis of COVID-19 blood, lung, and airway. Sci Rep (2021) 11(1):7052. doi: 10.1038/s41598-021-86002-x
9. Jain R, Ramaswamy S, Harilal D, Uddin M, Loney T, Nowotny N, et al. Host Transcriptomic Profiling of COVID-19 Patients With Mild, Moderate, and Severe Clinical Outcomes. Comput Struct Biotechnol J (2021) 19:153–60. doi: 10.1016/j.csbj.2020.12.016
10. Islam T, Rahman MR, Aydin B, Beklen H, Arga KY, Shahjaman M. Integrative Transcriptomics Analysis of Lung Epithelial Cells and Identification of Repurposable Drug Candidates for COVID-19. Eur J Pharmacol (2020) 887:173594. doi: 10.1016/j.ejphar.2020.173594
11. Oommen A, Cunningham S, Joshi L. Transcriptomic Analysis of Respiratory Tissue and Cell Line Models to Examine Glycosylation Machinery During SARS-CoV-2 Infection. Viruses (2021) 13(1):82. doi: 10.3390/v13010082
12. Blanco-Melo D, Nilsson-Payant BE, Liu W-C, Møller R, Panis M, Sachs D, et al. SARS-CoV-2 Launches a Unique Transcriptional Signature From In Vitro, Ex Vivo, and In Vivo Systems. bioRxiv (2020) 2020.03.24.004655. doi: 10.1101/2020.03.24.004655
13. Fagone P, Ciurleo R, Lombardo SD, Iacobello C, Palermo CI, Shoenfeld Y, et al. Transcriptional Landscape of SARS-CoV-2 Infection Dismantles Pathogenic Pathways Activated by the Virus, Proposes Unique Sex-Specific Differences and Predicts Tailored Therapeutic Strategies. Autoimmun Rev (2020) 19(7):102571. doi: 10.1016/j.autrev.2020.102571
14. Yang S, Wu S, Yu Z, Huang J, Zhong X, Liu X, et al. Transcriptomic Analysis Reveals Novel Mechanisms of SARS-CoV-2 Infection in Human Lung Cells. Immun Inflamm Dis (2020) 8(4):753–62. doi: 10.1002/iid3.366
15. Ong EZ, Chan YFZ, Leong WY, Lee NMY, Kalimuddin S, Haja Mohideen SM, et al. A Dynamic Immune Response Shapes COVID-19 Progression. Cell Host Microbe (2020) 27(6):879–82.e2. doi: 10.1016/j.chom.2020.03.021
16. Bakhtiarizadeh MR, Mirzaei S, Norouzi M, Sheybani N, Vafaei Sadi MS. Identification of Gene Modules and Hub Genes Involved in Mastitis Development Using a Systems Biology Approach. Front Genet (2020) 11:722(722). doi: 10.3389/fgene.2020.00722
17. Liu L, Amorín R, Moriel P, DiLorenzo N, Lancaster PA, Peñagaricano F. Differential Network Analysis of Bovine Muscle Reveals Changes in Gene Coexpression Patterns in Response to Changes in Maternal Nutrition. BMC Genomics (2020) 21(1):684. doi: 10.1186/s12864-020-07068-x
18. Joshi A, Rienks M, Theofilatos K, Mayr M. Systems Biology in Cardiovascular Disease: A Multiomics Approach. Nat Rev Cardiol (2021) 18(5):313–30. doi: 10.1038/s41569-020-00477-1
19. Langfelder P, Horvath S. WGCNA: An R Package for Weighted Correlation Network Analysis. BMC Bioinf (2008) 9(1):559. doi: 10.1186/1471-2105-9-559
20. Guo J, Yu H, Yang J, Li Y, Zhang X, Mei K, et al. Weighted Gene Co-Expression Network Analysis Revealed Host Transcriptional Response to H1N1 Influenza A Virus Infection. J Infect (2021) 82(3):e4–7. doi: 10.1016/j.jinf.2020.12.017
21. Xu S, Yuan H, Li L, Bai F, Yang K, Zhao L. Identification Potential Epigenetic Biomarkers of a Human Immunodeficiency Virus/Tuberculosis Co-Infection Based on Weighted Gene Co-Expression Network Analysis. Microbiol Immunol (2021) 65:422–31. doi: 10.1111/1348-0421.12926
22. Jha M, Malhotra AG, Singh S, Pandey KM. Gene Co-Expression Network Analysis Reveals Common System-Level Properties of Genes Involved in Tuberculosis Across Independent Gene Expression Studies. Network Model Anal Health Inf Bioinf (2016) 5(1):24. doi: 10.1007/s13721-016-0131-8
23. Liu C, Dai Q, Ding Q, Wei M, Kong X. Identification of Key Genes in Hepatitis B Associated Hepatocellular Carcinoma Based on WGCNA. Infect Agents Cancer (2021) 16(1):18. doi: 10.1186/s13027-021-00357-4
24. Lin H, Zhang Q, Li X, Wu Y, Liu Y, Hu Y. Identification of Key Candidate Genes and Pathways in Hepatitis B Virus-Associated Acute Liver Failure by Bioinformatical Analysis. Medicine (2018) 97(5):e9687. doi: 10.1097/md.0000000000009687
25. Cheng Y, Ping J, Chen J. Identification of Potential Gene Network Associated With HCV-Related Hepatocellular Carcinoma Using Microarray Analysis. Pathol Oncol Res (2018) 24(3):507–14. doi: 10.1007/s12253-017-0273-8
26. Sehl ME, Rickabaugh TM, Shih R, Martinez-Maza O, Horvath S, Ramirez CM, et al. The Effects of Anti-Retroviral Therapy on Epigenetic Age Acceleration Observed in HIV-1-Infected Adults. Pathog Immun (2020) 5(1):291–311. doi: 10.20411/pai.v5i1.376
27. Tian Z, He W, Tang J, Liao X, Yang Q, Wu Y, et al. Identification of Important Modules and Biomarkers in Breast Cancer Based on WGCNA. Onco Targets Ther (2020) 13:6805–17. doi: 10.2147/ott.S258439
28. Di Y, Chen D, Yu W, Yan L. Bladder Cancer Stage-Associated Hub Genes Revealed by WGCNA Co-Expression Network Analysis. Hereditas (2019) 156(1):7. doi: 10.1186/s41065-019-0083-y
29. Ding M, Li F, Wang B, Chi G, Liu H. A Comprehensive Analysis of WGCNA and Serum Metabolomics Manifests the Lung Cancer-Associated Disordered Glucose Metabolism. J Cell Biochem (2019) 120(6):10855–63. doi: 10.1002/jcb.28377
30. Zhai X, Xue Q, Liu Q, Guo Y, Chen Z. Colon Cancer Recurrence−Associated Genes Revealed by WGCNA Co−Expression Network Analysis. Mol Med Rep (2017) 16(5):6499–505. doi: 10.3892/mmr.2017.7412
31. Overmyer KA, Shishkova E, Miller IJ, Balnis J, Bernstein MN, Peters-Clarke TM, et al. Large-Scale Multi-Omic Analysis of COVID-19 Severity. Cell Syst (2021) 12(1):23–40. doi: 10.1016/j.cels.2020.10.003
32. Zhang J, Lin D, Li K, Ding X, Li L, Liu Y, et al. Transcriptome Analysis of Peripheral Blood Mononuclear Cells Reveals Distinct Immune Response in Asymptomatic and Re-Detectable Positive COVID-19 Patients. Front Immunol (2021) 12:716075(3109). doi: 10.3389/fimmu.2021.716075
33. Zheng H-Y, Xu M, Yang C-X, Tian R-R, Zhang M, Li J-J, et al. Longitudinal Transcriptome Analyses Show Robust T Cell Immunity During Recovery From COVID-19. Signal Transduct Targeted Ther (2020) 5(1):294. doi: 10.1038/s41392-020-00457-4
34. Langfelder P, Luo R, Oldham MC, Horvath S. Is My Network Module Preserved and Reproducible? PloS Comput Biol (2011) 7(1):e1001057. doi: 10.1371/journal.pcbi.1001057
35. Hasankhani A, Bahrami A, Sheybani N, Fatehi F, Abadeh R, Ghaem Maghami Farahani H, et al. Integrated Network Analysis to Identify Key Modules and Potential Hub Genes Involved in Bovine Respiratory Disease: A Systems Biology Approach. Front Genet (2021) 12:753839. doi: 10.1371/journal.pone.0156006
36. Riquelme Medina I, Lubovac-Pilav Z. Gene co-expression network analysis for identifying modules and functionally enriched pathways in type 1 diabetes. PloS One (2016) 11(6):e0156006. doi: 10.1371/journal.pone.0156006
37. Mukund K, Subramaniam S. Dysregulated Mechanisms Underlying Duchenne Muscular Dystrophy From Co-Expression Network Preservation Analysis. BMC Res Notes (2015) 8(1):182. doi: 10.1186/s13104-015-1141-9
38. Heidari M, Pakdel A, Bakhtiarizadeh MR, Dehghanian F. Integrated Analysis of lncRNAs, mRNAs, and TFs to Identify Regulatory Networks Underlying MAP Infection in Cattle. Front Genet (2021) 12:668448(1122). doi: 10.3389/fgene.2021.668448
39. van Dam S, Võsa U, van der Graaf A, Franke L, de Magalhães JP. Gene Co-Expression Analysis for Functional Classification and Gene–Disease Predictions. Briefings Bioinf (2017) 19(4):575–92. doi: 10.1093/bib/bbw139
40. Bolger AM, Lohse M, Usadel B. Trimmomatic: A Flexible Trimmer for Illumina Sequence Data. Bioinformatics (2014) 30(15):2114–20. doi: 10.1093/bioinformatics/btu170
41. Kim D, Paggi JM, Park C, Bennett C, Salzberg SL. Graph-Based Genome Alignment and Genotyping With HISAT2 and HISAT-Genotype. Nat Biotechnol (2019) 37(8):907–15. doi: 10.1038/s41587-019-0201-4
42. Anders S, Pyl PT, Huber W. HTSeq—a Python Framework to Work With High-Throughput Sequencing Data. Bioinformatics (2014) 31(2):166–9. doi: 10.1093/bioinformatics/btu638
43. Law CW, Chen Y, Shi W, Smyth GK. Voom: Precision Weights Unlock Linear Model Analysis Tools for RNA-Seq Read Counts. Genome Biol (2014) 15(2):R29. doi: 10.1186/gb-2014-15-2-r29
44. Smyth GK. Limma: Linear Models for Microarray Data. In: Gentleman R, Carey VJ, Huber W, Irizarry RA, Dudoit S, editors. Bioinformatics and Computational Biology Solutions Using R and Bioconductor. New York, NY: Springer New York (2005). p. 397–420.
45. Mason MJ, Fan G, Plath K, Zhou Q, Horvath S. Signed Weighted Gene Co-Expression Network Analysis of Transcriptional Regulation in Murine Embryonic Stem Cells. BMC Genomics (2009) 10(1):327. doi: 10.1186/1471-2164-10-327
46. Langfelder P, Horvath S. Fast R Functions for Robust Correlations and Hierarchical Clustering. J Stat Software (2012) 46(11):17. doi: 10.18637/jss.v046.i11
47. Song L, Langfelder P, Horvath S. Comparison of Co-Expression Measures: Mutual Information, Correlation, and Model Based Indices. BMC Bioinf (2012) 13(1):328. doi: 10.1186/1471-2105-13-328
48. Chen EY, Tan CM, Kou Y, Duan Q, Wang Z, Meirelles GV, et al. Enrichr: Interactive and Collaborative HTML5 Gene List Enrichment Analysis Tool. BMC Bioinf (2013) 14(1):128. doi: 10.1186/1471-2105-14-128
49. Cui H, Shan H, Miao MZ, Jiang Z, Meng Y, Chen R, et al. Identification of the Key Genes and Pathways Involved in the Tumorigenesis and Prognosis of Kidney Renal Clear Cell Carcinoma. Sci Rep (2020) 10(1):4271. doi: 10.1038/s41598-020-61162-4
50. Liu Y, Gu H-Y, Zhu J, Niu Y-M, Zhang C, Guo G-L. Identification of Hub Genes and Key Pathways Associated With Bipolar Disorder Based on Weighted Gene Co-Expression Network Analysis. Front Physiol (2019) 10:1081(1081). doi: 10.3389/fphys.2019.01081
51. Yao Q, Song Z, Wang B, Qin Q, Zhang J-A. Identifying Key Genes and Functionally Enriched Pathways in Sjögren’s Syndrome by Weighted Gene Co-Expression Network Analysis. Front Genet (2019) 10:1142(1142). doi: 10.3389/fgene.2019.01142
52. Goh K-I, Cusick ME, Valle D, Childs B, Vidal M, Barabási A-L. The Human Disease Network. Proc Natl Acad Sci (2007) 104(21):8685–90. doi: 10.1073/pnas.0701361104
53. Li J, Zhou D, Qiu W, Shi Y, Yang J-J, Chen S, et al. Application of Weighted Gene Co-Expression Network Analysis for Data From Paired Design. Sci Rep (2018) 8(1):622. doi: 10.1038/s41598-017-18705-z
54. Zhou Y, Xu J, Liu Y, Li J, Chang C, Xu C. Rat Hepatocytes Weighted Gene Co-Expression Network Analysis Identifies Specific Modules and Hub Genes Related to Liver Regeneration After Partial Hepatectomy. PloS One (2014) 9(4):e94868. doi: 10.1371/journal.pone.0094868
55. Szklarczyk D, Gable AL, Lyon D, Junge A, Wyder S, Huerta-Cepas J, et al. STRING V11: Protein–Protein Association Networks With Increased Coverage, Supporting Functional Discovery in Genome-Wide Experimental Datasets. Nucleic Acids Res (2018) 47(D1):D607–13. doi: 10.1093/nar/gky1131
56. Hu H, Miao Y-R, Jia L-H, Yu Q-Y, Zhang Q, Guo A-Y. AnimalTFDB 3.0: A Comprehensive Resource for Annotation and Prediction of Animal Transcription Factors. Nucleic Acids Res (2018) 47(D1):D33–8. doi: 10.1093/nar/gky822
57. Jiminez J, Timsit E, Orsel K, van der Meer F, Guan LL, Plastow G. Whole-Blood Transcriptome Analysis of Feedlot Cattle With and Without Bovine Respiratory Disease. Front Genet (2021) 12:627623(257). doi: 10.3389/fgene.2021.627623
58. Yang J-L, Wang CCN, Cai J-H, Chou C-Y, Lin Y-C, Hung C-C. Identification of GSN and LAMC2 as Key Prognostic Genes of Bladder Cancer by Integrated Bioinformatics Analysis. Cancers (2020) 12(7):1809. doi: 10.3390/cancers12071809
59. McDermott JE, Diamond DL, Corley C, Rasmussen AL, Katze MG, Waters KM. Topological Analysis of Protein Co-Abundance Networks Identifies Novel Host Targets Important for HCV Infection and Pathogenesis. BMC Syst Biol (2012) 6(1):28. doi: 10.1186/1752-0509-6-28
60. Duran-Pinedo AE, Paster B, Teles R, Frias-Lopez J. Correlation Network Analysis Applied to Complex Biofilm Communities. PloS One (2011) 6(12):e28438. doi: 10.1371/journal.pone.0028438
61. Barrat A, Barthélemy M, Pastor-Satorras R, Vespignani A. The Architecture of Complex Weighted Networks. Proc Natl Acad Sci USA (2004) 101(11):3747. doi: 10.1073/pnas.0400087101
62. Wang CCN, Li CY, Cai J-H, Sheu PCY, Tsai JJP, Wu M-Y, et al. Identification of Prognostic Candidate Genes in Breast Cancer by Integrated Bioinformatic Analysis. J Clin Med (2019) 8(8):1160. doi: 10.3390/jcm8081160
63. Chin C-H, Chen S-H, Wu H-H, Ho C-W, Ko M-T, Lin C-Y. Cytohubba: Identifying Hub Objects and Sub-Networks From Complex Interactome. BMC Syst Biol (2014) 8(4):S11. doi: 10.1186/1752-0509-8-S4-S11
64. Saito R, Smoot ME, Ono K, Ruscheinski J, Wang P-L, Lotia S, et al. A Travel Guide to Cytoscape Plugins. Nat Methods (2012) 9(11):1069–76. doi: 10.1038/nmeth.2212
65. Lee C, Choi WJ. Overview of COVID-19 Inflammatory Pathogenesis From the Therapeutic Perspective. Arch Pharmacal Res (2021) 44(1):99–116. doi: 10.1007/s12272-020-01301-7
66. Mathew D, Giles JR, Baxter AE, Oldridge DA, Greenplate AR, Wu JE, et al. Deep Immune Profiling of COVID-19 Patients Reveals Distinct Immunotypes With Therapeutic Implications. Science (2020) 369(6508):eabc8511. doi: 10.1126/science.abc8511
67. Torequl Islam M, Nasiruddin M, Khan IN, Mishra SK, Kudrat-E-Zahan M, Alam Riaz T, et al. A Perspective on Emerging Therapeutic Interventions for COVID-19. Front Public Health (2020) 8:281(281). doi: 10.3389/fpubh.2020.00281
68. Wu R, Wang L, Kuo H-CD, Shannar A, Peter R, Chou PJ, et al. An Update on Current Therapeutic Drugs Treating COVID-19. Curr Pharmacol Rep (2020) 6(3):56–70. doi: 10.1007/s40495-020-00216-7
69. Lotfi M, Hamblin MR, Rezaei N. COVID-19: Transmission, Prevention, and Potential Therapeutic Opportunities. Clinica Chimica Acta (2020) 508:254–66. doi: 10.1016/j.cca.2020.05.044
70. Zhang J, Xie B, Hashimoto K. Current Status of Potential Therapeutic Candidates for the COVID-19 Crisis. Brain Behavior Immun (2020) 87:59–73. doi: 10.1016/j.bbi.2020.04.046
71. Fitzgerald KA, Kagan JC. Toll-Like Receptors and the Control of Immunity. Cell (2020) 180(6):1044–66. doi: 10.1016/j.cell.2020.02.041
72. Debnath M, Banerjee M, Berk M. Genetic Gateways to COVID-19 Infection: Implications for Risk, Severity, and Outcomes. FASEB J (2020) 34(7):8787–95. doi: 10.1096/fj.202001115R
73. Khanmohammadi S, Rezaei N. Role of Toll-Like Receptors in the Pathogenesis of COVID-19. J Med Virol (2021) 93(5):2735–9. doi: 10.1002/jmv.26826
74. Patra R, Chandra Das N, Mukherjee S. Targeting Human TLRs to Combat COVID-19: A Solution? J Med Virol (2021) 93(2):615–7. doi: 10.1002/jmv.26387
75. Brandão SCS, Ramos JDOX, Dompieri LT, Godoi ETAM, Figueiredo JL, Sarinho ESC, et al. Is Toll-Like Receptor 4 Involved in the Severity of COVID-19 Pathology in Patients With Cardiometabolic Comorbidities? Cytokine Growth Factor Rev (2021) 58:102–10. doi: 10.1016/j.cytogfr.2020.09.002
76. Conti P, Ronconi G, Caraffa A, Gallenga CE, Ross R, Frydas I, et al. Induction of Pro-Inflammatory Cytokines (IL-1 and IL-6) and Lung Inflammation by Coronavirus-19 (COVI-19 or SARS-CoV-2): Anti-Inflammatory Strategies. J Biol Regul Homeost Agents (2020) 34(2):327–31. doi: 10.23812/conti-e
77. Mukherjee S, Huda S, Sinha Babu SP. Toll-Like Receptor Polymorphism in Host Immune Response to Infectious Diseases: A Review. Scand J Immunol (2019) 90(1):e12771. doi: 10.1111/sji.12771
78. Lu Q, Liu J, Zhao S, Gomez Castro MF, Laurent-Rolle M, Dong J, et al. SARS-CoV-2 Exacerbates Proinflammatory Responses in Myeloid Cells Through C-Type Lectin Receptors and Tweety Family Member 2. Immunity (2021) 54(6):1304–19.e9. doi: 10.1016/j.immuni.2021.05.006
79. Zhao X, Chu H, Wong BH-Y, Chiu MC, Wang D, Li C, et al. Activation of C-Type Lectin Receptor and (RIG)-I-Like Receptors Contributes to Proinflammatory Response in Middle East Respiratory Syndrome Coronavirus-Infected Macrophages. J Infect Dis (2019) 221(4):647–59. doi: 10.1093/infdis/jiz483
80. Lin X, Fu B, Yin S, Li Z, Liu H, Zhang H, et al. ORF8 Contributes to Cytokine Storm During SARS-CoV-2 Infection by Activating IL-17 Pathway. iScience (2021) 24(4):102293. doi: 10.1016/j.isci.2021.102293
81. Tay MZ, Poh CM, Rénia L, MacAry PA, Ng LFP. The Trinity of COVID-19: Immunity, Inflammation and Intervention. Nat Rev Immunol (2020) 20(6):363–74. doi: 10.1038/s41577-020-0311-8
82. McGonagle D, Sharif K, O'Regan A, Bridgewood C. The Role of Cytokines Including Interleukin-6 in COVID-19 Induced Pneumonia and Macrophage Activation Syndrome-Like Disease. Autoimmun Rev (2020) 19(6):102537. doi: 10.1016/j.autrev.2020.102537
83. Hariharan A, Hakeem AR, Radhakrishnan S, Reddy MS, Rela M. The Role and Therapeutic Potential of NF-Kappa-B Pathway in Severe COVID-19 Patients. Inflammopharmacology (2021) 29(1):91–100. doi: 10.1007/s10787-020-00773-9
84. Sun X, Wang T, Cai D, Hu Z, Chen JA, Liao H, et al. Cytokine Storm Intervention in the Early Stages of COVID-19 Pneumonia. Cytokine Growth Factor Rev (2020) 53:38–42. doi: 10.1016/j.cytogfr.2020.04.002
85. Kircheis R, Haasbach E, Lueftenegger D, Heyken WT, Ocker M, Planz O. NF-κb Pathway as a Potential Target for Treatment of Critical Stage COVID-19 Patients. Front Immunol (2020) 11:598444(3446). doi: 10.3389/fimmu.2020.598444
86. Rehan M, Ahmed F, Howladar SM, Refai MY, Baeissa HM, Zughaibi TA, et al. A Computational Approach Identified Andrographolide as a Potential Drug for Suppressing COVID-19-Induced Cytokine Storm. Front Immunol (2021) 12:648250(2343). doi: 10.3389/fimmu.2021.648250
87. Ahmed F. A Network-Based Analysis Reveals the Mechanism Underlying Vitamin D in Suppressing Cytokine Storm and Virus in SARS-CoV-2 Infection. Front Immunol (2020) 11:590459(3084). doi: 10.3389/fimmu.2020.590459
88. Patel BV, Wilson MR, O’Dea KP, Takata M. TNF-Induced Death Signaling Triggers Alveolar Epithelial Dysfunction in Acute Lung Injury. J Immunol (2013) 190(8):4274–82. doi: 10.4049/jimmunol.1202437
89. He B, Garmire L. Prediction of Repurposed Drugs for Treating Lung Injury in COVID-19. F1000Research (2020) 9:609. doi: 10.12688/f1000research.23996.2
90. Taghiloo S, Aliyali M, Abedi S, Mehravaran H, Sharifpour A, Zaboli E, et al. Apoptosis and Immunophenotyping of Peripheral Blood Lymphocytes in Iranian COVID-19 Patients: Clinical and Laboratory Characteristics. J Med Virol (2021) 93(3):1589–98. doi: 10.1002/jmv.26505
91. Chu H, Zhou J, Wong BH-Y, Li C, Chan JF-W, Cheng Z-S, et al. Middle East Respiratory Syndrome Coronavirus Efficiently Infects Human Primary T Lymphocytes and Activates the Extrinsic and Intrinsic Apoptosis Pathways. J Infect Dis (2016) 213(6):904–14. doi: 10.1093/infdis/jiv380
92. Haljasmägi L, Salumets A, Rumm AP, Jürgenson M, Krassohhina E, Remm A, et al. Longitudinal Proteomic Profiling Reveals Increased Early Inflammation and Sustained Apoptosis Proteins in Severe COVID-19. Sci Rep (2020) 10(1):20533. doi: 10.1038/s41598-020-77525-w
93. Huang C, Wang Y, Li X, Ren L, Zhao J, Hu Y, et al. Clinical Features of Patients Infected With 2019 Novel Coronavirus in Wuhan, China. Lancet (2020) 395(10223):497–506. doi: 10.1016/S0140-6736(20)30183-5
94. Cizmecioglu A, Akay Cizmecioglu H, Goktepe MH, Emsen A, Korkmaz C, Esenkaya Tasbent F, et al. Apoptosis-Induced T-Cell Lymphopenia is Related to COVID-19 Severity. J Med Virol (2021) 93(5):2867–74. doi: 10.1002/jmv.26742
95. Thompson EA, Cascino K, Ordonez AA, Zhou W, Vaghasia A, Hamacher-Brady A, et al. Metabolic Programs Define Dysfunctional Immune Responses in Severe COVID-19 Patients. Cell Rep (2021) 34(11):108863. doi: 10.1016/j.celrep.2021.108863
96. Diao B, Wang C, Tan Y, Chen X, Liu Y, Ning L, et al. Reduction and Functional Exhaustion of T Cells in Patients With Coronavirus Disease 2019 (COVID-19). Front Immunol (2020) 11:827(827). doi: 10.3389/fimmu.2020.00827
97. Xiong Y, Liu Y, Cao L, Wang D, Guo M, Jiang A, et al. Transcriptomic Characteristics of Bronchoalveolar Lavage Fluid and Peripheral Blood Mononuclear Cells in COVID-19 Patients. Emerging Microbes Infect (2020) 9(1):761–70. doi: 10.1080/22221751.2020.1747363
98. Courties A, Boussier J, Hadjadj J, Yatim N, Barnabei L, Péré H, et al. Regulation of the Acetylcholine/α7nachr Anti-Inflammatory Pathway in COVID-19 Patients. Sci Rep (2021) 11(1):11886. doi: 10.1038/s41598-021-91417-7
99. Ragab D, Salah Eldin H, Taeimah M, Khattab R, Salem R. The COVID-19 Cytokine Storm; What We Know So Far. Front Immunol (2020) 11:1446(1446). doi: 10.3389/fimmu.2020.01446
100. Merad M, Martin JC. Pathological Inflammation in Patients With COVID-19: A Key Role for Monocytes and Macrophages. Nat Rev Immunol (2020) 20(6):355–62. doi: 10.1038/s41577-020-0331-4
101. Cao X. COVID-19: Immunopathology and its Implications for Therapy. Nat Rev Immunol (2020) 20(5):269–70. doi: 10.1038/s41577-020-0308-3
102. Qin C, Zhou L, Hu Z, Zhang S, Yang S, Tao Y, et al. Dysregulation of Immune Response in Patients With Coronavirus 2019 (COVID-19) in Wuhan, China. Clin Infect Dis (2020) 71(15):762–8. doi: 10.1093/cid/ciaa248
103. Gan J, Li J, Li S, Yang C. Leucocyte Subsets Effectively Predict the Clinical Outcome of Patients With COVID-19 Pneumonia: A Retrospective Case-Control Study. Front Public Health (2020) 8:299(299). doi: 10.3389/fpubh.2020.00299
104. Trecarichi EM, Mazzitelli M, Serapide F, Pelle MC, Tassone B, Arrighi E, et al. Clinical Characteristics and Predictors of Mortality Associated With COVID-19 in Elderly Patients From a Long-Term Care Facility. Sci Rep (2020) 10(1):20834. doi: 10.1038/s41598-020-77641-7
105. de Bruin S, Bos LD, van Roon MA, Tuip-de Boer AM, Schuurman AR, Koel-Simmelinck MJA, et al. Clinical Features and Prognostic Factors in Covid-19: A Prospective Cohort Study. EBioMedicine (2021) 67:103378. doi: 10.1016/j.ebiom.2021.103378
106. Silberstein M. Correlation Between Premorbid IL-6 Levels and COVID-19 Mortality: Potential Role for Vitamin D. Int Immunopharmacol (2020) 88:106995. doi: 10.1016/j.intimp.2020.106995
107. Pandolfi L, Fossali T, Frangipane V, Bozzini S, Morosini M, D’Amato M, et al. Broncho-Alveolar Inflammation in COVID-19 Patients: A Correlation With Clinical Outcome. BMC Pulm Med (2020) 20(1):301. doi: 10.1186/s12890-020-01343-z
108. Patel H, Ashton NJ, Dobson RJB, Andersson L-M, Yilmaz A, Blennow K, et al. Proteomic Blood Profiling in Mild, Severe and Critical COVID-19 Patients. Sci Rep (2021) 11(1):6357. doi: 10.1038/s41598-021-85877-0
109. Zhang J, Wang Z, Wang X, Hu Z, Yang C, Lei P. Risk Factors for Mortality of COVID-19 Patient Based on Clinical Course: A Single Center Retrospective Case-Control Study. Front Immunol (2021) 12:581469(234). doi: 10.3389/fimmu.2021.581469
110. Castelnovo L, Tamburello A, Lurati A, Zaccara E, Marrazza MG, Olivetti M, et al. Anti-IL6 Treatment of Serious COVID-19 Disease: A Monocentric Retrospective Experience. Medicine (2021) 100(1):e23582. doi: 10.1097/md.0000000000023582
111. Han Q, Guo M, Zheng Y, Zhang Y, De Y, Xu C, et al. Current Evidence of Interleukin-6 Signaling Inhibitors in Patients With COVID-19: A Systematic Review and Meta-Analysis. Front Pharmacol (2020) 11:615972(2119). doi: 10.3389/fphar.2020.615972
112. Wu J, Shen J, Han Y, Qiao Q, Dai W, He B, et al. Upregulated IL-6 Indicates a Poor COVID-19 Prognosis: A Call for Tocilizumab and Convalescent Plasma Treatment. Front Immunol (2021) 12:598799(455). doi: 10.3389/fimmu.2021.598799
113. Flisiak R, Jaroszewicz J, Rogalska M, Łapiński T, Berkan-Kawińska A, Bolewska B, et al. Tocilizumab Improves the Prognosis of COVID-19 in Patients With High IL-6. J Clin Med (2021) 10(8):1583. doi: 10.3390/jcm10081583
114. Saghazadeh A, Rezaei N. Towards Treatment Planning of COVID-19: Rationale and Hypothesis for the Use of Multiple Immunosuppressive Agents: Anti-Antibodies, Immunoglobulins, and Corticosteroids. Int Immunopharmacol (2020) 84:106560. doi: 10.1016/j.intimp.2020.106560
115. Rossotti R, Travi G, Ughi N, Corradin M, Baiguera C, Fumagalli R, et al. Safety and Efficacy of Anti-Il6-Receptor Tocilizumab Use in Severe and Critical Patients Affected by Coronavirus Disease 2019: A Comparative Analysis. J Infect (2020) 81(4):e11–7. doi: 10.1016/j.jinf.2020.07.008
116. Del Valle DM, Kim-Schulze S, Huang H-H, Beckmann ND, Nirenberg S, Wang B, et al. An Inflammatory Cytokine Signature Predicts COVID-19 Severity and Survival. Nat Med (2020) 26(10):1636–43. doi: 10.1038/s41591-020-1051-9
117. Robinson PC, Liew DFL, Liew JW, Monaco C, Richards D, Shivakumar S, et al. The Potential for Repurposing Anti-TNF as a Therapy for the Treatment of COVID-19. Med (2020) 1(1):90–102. doi: 10.1016/j.medj.2020.11.005
118. Kim HW, Lee C-K, Cha H-S, Choe J-Y, Park E-J, Kim J. Effect of Anti-Tumor Necrosis Factor Alpha Treatment of Rheumatoid Arthritis and Chronic Kidney Disease. Rheumatol Int (2015) 35(4):727–34. doi: 10.1007/s00296-014-3146-4
119. Ulfgren A-K, Andersson U, Engström M, Klareskog L, Maini RN, Taylor PC. Systemic Anti–Tumor Necrosis Factor α Therapy in Rheumatoid Arthritis Down-Regulates Synovial Tumor Necrosis Factor α Synthesis. Arthritis Rheumatism (2000) 43(11):2391–6. doi: 10.1002/1529-0131(200011)43:11<2391::AID-ANR3>3.0.CO;2-F
120. Van Deventer SJ. Tumour Necrosis Factor and Crohn's Disease. Gut (1997) 40(4):443–8. doi: 10.1136/gut.40.4.443
121. Goedkoop AY, Kraan MC, Picavet DI, de Rie MA, Teunissen MBM, Bos JD, et al. Deactivation of Endothelium and Reduction in Angiogenesis in Psoriatic Skin and Synovium by Low Dose Infliximab Therapy in Combination With Stable Methotrexate Therapy: A Prospective Single-Centre Study. Arthritis Res Ther (2004) 6(4):R326. doi: 10.1186/ar1182
122. Lv S, Han M, Yi R, Kwon S, Dai C, Wang R. Anti-TNF-α Therapy for Patients With Sepsis: A Systematic Meta-Analysis. Int J Clin Pract (2014) 68(4):520–8. doi: 10.1111/ijcp.12382
123. Paleolog EM, Young S, Stark AC, McCloskey RV, Feldmann M, Maini RN. Modulation of Angiogenic Vascular Endothelial Growth Factor by Tumor Necrosis Factor α and Interleukin-1 in Rheumatoid Arthritis. Arthritis Rheumatism (1998) 41(7):1258–65. doi: 10.1002/1529-0131(199807)41:7<1258::AID-ART17>3.0.CO;2-1
124. Taylor PC, Peters AM, Paleolog E, Chapman PT, Elliott MJ, McCloskey R, et al. Reduction of Chemokine Levels and Leukocyte Traffic to Joints by Tumor Necrosis Factor α Blockade in Patients With Rheumatoid Arthritis. Arthritis Rheumatism (2000) 43(1):38–47. doi: 10.1002/1529-0131(200001)43:1<38::AID-ANR6>3.0.CO;2-L
125. Pittoni V, Bombardieri M, Spinelli FR, Scrivo R, Alessandri C, Conti F, et al. Anti-Tumour Necrosis Factor (TNF) α Treatment of Rheumatoid Arthritis (Infliximab) Selectively Down Regulates the Production of Interleukin (IL) 18 But Not of IL12 and IL13. Ann Rheumatic Dis (2002) 61(8):723–5. doi: 10.1136/ard.61.8.723
126. Tak PP, Taylor PC, Breedveld FC, Smeets TJM, Daha MR, Kluin PM, et al. Decrease in Cellularity and Expression of Adhesion Molecules by Anti–Tumor Necrosis Factor α Monoclonal Antibody Treatment in Patients With Rheumatoid Arthritis. Arthritis Rheumatism (1996) 39(7):1077–81. doi: 10.1002/art.1780390702
127. Schotte H, Schl̈ter B, Willeke P, Mickholz E, Schorat MA, Domschke W, et al. Long-Term Treatment With Etanercept Significantly Reduces the Number of Proinflammatory Cytokine-Secreting Peripheral Blood Mononuclear Cells in Patients With Rheumatoid Arthritis. Rheumatology (2004) 43(8):960–4. doi: 10.1093/rheumatology/keh178
128. Feldmann M, Maini RN, Woody JN, Holgate ST, Winter G, Rowland M, et al. Trials of Anti-Tumour Necrosis Factor Therapy for COVID-19 are Urgently Needed. Lancet (2020) 395(10234):1407–9. doi: 10.1016/S0140-6736(20)30858-8
129. Robinson PC, Richards D, Tanner HL, Feldmann M. Accumulating Evidence Suggests Anti-TNF Therapy Needs to be Given Trial Priority in COVID-19 Treatment. Lancet Rheumatol (2020) 2(11):e653–e5. doi: 10.1016/S2665-9913(20)30309-X
130. Liu T, Zhang L, Joo D, Sun S-C. NF-κb Signaling in Inflammation. Signal Transduct Target Ther (2017) 2(1):17023. doi: 10.1038/sigtrans.2017.23
131. Cartwright T, Perkins ND, Wilson C. NFKB1: A Suppressor of Inflammation, Ageing and Cancer. FEBS J (2016) 283(10):1812–22. doi: 10.1111/febs.13627
132. Hou Y, Zhou Y, Gack MU, Lathia JD, Kallianpur A, Mehra R, et al. Multimodal Single-Cell Omics Analysis Identifies Epithelium–Immune Cell Interactions and Immune Vulnerability Associated With Sex Differences in COVID-19. Signal Transduction Targeted Ther (2021) 6(1):292. doi: 10.1038/s41392-021-00709-x
133. Wauters E, Van Mol P, Garg AD, Jansen S, Van Herck Y, Vanderbeke L, et al. Discriminating Mild From Critical COVID-19 by Innate and Adaptive Immune Single-Cell Profiling of Bronchoalveolar Lavages. Cell Res (2021) 31(3):272–90. doi: 10.1038/s41422-020-00455-9
134. Saichi M, Ladjemi MZ, Korniotis S, Rousseau C, Ait Hamou Z, Massenet-Regad L, et al. Single-Cell RNA Sequencing of Blood Antigen-Presenting Cells in Severe COVID-19 Reveals Multi-Process Defects in Antiviral Immunity. Nat Cell Biol (2021) 23(5):538–51. doi: 10.1038/s41556-021-00681-2
135. Sharma P, Pandey AK, Bhattacharyya DK. Determining Crucial Genes Associated With COVID-19 Based on COPD Findings✶,✶✶. Comput Biol Med (2021) 128:104126. doi: 10.1016/j.compbiomed.2020.104126
136. Meydan C, Madrer N, Soreq H. The Neat Dance of COVID-19: NEAT1, DANCR, and Co-Modulated Cholinergic RNAs Link to Inflammation. Front Immunol (2020) 11:590870(2638). doi: 10.3389/fimmu.2020.590870
137. Amini-Farsani Z, Yadollahi-Farsani M, Arab S, Forouzanfar F, Yadollahi M, Asgharzade S. Prediction and Analysis of microRNAs Involved in COVID-19 Inflammatory Processes Associated With the NF-kB and JAK/STAT Signaling Pathways. Int Immunopharmacol (2021) 100:108071. doi: 10.1016/j.intimp.2021.108071
138. Wang J, Huang R, Xu Q, Zheng G, Qiu G, Ge M, et al. Mesenchymal Stem Cell–Derived Extracellular Vesicles Alleviate Acute Lung Injury via Transfer of miR-27a-3p*. Crit Care Med (2020) 48(7):e599–610. doi: 10.1097/ccm.0000000000004315
139. Barh D, Aljabali AA, Tambuwala MM, Tiwari S, Serrano-Aroca Á, Alzahrani KJ, et al. Predicting COVID-19—Comorbidity Pathway Crosstalk-Based Targets and Drugs: Towards Personalized COVID-19 Management. Biomedicines (2021) 9(5):556. doi: 10.3390/biomedicines9050556
140. Creeden JF, Imami AS, Eby HM, Gillman C, Becker KN, Reigle J, et al. Fluoxetine as an Anti-Inflammatory Therapy in SARS-CoV-2 Infection. Biomed Pharmacother (2021) 138:111437. doi: 10.1016/j.biopha.2021.111437
141. Kang K, Kim HH, Choi Y. Tiotropium is Predicted to be a Promising Drug for COVID-19 Through Transcriptome-Based Comprehensive Molecular Pathway Analysis. Viruses (2020) 12(7):776. doi: 10.3390/v12070776
142. Jahani M, Dokaneheifard S, Mansouri K. Hypoxia: A Key Feature of COVID-19 Launching Activation of HIF-1 and Cytokine Storm. J Inflamm (2020) 17(1):33. doi: 10.1186/s12950-020-00263-3
143. Romeo-Guitart D, Leiva-Rodriguez T, Forés J, Casas C. Improved Motor Nerve Regeneration by SIRT1/Hif1a-Mediated Autophagy. Cells (2019) 8(11):1354. doi: 10.3390/cells8111354
144. AbdelMassih A, Yacoub E, Husseiny RJ, Kamel A, Hozaien R, El Shershaby M, et al. Hypoxia-Inducible Factor (HIF): The Link Between Obesity and COVID-19. Obes Med (2021) 22:100317. doi: 10.1016/j.obmed.2020.100317
145. Santoso CS, Li Z, Rottenberg JT, Liu X, Shen VX, Fuxman Bass JI. Therapeutic Targeting of Transcription Factors to Control the Cytokine Release Syndrome in COVID-19. Front Pharmacol (2021) 12:673485(1411). doi: 10.3389/fphar.2021.673485
146. Salgado-Albarrán M, Navarro-Delgado EI, Del Moral-Morales A, Alcaraz N, Baumbach J, González-Barrios R, et al. Comparative Transcriptome Analysis Reveals Key Epigenetic Targets in SARS-CoV-2 Infection. NPJ Syst Biol Appl (2021) 7(1):21. doi: 10.1038/s41540-021-00181-x
147. Desterke C, Turhan AG, Bennaceur-Griscelli A, Griscelli F. Pparγ Cistrome Repression During Activation of Lung Monocyte-Macrophages in Severe COVID-19. iScience (2020) 23(10):101611. doi: 10.1016/j.isci.2020.101611
148. Saha S, Halder AK, Bandyopadhyay SS, Chatterjee P, Nasipuri M, Bose D, et al. Drug Repurposing for COVID-19 Using Computational Screening: Is Fostamatinib/R406 a Potential Candidate? Methods (2021). doi: 10.1016/j.ymeth.2021.08.007
149. Tamayo-Velasco Á, Peñarrubia-Ponce MJ, Álvarez FJ, Gonzalo-Benito H, de la Fuente I, Martín-Fernández M, et al. Evaluation of Cytokines as Robust Diagnostic Biomarkers for COVID-19 Detection. J Pers Med (2021) 11(7):681. doi: 10.3390/jpm11070681
150. Laudanski K, Jihane H, Antalosky B, Ghani D, Phan U, Hernandez R, et al. Unbiased Analysis of Temporal Changes in Immune Serum Markers in Acute COVID-19 Infection With Emphasis on Organ Failure, Anti-Viral Treatment, and Demographic Characteristics. Front Immunol (2021) 12:650465(1966). doi: 10.3389/fimmu.2021.650465
151. Stolfi P, Manni L, Soligo M, Vergni D, Tieri P. Designing a Network Proximity-Based Drug Repurposing Strategy for COVID-19. Front Cell Dev Biol (2020) 8:545089(1021). doi: 10.3389/fcell.2020.545089
152. Nain Z, Rana HK, Liò P, Islam SMS, Summers MA, Moni MA. Pathogenetic Profiling of COVID-19 and SARS-Like Viruses. Briefings Bioinf (2020) 22(2):1175–96. doi: 10.1093/bib/bbaa173
153. He L, Zhang Q, Zhang Y, Fan Y, Yuan F, Li S. Single-Cell Analysis Reveals Cell Communication Triggered by Macrophages Associated With the Reduction and Exhaustion of CD8+ T Cells in COVID-19. Cell Commun Signaling (2021) 19(1):73. doi: 10.1186/s12964-021-00754-7
154. Islam H, Chamberlain TC, Mui AL, Little JP. Elevated Interleukin-10 Levels in COVID-19: Potentiation of Pro-Inflammatory Responses or Impaired Anti-Inflammatory Action? Front Immunol (2021) 12:677008(2485). doi: 10.3389/fimmu.2021.677008
155. Tang H, Gao Y, Li Z, Miao Y, Huang Z, Liu X, et al. The Noncoding and Coding Transcriptional Landscape of the Peripheral Immune Response in Patients With COVID-19. Clin Trans Med (2020) 10(6):e200. doi: 10.1002/ctm2.200
156. Johnson HM, Lewin AS, Ahmed CM. SOCS, Intrinsic Virulence Factors, and Treatment of COVID-19. Front Immunol (2020) 11:582102(2803). doi: 10.3389/fimmu.2020.582102
157. Bordoni V, Tartaglia E, Sacchi A, Fimia GM, Cimini E, Casetti R, et al. The Unbalanced P53/SIRT1 Axis may Impact Lymphocyte Homeostasis in COVID-19 Patients. Int J Infect Dis (2021) 105:49–53. doi: 10.1016/j.ijid.2021.02.019
158. de Oliveira M, De Sibio MT, Mathias LS, Rodrigues BM, Sakalem ME, Nogueira CR. Irisin Modulates Genes Associated With Severe Coronavirus Disease (COVID-19) Outcome in Human Subcutaneous Adipocytes Cell Culture. Mol Cell Endocrinol (2020) 515:110917. doi: 10.1016/j.mce.2020.110917
159. Wijst MGPVD, Vazquez SE, Hartoularos GC, Bastard P, Grant T, Bueno R, et al. Type I Interferon Autoantibodies are Associated With Systemic Immune Alterations in Patients With COVID-19. Sci Trans Med (2021) 0(0):eabh2624. doi: 10.1126/scitranslmed.abh2624
160. Wu C-T, Lidsky PV, Xiao Y, Lee IT, Cheng R, Nakayama T, et al. SARS-CoV-2 Infects Human Pancreatic β Cells and Elicits β Cell Impairment. Cell Metab (2021) 33(8):1565–76.e5. doi: 10.1016/j.cmet.2021.05.013
161. Geravandi S, Mahmoudi-aznaveh A, Azizi Z, Maedler K, Ardestani A. SARS-CoV-2 and Pancreas: A Potential Pathological Interaction? Trends Endocrinol Metab (2021) 32(11):842–5. doi: 10.1016/j.tem.2021.07.004
162. Hammoudeh SM, Hammoudeh AM, Bhamidimarri PM, Al Safar H, Mahboub B, Künstner A, et al. Systems Immunology Analysis Reveals the Contribution of Pulmonary and Extrapulmonary Tissues to the Immunopathogenesis of Severe COVID-19 Patients. Front Immunol (2021) 12:595150(2370). doi: 10.3389/fimmu.2021.595150
163. Yan Q, Li P, Ye X, Huang X, Feng B, Ji T, et al. Longitudinal Peripheral Blood Transcriptional Analysis Reveals Molecular Signatures of Disease Progression in COVID-19 Patients. J Immunol (2021) 206(9):2146–59. doi: 10.4049/jimmunol.2001325
164. Fließer E, Birnhuber A, Marsh LM, Gschwandtner E, Klepetko W, Olschewski H, et al. Dysbalance of ACE2 Levels – a Possible Cause for Severe COVID-19 Outcome in COPD. J Pathol: Clin Res (2021) 7(5):446–58. doi: 10.1002/cjp2.224
165. Aoe T. Pathological Aspects of COVID-19 as a Conformational Disease and the Use of Pharmacological Chaperones as a Potential Therapeutic Strategy. Front Pharmacol (2020) 11:1095(1095). doi: 10.3389/fphar.2020.01095
166. Islam ABMMK, Khan MA-A-K, Ahmed R, Hossain MS, Kabir SMT, Islam MS, et al. Transcriptome of Nasopharyngeal Samples From COVID-19 Patients and a Comparative Analysis With Other SARS-CoV-2 Infection Models Reveal Disparate Host Responses Against SARS-CoV-2. J Trans Med (2021) 19(1):32. doi: 10.1186/s12967-020-02695-0
167. Guo C, Li B, Ma H, Wang X, Cai P, Yu Q, et al. Single-Cell Analysis of Two Severe COVID-19 Patients Reveals a Monocyte-Associated and Tocilizumab-Responding Cytokine Storm. Nat Commun (2020) 11(1):3924. doi: 10.1038/s41467-020-17834-w
168. Tong M, Jiang Y, Xia D, Xiong Y, Zheng Q, Chen F, et al. Elevated Expression of Serum Endothelial Cell Adhesion Molecules in COVID-19 Patients. J Infect Dis (2020) 222(6):894–8. doi: 10.1093/infdis/jiaa349
169. Zheng W, Huang X, Lai Y, Liu X, Jiang Y, Zhan S. Glycyrrhizic Acid for COVID-19: Findings of Targeting Pivotal Inflammatory Pathways Triggered by SARS-CoV-2. Front Pharmacol (2021) 12:631206(1402). doi: 10.3389/fphar.2021.631206
170. Li X, Lin H, Wang Q, Cui L, Luo H, Luo L. Chemical Composition and Pharmacological Mechanism of Shenfu Decoction in the Treatment of Novel Coronavirus Pneumonia (COVID-19). Drug Dev Ind Pharm (2020) 46(12):1947–59. doi: 10.1080/03639045.2020.1826510
171. Chen G, Shaw MH, Kim Y-G, Nuñez G. NOD-Like Receptors: Role in Innate Immunity and Inflammatory Disease. Annu Rev Pathol: Mech Dis (2009) 4(1):365–98. doi: 10.1146/annurev.pathol.4.110807.092239
172. Krishnamoorthy P, Raj AS, Roy S, Kumar NS, Kumar H. Comparative Transcriptome Analysis of SARS-CoV, MERS-CoV, and SARS-CoV-2 to Identify Potential Pathways for Drug Repurposing. Comput Biol Med (2021) 128:104123. doi: 10.1016/j.compbiomed.2020.104123
173. Li Z, Huang Z, Li X, Huang C, Shen J, Li S, et al. Bioinformatic Analyses Hinted at Augmented T Helper 17 Cell Differentiation and Cytokine Response as the Central Mechanism of COVID-19–Associated Guillain-Barré Syndrome. Cell Proliferation (2021) 54(5):e13024. doi: 10.1111/cpr.13024
174. Freeman TL, Swartz TH. Targeting the NLRP3 Inflammasome in Severe COVID-19. Front Immunol (2020) 11:1518(1518). doi: 10.3389/fimmu.2020.01518
175. Huang Q, Wu X, Zheng X, Luo S, Xu S, Weng J. Targeting Inflammation and Cytokine Storm in COVID-19. Pharmacol Res (2020) 159:105051. doi: 10.1016/j.phrs.2020.105051
176. Liu T, Guo Y, Zhao J, He S, Bai Y, Wang N, et al. Systems Pharmacology and Verification of ShenFuHuang Formula in Zebrafish Model Reveal Multi-Scale Treatment Strategy for Septic Syndrome in COVID-19. Front Pharmacol (2020) 11:584057(1464). doi: 10.3389/fphar.2020.584057
177. Nailwal H, Chan FK-M. Necroptosis in Anti-Viral Inflammation. Cell Death Differ (2019) 26(1):4–13. doi: 10.1038/s41418-018-0172-x
178. Liu Y, Liu T, Lei T, Zhang D, Du S, Girani L, et al. RIP1/RIP3-Regulated Necroptosis as a Target for Multifaceted Disease Therapy (Review). Int J Mol Med (2019) 44(3):771–86. doi: 10.3892/ijmm.2019.4244
179. Frühbeck G, Catalán V, Valentí V, Moncada R, Gómez-Ambrosi J, Becerril S, et al. FNDC4 and FNDC5 Reduce SARS-CoV-2 Entry Points and Spike Glycoprotein S1-Induced Pyroptosis, Apoptosis, and Necroptosis in Human Adipocytes. Cell Mol Immunol (2021) 18(10):2457–9. doi: 10.1038/s41423-021-00762-0
180. Karki R, Sharma BR, Tuladhar S, Williams EP, Zalduondo L, Samir P, et al. Synergism of TNF-α and IFN-γ Triggers Inflammatory Cell Death, Tissue Damage, and Mortality in SARS-CoV-2 Infection and Cytokine Shock Syndromes. Cell (2021) 184(1):149–68.e17. doi: 10.1016/j.cell.2020.11.025
181. Li S, Zhang Y, Guan Z, Li H, Ye M, Chen X, et al. SARS-CoV-2 Triggers Inflammatory Responses and Cell Death Through Caspase-8 Activation. Signal Transduction Targeted Ther (2020) 5(1):235. doi: 10.1038/s41392-020-00334-0
182. Koupenova M, Corkrey HA, Vitseva O, Tanriverdi K, Somasundaran M, Liu P, et al. SARS-CoV-2 Initiates Programmed Cell Death in Platelets. Circ Res (2021) 129(6):631–46. doi: 10.1161/CIRCRESAHA.121.319117
183. Cao L, Mu W. Necrostatin-1 and Necroptosis Inhibition: Pathophysiology and Therapeutic Implications. Pharmacol Res (2021) 163:105297. doi: 10.1016/j.phrs.2020.105297
184. Kumar BV, Connors TJ, Farber DL. Human T Cell Development, Localization, and Function Throughout Life. Immunity (2018) 48(2):202–13. doi: 10.1016/j.immuni.2018.01.007
185. Marongiu L, Protti G, Facchini FA, Valache M, Mingozzi F, Ranzani V, et al. Maturation Signatures of Conventional Dendritic Cell Subtypes in COVID-19 Suggest Direct Viral Sensing. Eur J Immunol (2021). doi: 10.1002/eji.202149298
186. Tan L, Wang Q, Zhang D, Ding J, Huang Q, Tang Y-Q, et al. Lymphopenia Predicts Disease Severity of COVID-19: A Descriptive and Predictive Study. Signal Transduction Targeted Ther (2020) 5(1):33. doi: 10.1038/s41392-020-0148-4
187. Vardhana SA, Wolchok JD. The Many Faces of the Anti-COVID Immune responseA Review of the Anti-COVID Immune Response. J Exp Med (2020) 217(6):e20200678. doi: 10.1084/jem.20200678
188. Zheng M, Gao Y, Wang G, Song G, Liu S, Sun D, et al. Functional Exhaustion of Antiviral Lymphocytes in COVID-19 Patients. Cell Mol Immunol (2020) 17(5):533–5. doi: 10.1038/s41423-020-0402-2
189. Li R, Wu K, Li Y, Liang X, Lai KP, Chen J. Integrative Pharmacological Mechanism of Vitamin C Combined With Glycyrrhizic Acid Against COVID-19: Findings of Bioinformatics Analyses. Briefings Bioinf (2020) 22(2):1161–74. doi: 10.1093/bib/bbaa141
190. Toor SM, Saleh R, Sasidharan Nair V, Taha RZ, Elkord E. T-Cell Responses and Therapies Against SARS-CoV-2 Infection. Immunology (2021) 162(1):30–43. doi: 10.1111/imm.13262
191. Wu M, Chen Y, Xia H, Wang C, Tan CY, Cai X, et al. Transcriptional and Proteomic Insights Into the Host Response in Fatal COVID-19 Cases. Proc Natl Acad Sci (2020) 117(45):28336–43. doi: 10.1073/pnas.2018030117
192. Wang J, Li Q, Yin Y, Zhang Y, Cao Y, Lin X, et al. Excessive Neutrophils and Neutrophil Extracellular Traps in COVID-19. Front Immunol (2020) 11:2063(2063). doi: 10.3389/fimmu.2020.02063
193. Radermecker C, Detrembleur N, Guiot J, Cavalier E, Henket M, d’Emal C, et al. Neutrophil Extracellular Traps Infiltrate the Lung Airway, Interstitial, and Vascular Compartments in Severe COVID-19. J Exp Med (2020) 217(12):e20201012. doi: 10.1084/jem.20201012
194. Veras FP, Pontelli MC, Silva CM, Toller-Kawahisa JE, de Lima M, Nascimento DC, et al. SARS-CoV-2–Triggered Neutrophil Extracellular Traps Mediate COVID-19 Pathology. J Exp Med (2020) 217(12). doi: 10.1084/jem.20201129
195. Leppkes M, Knopf J, Naschberger E, Lindemann A, Singh J, Herrmann I, et al. Vascular Occlusion by Neutrophil Extracellular Traps in COVID-19. EBioMedicine (2020) 58:102925. doi: 10.1016/j.ebiom.2020.102925
196. Zuo Y, Zuo M, Yalavarthi S, Gockman K, Madison JA, Shi H, et al. Neutrophil Extracellular Traps and Thrombosis in COVID-19. J Thromb Thrombolysis (2021) 51(2):446–53. doi: 10.1007/s11239-020-02324-z
197. Middleton EA, He X-Y, Denorme F, Campbell RA, Ng D, Salvatore SP, et al. Neutrophil Extracellular Traps Contribute to Immunothrombosis in COVID-19 Acute Respiratory Distress Syndrome. Blood (2020) 136(10):1169–79. doi: 10.1182/blood.2020007008
198. Mills EL, Kelly B, O'Neill LAJ. Mitochondria are the Powerhouses of Immunity. Nat Immunol (2017) 18(5):488–98. doi: 10.1038/ni.3704
199. Holder K, Reddy PH. The COVID-19 Effect on the Immune System and Mitochondrial Dynamics in Diabetes, Obesity, and Dementia. Neuroscientist (2021) 27(4):331–9. doi: 10.1177/1073858420960443
200. Ganji R, Reddy PH. Impact of COVID-19 on Mitochondrial-Based Immunity in Aging and Age-Related Diseases. Front Aging Neurosci (2021) 12:614650(502). doi: 10.3389/fnagi.2020.614650
201. Singh KK, Chaubey G, Chen JY, Suravajhala P. Decoding SARS-CoV-2 Hijacking of Host Mitochondria in COVID-19 Pathogenesis. Am J Physiol-Cell Physiol (2020) 319(2):C258–C67. doi: 10.1152/ajpcell.00224.2020
202. Alfarouk KO, Alhoufie STS, Hifny A, Schwartz L, Alqahtani AS, Ahmed SBM, et al. Of Mitochondrion and COVID-19. J Enzyme Inhib Med Chem (2021) 36(1):1258–67. doi: 10.1080/14756366.2021.1937144
203. Ouyang L, Gong J. Mitochondrial-Targeted Ubiquinone: A Potential Treatment for COVID-19. Med Hypotheses (2020) 144:110161. doi: 10.1016/j.mehy.2020.110161
204. Mittal M, Siddiqui MR, Tran K, Reddy SP, Malik AB. Reactive Oxygen Species in Inflammation and Tissue Injury. Antioxid Redox Signaling (2013) 20(7):1126–67. doi: 10.1089/ars.2012.5149
205. Naha PC, Davoren M, Lyng FM, Byrne HJ. Reactive Oxygen Species (ROS) Induced Cytokine Production and Cytotoxicity of PAMAM Dendrimers in J774A.1 Cells. Toxicol Appl Pharmacol (2010) 246(1):91–9. doi: 10.1016/j.taap.2010.04.014
206. Saleh J, Peyssonnaux C, Singh KK, Edeas M. Mitochondria and Microbiota Dysfunction in COVID-19 Pathogenesis. Mitochondrion (2020) 54:1–7. doi: 10.1016/j.mito.2020.06.008
207. López-Cortés A, Guevara-Ramírez P, Kyriakidis NC, Barba-Ostria C, León Cáceres Á, Guerrero S, et al. In Silico Analyses of Immune System Protein Interactome Network, Single-Cell RNA Sequencing of Human Tissues, and Artificial Neural Networks Reveal Potential Therapeutic Targets for Drug Repurposing Against COVID-19. Front Pharmacol (2021) 12:598925(63). doi: 10.3389/fphar.2021.598925
208. Seethy AA, Singh S, Mukherjee I, Pethusamy K, Purkayastha K, Sharma JB, et al. Potential SARS-CoV-2 Interactions With Proteins Involved in Trophoblast Functions – An in-Silico Study. Placenta (2021) 103:141–51. doi: 10.1016/j.placenta.2020.10.027
209. Das JK, Roy S, Guzzi PH. Analyzing Host-Viral Interactome of SARS-CoV-2 for Identifying Vulnerable Host Proteins During COVID-19 Pathogenesis. Infect Genet Evol (2021) 93:104921. doi: 10.1016/j.meegid.2021.104921
210. Islam ABMMK, Khan MA-A-K. Lung Transcriptome of a COVID-19 Patient and Systems Biology Predictions Suggest Impaired Surfactant Production Which may be Druggable by Surfactant Therapy. Sci Rep (2020) 10(1):19395. doi: 10.1038/s41598-020-76404-8
211. Mulay A, Konda B, Garcia G Jr., Yao C, Beil S, Villalba JM, et al. SARS-CoV-2 Infection of Primary Human Lung Epithelium for COVID-19 Modeling and Drug Discovery. Cell Rep (2021) 35(5):109055. doi: 10.1016/j.celrep.2021.109055
212. Ganguly B. A Parsimonious Approach for Recognizing SARS-CoV-2 and Host Interactions. J Med Virol (2021) 93(7):4576–84. doi: 10.1002/jmv.26824
213. Sarma A, Christenson SA, Byrne A, Mick E, Pisco AO, DeVoe C, et al. Tracheal Aspirate RNA Sequencing Identifies Distinct Immunological Features of COVID-19 ARDS. Nat Commun (2021) 12(1):5152. doi: 10.1038/s41467-021-25040-5
214. Zhu Y, Feng F, Hu G, Wang Y, Yu Y, Zhu Y, et al. A Genome-Wide CRISPR Screen Identifies Host Factors That Regulate SARS-CoV-2 Entry. Nat Commun (2021) 12(1):961. doi: 10.1038/s41467-021-21213-4
215. Daniloski Z, Jordan TX, Wessels H-H, Hoagland DA, Kasela S, Legut M, et al. Identification of Required Host Factors for SARS-CoV-2 Infection in Human Cells. Cell (2021) 184(1):92–105.e16. doi: 10.1016/j.cell.2020.10.030
216. Shao Y, Saredy J, Xu K, Sun Y, Saaoud F, Drummer C, et al. Endothelial Immunity Trained by Coronavirus Infections, DAMP Stimulations and Regulated by Anti-Oxidant NRF2 may Contribute to Inflammations, Myelopoiesis, COVID-19 Cytokine Storms and Thromboembolism. Front Immunol (2021) 12:653110(2027). doi: 10.3389/fimmu.2021.653110
217. Yan H, Liang X, Du J, He Z, Wang Y, Lyu M, et al. Proteomic and Metabolomic Investigation of Serum Lactate Dehydrogenase Elevation in COVID-19 Patients. Proteomics (2021) 21(15):2100002. doi: 10.1002/pmic.202100002
218. Saheb Sharif-Askari N, Saheb Sharif-Askari F, Mdkhana B, Hussain Alsayed HA, Alsafar H, Alrais ZF, et al. Upregulation of Oxidative Stress Gene Markers During SARS-COV-2 Viral Infection. Free Radical Biol Med (2021) 172:688–98. doi: 10.1016/j.freeradbiomed.2021.06.018
219. Ricke-Hoch M, Stelling E, Lasswitz L, Gunesch AP, Kasten M, Zapatero-Belinchón FJ, et al. Impaired Immune Response Mediated by Prostaglandin E2 Promotes Severe COVID-19 Disease. PloS One (2021) 16(8):e0255335. doi: 10.1371/journal.pone.0255335
220. Robb CT, Goepp M, Rossi AG, Yao C. Non-Steroidal Anti-Inflammatory Drugs, Prostaglandins, and COVID-19. Br J Pharmacol (2020) 177(21):4899–920. doi: 10.1111/bph.15206
221. Khatoon F, Prasad K, Kumar V. Neurological Manifestations of COVID-19: Available Evidences and a New Paradigm. J NeuroVirol (2020) 26(5):619–30. doi: 10.1007/s13365-020-00895-4
222. Abedi F, Rezaee R, Karimi G. Plausibility of Therapeutic Effects of Rho Kinase Inhibitors Against Severe Acute Respiratory Syndrome Coronavirus 2 (COVID-19). Pharmacol Res (2020) 156:104808. doi: 10.1016/j.phrs.2020.104808
223. Dolan ME, Hill DP, Mukherjee G, McAndrews MS, Chesler EJ, Blake JA. Investigation of COVID-19 Comorbidities Reveals Genes and Pathways Coincident With the SARS-CoV-2 Viral Disease. Sci Rep (2020) 10(1):20848. doi: 10.1038/s41598-020-77632-8
224. Schneider WM, Luna JM, Hoffmann HH, Sánchez-Rivera FJ, Leal AA, Ashbrook AW, et al. Genome-Scale Identification of SARS-CoV-2 and Pan-Coronavirus Host Factor Networks. Cell (2021) 184(1):120–32.e14. doi: 10.1016/j.cell.2020.12.006
225. Maras JS, Sharma S, Bhat A, Rooge S, aggrawal R, Gupta E, et al. Multi-Omics Analysis of Respiratory Specimen Characterizes Baseline Molecular Determinants Associated With SARS-CoV-2 Outcome. iScience (2021) 24(8):102823. doi: 10.1016/j.isci.2021.102823
226. Hachim MY, Al Heialy S, Senok A, Hamid Q, Alsheikh-Ali A. Molecular Basis of Cardiac and Vascular Injuries Associated With COVID-19. Front Cardiovasc Med (2020) 7:582399(203). doi: 10.3389/fcvm.2020.582399
227. O’Carroll SM, O’Neill LAJ. Targeting Immunometabolism to Treat COVID-19. Immunother Adv (2021) 1(1):ltab013. doi: 10.1093/immadv/ltab013
228. Wang F, Huang S, Gao R, Zhou Y, Lai C, Li Z, et al. Initial Whole-Genome Sequencing and Analysis of the Host Genetic Contribution to COVID-19 Severity and Susceptibility. Cell Discov (2020) 6(1):83. doi: 10.1038/s41421-020-00231-4
229. Tiwari R, Mishra AR, Gupta A, Nayak D. Structural Similarity-Based Prediction of Host Factors Associated With SARS-CoV-2 Infection and Pathogenesis. J Biomol Struct Dyn (2021) 28:1–12. doi: 10.1080/07391102.2021.1874532
230. Feng L, Yin Y-Y, Liu C-H, Xu K-R, Li Q-R, Wu J-R, et al. Proteome-Wide Data Analysis Reveals Tissue-Specific Network Associated With SARS-CoV-2 Infection. J Mol Cell Biol (2020) 12(12):946–57. doi: 10.1093/jmcb/mjaa033
231. Doykov I, Hällqvist J, Gilmour K, Grandjean L, Mills K, Heywood W. 'The Long Tail of Covid-19' - The Detection of a Prolonged Inflammatory Response After a SARS-CoV-2 Infection in Asymptomatic and Mildly Affected Patients. F1000Research (2021) 9:1349. doi: 10.12688/f1000research.27287.2
232. Satu MS, Khan MI, Rahman MR, Howlader KC, Roy S, Roy SS, et al. Diseasome and Comorbidities Complexities of SARS-CoV-2 Infection With Common Malignant Diseases. Briefings Bioinf (2021) 22(2):1415–29. doi: 10.1093/bib/bbab003
233. McFarland AJ, Yousuf MS, Shiers S, Price TJ. Neurobiology of SARS-CoV-2 Interactions With the Peripheral Nervous System: Implications for COVID-19 and Pain. Pain Rep (2021) 6(1):e885. doi: 10.1097/pr9.0000000000000885
234. Hadjadj J, Yatim N, Barnabei L, Corneau A, Boussier J, Smith N, et al. Impaired Type I Interferon Activity and Inflammatory Responses in Severe COVID-19 Patients. Science (2020) 369(6504):718–24. doi: 10.1126/science.abc6027
235. Rodrigues TS, de Sá KSG, Ishimoto AY, Becerra A, Oliveira S, Almeida L, et al. Inflammasomes are Activated in Response to SARS-CoV-2 Infection and are Associated With COVID-19 Severity in Patients. J Exp Med (2020) 218(3):e20201707. doi: 10.1084/jem.20201707
236. Meng Y, Zhang Q, Wang K, Zhang X, Yang R, Bi K, et al. RBM15-Mediated N6-Methyladenosine Modification Affects COVID-19 Severity by Regulating the Expression of Multitarget Genes. Cell Death Dis (2021) 12(8):732. doi: 10.1038/s41419-021-04012-z
237. Yang C-A, Huang Y-L, Chiang B-L. Innate Immune Response Analysis in COVID-19 and Kawasaki Disease Reveals MIS-C Predictors. J Formosan Med Assoc (2021). doi: 10.1016/j.jfma.2021.06.009
238. Nagaraja S, Jain D, Kesavardhana S. Inflammasome Regulation in Driving COVID-19 Severity in Humans and Immune Tolerance in Bats. J Leukocyte Biol (2021). doi: 10.1002/JLB.4COVHR0221-093RR
239. Jamilloux Y, Henry T, Belot A, Viel S, Fauter M, El Jammal T, et al. Should We Stimulate or Suppress Immune Responses in COVID-19? Cytokine and Anti-Cytokine Interventions. Autoimmun Rev (2020) 19(7):102567. doi: 10.1016/j.autrev.2020.102567
240. Keller M, Rüegg A, Werner S, Beer H-D. Active Caspase-1 is a Regulator of Unconventional Protein Secretion. Cell (2008) 132(5):818–31. doi: 10.1016/j.cell.2007.12.040
241. Soy M, Keser G, Atagündüz P, Tabak F, Atagündüz I, Kayhan S. Cytokine Storm in COVID-19: Pathogenesis and Overview of Anti-Inflammatory Agents Used in Treatment. Clin Rheumatol (2020) 39(7):2085–94. doi: 10.1007/s10067-020-05190-5
242. Shi J, Zhao Y, Wang K, Shi X, Wang Y, Huang H, et al. Cleavage of GSDMD by Inflammatory Caspases Determines Pyroptotic Cell Death. Nature (2015) 526(7575):660–5. doi: 10.1038/nature15514
243. Ghaleh HEG, Hosseini A, Aghamollaei H, Fasihi-Ramandi M, Alishiri G, Saeedi-Boroujeni A, et al. NLRP3 Inflammasome Activation and Oxidative Stress Status in the Mild and Moderate SARS-CoV-2 Infected Patients: Impact of Melatonin as a Medicinal Supplement. Z für Naturforsch C (2021) 000010151520210101). doi: 10.1515/znc-2021-0101
244. Wu Q, Coumoul X, Grandjean P, Barouki R, Audouze K. Endocrine Disrupting Chemicals and COVID-19 Relationships: A Computational Systems Biology Approach. Environ Int (2021) 157:106232. doi: 10.1016/j.envint.2020.106232
245. Crespo-Facorro B, Ruiz-Veguilla M, Vázquez-Bourgon J, Sánchez-Hidalgo AC, Garrido-Torres N, Cisneros JM, et al. Aripiprazole as a Candidate Treatment of COVID-19 Identified Through Genomic Analysis. Front Pharmacol (2021) 12:646701(346). doi: 10.3389/fphar.2021.646701
246. Yang J, Chen C, Chen W, Huang L, Fu Z, Ye K, et al. Proteomics and Metabonomics Analyses of Covid-19 Complications in Patients With Pulmonary Fibrosis. Sci Rep (2021) 11(1):14601. doi: 10.1038/s41598-021-94256-8
247. Kenig A, Ishay Y, Kharouf F, Rubin L. Treatment of B-Cell Depleted COVID-19 Patients With Convalescent Plasma and Plasma-Based Products. Clin Immunol (2021) 227:108723. doi: 10.1016/j.clim.2021.108723
248. Lenti MV, Aronico N, Pellegrino I, Boveri E, Giuffrida P, Borrelli de Andreis F, et al. Depletion of Circulating IgM Memory B Cells Predicts Unfavourable Outcome in COVID-19. Sci Rep (2020) 10(1):20836. doi: 10.1038/s41598-020-77945-8
249. Sosa-Hernández VA, Torres-Ruíz J, Cervantes-Díaz R, Romero-Ramírez S, Páez-Franco JC, Meza-Sánchez DE, et al. B Cell Subsets as Severity-Associated Signatures in COVID-19 Patients. Front Immunol (2020) 11:611004(3244). doi: 10.3389/fimmu.2020.611004
250. Xiao Y, Shi X, She Q, Chen Q, Pan H, Zhang J, et al. Exploration of Turn-Positive RT-PCR Results and Factors Related to Treatment Outcome in COVID-19: A Retrospective Cohort Study. Virulence (2020) 11(1):1250–6. doi: 10.1080/21505594.2020.1816076
251. Delshad M, Tavakolinia N, Pourbagheri-Sigaroodi A, Safaroghli-Azar A, Bagheri N, Bashash D. The Contributory Role of Lymphocyte Subsets, Pathophysiology of Lymphopenia and its Implication as Prognostic and Therapeutic Opportunity in COVID-19. Int Immunopharmacol (2021) 95:107586. doi: 10.1016/j.intimp.2021.107586
252. Wen W, Su W, Tang H, Le W, Zhang X, Zheng Y, et al. Immune Cell Profiling of COVID-19 Patients in the Recovery Stageby Single-Cell Sequencing. Cell Discov (2020) 6(1):31. doi: 10.1038/s41421-020-0168-9
253. Sun J, Zhuang Z, Zheng J, Li K, Wong RL-Y, Liu D, et al. Generation of a Broadly Useful Model for COVID-19 Pathogenesis, Vaccination, and Treatment. Cell (2020) 182(3):734–43.e5. doi: 10.1016/j.cell.2020.06.010
254. Tan Y, Zhou J, Zhou Q, Hu L, Long Y. Role of Eosinophils in the Diagnosis and Prognostic Evaluation of COVID-19. J Med Virol (2021) 93(2):1105–10. doi: 10.1002/jmv.26506
255. Stephenson E, Reynolds G, Botting RA, Calero-Nieto FJ, Morgan MD, Tuong ZK, et al. Single-Cell Multi-Omics Analysis of the Immune Response in COVID-19. Nat Med (2021) 27(5):904–16. doi: 10.1038/s41591-021-01329-2
256. Li C-X, Chen J, Lv S-K, Li J-H, Li L-L, Hu X. Whole-Transcriptome RNA Sequencing Reveals Significant Differentially Expressed mRNAs, miRNAs, and lncRNAs and Related Regulating Biological Pathways in the Peripheral Blood of COVID-19 Patients. Mediators Inflamm (2021) 2021:6635925. doi: 10.1155/2021/6635925
257. Ren X, Wen W, Fan X, Hou W, Su B, Cai P, et al. COVID-19 Immune Features Revealed by a Large-Scale Single-Cell Transcriptome Atlas. Cell (2021) 184(7):1895–913.e19. doi: 10.1016/j.cell.2021.01.053
258. Zhang C, Wu Z, Li J-W, Zhao H, Wang G-Q. Cytokine Release Syndrome in Severe COVID-19: Interleukin-6 Receptor Antagonist Tocilizumab may be the Key to Reduce Mortality. Int J Antimicrob Agents (2020) 55(5):105954. doi: 10.1016/j.ijantimicag.2020.105954
259. De Biasi S, Meschiari M, Gibellini L, Bellinazzi C, Borella R, Fidanza L, et al. Marked T Cell Activation, Senescence, Exhaustion and Skewing Towards TH17 in Patients With COVID-19 Pneumonia. Nat Commun (2020) 11(1):3434. doi: 10.1038/s41467-020-17292-4
260. Jones BE, Maerz MD, Buckner JH. IL-6: A Cytokine at the Crossroads of Autoimmunity. Curr Opin Immunol (2018) 55:9–14. doi: 10.1016/j.coi.2018.09.002
261. Bettelli E, Carrier Y, Gao W, Korn T, Strom TB, Oukka M, et al. Reciprocal Developmental Pathways for the Generation of Pathogenic Effector TH17 and Regulatory T Cells. Nature (2006) 441(7090):235–8. doi: 10.1038/nature04753
262. Yu X, Li L, Chan MTV, Wu WKK. Bioinformatic Analyses Suggest Augmented Interleukin-17 Signaling as the Mechanism of COVID-19-Associated Herpes Zoster. Environ Sci Pollut Res (2021) 28(46):65769–75. doi: 10.1007/s11356-021-15567-x
263. Wu D, Yang XO. TH17 Responses in Cytokine Storm of COVID-19: An Emerging Target of JAK2 Inhibitor Fedratinib. J Microbiol Immunol Infect (2020) 53(3):368–70. doi: 10.1016/j.jmii.2020.03.005
264. Egwuagu CE. STAT3 in CD4+ T Helper Cell Differentiation and Inflammatory Diseases. Cytokine (2009) 47(3):149–56. doi: 10.1016/j.cyto.2009.07.003
265. Muyayalo KP, Huang D-H, Zhao S-J, Xie T, Mor G, Liao A-H. COVID-19 and Treg/Th17 Imbalance: Potential Relationship to Pregnancy Outcomes. Am J Reprod Immunol (2020) 84(5):e13304. doi: 10.1111/aji.13304
266. Kimura A, Kishimoto T. IL-6: Regulator of Treg/Th17 Balance. Eur J Immunol (2010) 40(7):1830–5. doi: 10.1002/eji.201040391
267. Tahmasebi S, El-Esawi MA, Mahmoud ZH, Timoshin A, Valizadeh H, Roshangar L, et al. Immunomodulatory Effects of Nanocurcumin on Th17 Cell Responses in Mild and Severe COVID-19 Patients. J Cell Physiol (2021) 236(7):5325–38. doi: 10.1002/jcp.30233
268. Qi F, Zhang W, Huang J, Fu L, Zhao J. Single-Cell RNA Sequencing Analysis of the Immunometabolic Rewiring and Immunopathogenesis of Coronavirus Disease 2019. Front Immunol (2021) 12:651656(1261). doi: 10.3389/fimmu.2021.651656
269. Joshi C, Jadeja V, Zhou H. Molecular Mechanisms of Palmitic Acid Augmentation in COVID-19 Pathologies. Int J Mol Sci (2021) 22(13):7127. doi: 10.3390/ijms22137127
270. Bernardes JP, Mishra N, Tran F, Bahmer T, Best L, Blase JI, et al. Longitudinal Multi-Omics Analyses Identify Responses of Megakaryocytes, Erythroid Cells, and Plasmablasts as Hallmarks of Severe COVID-19. Immunity (2020) 53(6):1296–314.e9. doi: 10.1016/j.immuni.2020.11.017
271. Kumar V. How Could We Forget Immunometabolism in SARS-CoV2 Infection or COVID-19? Int Rev Immunol (2021) 40(1-2):72–107. doi: 10.1080/08830185.2020.1840567
272. Wilk AJ, Rustagi A, Zhao NQ, Roque J, Martínez-Colón GJ, McKechnie JL, et al. A Single-Cell Atlas of the Peripheral Immune Response in Patients With Severe COVID-19. Nat Med (2020) 26(7):1070–6. doi: 10.1038/s41591-020-0944-y
273. Ahmad A, Rehman MU, Ahmad P, Alkharfy KM. Covid-19 and Thymoquinone: Connecting the Dots. Phytother Res (2020) 34(11):2786–9. doi: 10.1002/ptr.6793
274. Mohkhedkar M, Venigalla SSK, Janakiraman V. Autoantigens That may Explain Postinfection Autoimmune Manifestations in Patients With Coronavirus Disease 2019 Displaying Neurological Conditions. J Infect Dis (2020) 223(3):536–7. doi: 10.1093/infdis/jiaa703
275. Zhou Y, Hou Y, Shen J, Mehra R, Kallianpur A, Culver DA, et al. A Network Medicine Approach to Investigation and Population-Based Validation of Disease Manifestations and Drug Repurposing for COVID-19. PloS Biol (2020) 18(11):e3000970. doi: 10.1371/journal.pbio.3000970
276. Huang J, Tao G, Liu J, Cai J, Huang Z, Chen J-X. Current Prevention of COVID-19: Natural Products and Herbal Medicine. Front Pharmacol (2020) 11:588508(1635). doi: 10.3389/fphar.2020.588508
277. Elfiky AA. Natural Products may Interfere With SARS-CoV-2 Attachment to the Host Cell. J Biomol Struct Dyn (2021) 39(9):3194–203. doi: 10.1080/07391102.2020.1761881
278. Nashiry MA, Sumi SS, Sharif Shohan MU, Alyami SA, Azad AKM, Moni MA. Bioinformatics and System Biology Approaches to Identify the Diseasome and Comorbidities Complexities of SARS-CoV-2 Infection With the Digestive Tract Disorders. Briefings Bioinf (2021). doi: 10.1093/bib/bbab126
279. Balaci L, Spada MC, Olla N, Sole G, Loddo L, Anedda F, et al. IRAK-M is Involved in the Pathogenesis of Early-Onset Persistent Asthma. Am J Hum Genet (2007) 80(6):1103–14. doi: 10.1086/518259
280. Chen X, Yin Y-H, Zhang M-Y, Liu J-Y, Li R, Qu Y-Q. Investigating the Mechanism of ShuFeng JieDu Capsule for the Treatment of Novel Coronavirus Pneumonia (COVID-19) Based on Network Pharmacology. Int J Med Sci (2020) 17(16):2511–30. doi: 10.7150/ijms.46378
281. Giovannoni F, Li Z, Remes-Lenicov F, Dávola ME, Elizalde M, Paletta A, et al. AHR Signaling is Induced by Infection With Coronaviruses. Nat Commun (2021) 12(1):5148. doi: 10.1038/s41467-021-25412-x
282. Galani I-E, Rovina N, Lampropoulou V, Triantafyllia V, Manioudaki M, Pavlos E, et al. Untuned Antiviral Immunity in COVID-19 Revealed by Temporal Type I/III Interferon Patterns and Flu Comparison. Nat Immunol (2021) 22(1):32–40. doi: 10.1038/s41590-020-00840-x
283. Zhang J-Y, Wang X-M, Xing X, Xu Z, Zhang C, Song J-W, et al. Single-Cell Landscape of Immunological Responses in Patients With COVID-19. Nat Immunol (2020) 21(9):1107–18. doi: 10.1038/s41590-020-0762-x
284. Zhang F, Gan R, Zhen Z, Hu X, Li X, Zhou F, et al. Adaptive Immune Responses to SARS-CoV-2 Infection in Severe Versus Mild Individuals. Signal Transduction Targeted Ther (2020) 5(1):156. doi: 10.1038/s41392-020-00263-y
285. Xu G, Qi F, Li H, Yang Q, Wang H, Wang X, et al. The Differential Immune Responses to COVID-19 in Peripheral and Lung Revealed by Single-Cell RNA Sequencing. Cell Discov (2020) 6(1):73. doi: 10.1038/s41421-020-00225-2
286. Chua RL, Lukassen S, Trump S, Hennig BP, Wendisch D, Pott F, et al. COVID-19 Severity Correlates With Airway Epithelium–Immune Cell Interactions Identified by Single-Cell Analysis. Nat Biotechnol (2020) 38(8):970–9. doi: 10.1038/s41587-020-0602-4
287. Lee H, Park J, Im H-J, Na KJ, Choi H. Discovery of Potential Imaging and Therapeutic Targets for Severe Inflammation in COVID-19 Patients. Sci Rep (2021) 11(1):14151. doi: 10.1038/s41598-021-93743-2
288. Song J-W, Zhang C, Fan X, Meng F-P, Xu Z, Xia P, et al. Immunological and Inflammatory Profiles in Mild and Severe Cases of COVID-19. Nat Commun (2020) 11(1):3410. doi: 10.1038/s41467-020-17240-2
289. Bieberich F, Vazquez-Lombardi R, Yermanos A, Ehling RA, Mason DM, Wagner B, et al. A Single-Cell Atlas of Lymphocyte Adaptive Immune Repertoires and Transcriptomes Reveals Age-Related Differences in Convalescent COVID-19 Patients. Front Immunol (2021) 12:701085(2737). doi: 10.3389/fimmu.2021.701085
290. Meckiff BJ, Ramírez-Suástegui C, Fajardo V, Chee SJ, Kusnadi A, Simon H, et al. Imbalance of Regulatory and Cytotoxic SARS-CoV-2-Reactive CD4+ T Cells in COVID-19. Cell (2020) 183(5):1340–53.e16. doi: 10.1016/j.cell.2020.10.001
291. Su Y, Chen D, Yuan D, Lausted C, Choi J, Dai CL, et al. Multi-Omics Resolves a Sharp Disease-State Shift Between Mild and Moderate COVID-19. Cell (2020) 183(6):1479–95.e20. doi: 10.1016/j.cell.2020.10.037
292. Ahmad R, Sindhu STAK, Tran P, Toma E, Morisset R, Menezes J, et al. Modulation of Expression of the MHC Class I-Binding Natural Killer Cell Receptors, and NK Activity in Relation to Viral Load in HIV-Infected/AIDS Patients. J Med Virol (2001) 65(3):431–40. doi: 10.1002/jmv.2053
293. National Research Project for SARS BG. The Involvement of Natural Killer Cells in the Pathogenesis of Severe Acute Respiratory Syndrome. Am J Clin Pathol (2004) 121(4):507–11. doi: 10.1309/wpk7y2xknf4cbf3r
294. Market M, Angka L, Martel AB, Bastin D, Olanubi O, Tennakoon G, et al. Flattening the COVID-19 Curve With Natural Killer Cell Based Immunotherapies. Front Immunol (2020) 11:1512(1512). doi: 10.3389/fimmu.2020.01512
295. Pipkin ME, Lieberman J. Delivering the Kiss of Death: Progress on Understanding How Perforin Works. Curr Opin Immunol (2007) 19(3):301–8. doi: 10.1016/j.coi.2007.04.011
296. Voskoboinik I, Smyth MJ, Trapani JA. Perforin-Mediated Target-Cell Death and Immune Homeostasis. Nat Rev Immunol (2006) 6(12):940–52. doi: 10.1038/nri1983
297. Voskoboinik I, Whisstock JC, Trapani JA. Perforin and Granzymes: Function, Dysfunction and Human Pathology. Nat Rev Immunol (2015) 15(6):388–400. doi: 10.1038/nri3839
298. Trapani JA, Smyth MJ. Functional Significance of the Perforin/Granzyme Cell Death Pathway. Nat Rev Immunol (2002) 2(10):735–47. doi: 10.1038/nri911
299. Lee JW, Su Y, Baloni P, Chen D, Pavlovitch-Bedzyk AJ, Yuan D, et al. Integrated Analysis of Plasma and Single Immune Cells Uncovers Metabolic Changes in Individuals With COVID-19. Nat Biotechnol (2021). doi: 10.1038/s41587-021-01020-4
300. Chu R, van Eeden C, Suresh S, Sligl WI, Osman M, Cohen Tervaert JW. Do COVID-19 Infections Result in a Different Form of Secondary Hemophagocytic Lymphohistiocytosis. Int J Mol Sci (2021) 22(6):2967. doi: 10.3390/ijms22062967
301. Soy M, Atagündüz P, Atagündüz I, Sucak GT. Hemophagocytic Lymphohistiocytosis: A Review Inspired by the COVID-19 Pandemic. Rheumatol Int (2021) 41(1):7–18. doi: 10.1007/s00296-020-04636-y
302. Sieni E, Cetica V, Hackmann Y, Coniglio ML, Da Ros M, Ciambotti B, et al. Familial Hemophagocytic Lymphohistiocytosis: When Rare Diseases Shed Light on Immune System Functioning. Front Immunol (2014) 5:167(167). doi: 10.3389/fimmu.2014.00167
303. Ahmed F, Jo D-H, Lee S-H. Can Natural Killer Cells be a Principal Player in Anti-SARS-CoV-2 Immunity? Front Immunol (2020) 11:586765(3246). doi: 10.3389/fimmu.2020.586765
304. Ge C, He Y. In Silico Prediction of Molecular Targets of Astragaloside IV for Alleviation of COVID-19 Hyperinflammation by Systems Network Pharmacology and Bioinformatic Gene Expression Analysis. Front Pharmacol (2020) 11:556984(1494). doi: 10.3389/fphar.2020.556984
305. Li S, Wu B, Ling Y, Guo M, Qin B, Ren X, et al. Epigenetic Landscapes of Single-Cell Chromatin Accessibility and Transcriptomic Immune Profiles of T Cells in COVID-19 Patients. Front Immunol (2021) 12:625881(385). doi: 10.3389/fimmu.2021.625881
306. Fineschi S. Case Report: Systemic Sclerosis After Covid-19 Infection. Front Immunol (2021) 12:686699(2439). doi: 10.3389/fimmu.2021.686699
307. Kalfaoglu B, Almeida-Santos J, Tye CA, Satou Y, Ono M. T-Cell Hyperactivation and Paralysis in Severe COVID-19 Infection Revealed by Single-Cell Analysis. Front Immunol (2020) 11:589380(2605). doi: 10.3389/fimmu.2020.589380
308. de Moraes D, VBP B, Santiloni Cury S, Ludwig RGuimarães, Araújo Junior JoãoP, Mori MADS, et al. Prediction of SARS-CoV Interaction With Host Proteins During Lung Aging Reveals a Potential Role for TRIB3 in COVID-19. Aging Dis (2021) 12(1):42–9. doi: 10.14336/ad.2020.1112
309. Bharat A, Querrey M, Markov NS, Kim S, Kurihara C, Garza-Castillon R, et al. Lung Transplantation for Patients With Severe COVID-19. Sci Trans Med (2020) 12(574):eabe4282. doi: 10.1126/scitranslmed.abe4282
310. Chu C-F, Sabath F, Fibi-Smetana S, Sun S, Öllinger R, Noeßner E, et al. Convalescent COVID-19 Patients Without Comorbidities Display Similar Immunophenotypes Over Time Despite Divergent Disease Severities. Front Immunol (2021) 12:601080(3182). doi: 10.3389/fimmu.2021.601080
311. Patterson BK, Guevara-Coto J, Yogendra R, Francisco EB, Long E, Pise A, et al. Immune-Based Prediction of COVID-19 Severity and Chronicity Decoded Using Machine Learning. Front Immunol (2021) 12:700782(2520). doi: 10.3389/fimmu.2021.700782
312. Sur S, Khatun M, Steele R, Isbell TS, Ray R, Ray RB. Exosomes From COVID-19 Patients Carry Tenascin-C and Fibrinogen-β in Triggering Inflammatory Signals in Cells of Distant Organ. Int J Mol Sci (2021) 22(6):3184. doi: 10.3390/ijms22063184
313. Teixeira PC, Dorneles GP, Santana Filho PC, da Silva IM, Schipper LL, Postiga IAL, et al. Increased LPS Levels Coexist With Systemic Inflammation and Result in Monocyte Activation in Severe COVID-19 Patients. Int Immunopharmacol (2021) 100:108125. doi: 10.1016/j.intimp.2021.108125
314. Patterson BK, Seethamraju H, Dhody K, Corley MJ, Kazempour K, Lalezari J, et al. CCR5 Inhibition in Critical COVID-19 Patients Decreases Inflammatory Cytokines, Increases CD8 T-Cells, and Decreases SARS-CoV2 RNA in Plasma by Day 14. Int J Infect Dis (2021) 103:25–32. doi: 10.1016/j.ijid.2020.10.101
315. Blot M, Bour J-B, Quenot JP, Bourredjem A, Nguyen M, Guy J, et al. The Dysregulated Innate Immune Response in Severe COVID-19 Pneumonia That Could Drive Poorer Outcome. J Trans Med (2020) 18(1):457. doi: 10.1186/s12967-020-02646-9
316. Zhao Y, Qin L, Zhang P, Li K, Liang L, Sun J, et al. Longitudinal COVID-19 Profiling Associates IL-1RA and IL-10 With Disease Severity and RANTES With Mild Disease. JCI Insight (2020) 5(13):e139834. doi: 10.1172/jci.insight.139834
317. Haslbauer JD, Matter MS, Stalder AK, Tzankov A. Histomorphological Patterns of Regional Lymph Nodes in COVID-19 Lungs. Der Pathologe (2021). doi: 10.1007/s00292-021-00945-6
318. Chen L, Zhang Q, Yu C, Wang F, Kong X. Functional Roles of CCL5/RANTES in Liver Disease. Liver Res (2020) 4(1):28–34. doi: 10.1016/j.livres.2020.01.002
319. Yen Y-T, Liao F, Hsiao C-H, Kao C-L, Chen Y-C, Wu-Hsieh BA. Modeling the Early Events of Severe Acute Respiratory Syndrome Coronavirus Infection In Vitro. J Virol (2006) 80(6):2684–93. doi: 10.1128/JVI.80.6.2684-2693.2006
320. Law HKW, Cheung CY, Ng HY, Sia SF, Chan YO, Luk W, et al. Chemokine Up-Regulation in SARS-Coronavirus–Infected, Monocyte-Derived Human Dendritic Cells. Blood (2005) 106(7):2366–74. doi: 10.1182/blood-2004-10-4166
321. Hu B, Huang S, Yin L. The Cytokine Storm and COVID-19. J Med Virol (2021) 93(1):250–6. doi: 10.1002/jmv.26232
322. Tang Y, Liu J, Zhang D, Xu Z, Ji J, Wen C. Cytokine Storm in COVID-19: The Current Evidence and Treatment Strategies. Front Immunol (2020) 11:1708(1708). doi: 10.3389/fimmu.2020.01708
323. Teijaro JR, Walsh KB, Rice S, Rosen H, Oldstone MBA. Mapping the Innate Signaling Cascade Essential for Cytokine Storm During Influenza Virus Infection. Proc Natl Acad Sci (2014) 111(10):3799–804. doi: 10.1073/pnas.1400593111
324. Moratto D, Giacomelli M, Chiarini M, Savarè L, Saccani B, Motta M, et al. Immune Response in Children With COVID-19 is Characterized by Lower Levels of T-Cell Activation Than Infected Adults. Eur J Immunol (2020) 50(9):1412–4. doi: 10.1002/eji.202048724
325. Yu T-M, Palanisamy K, Sun K-T, Day Y-J, Shu K-H, Wang IK, et al. RANTES Mediates Kidney Ischemia Reperfusion Injury Through a Possible Role of HIF-1α and LncRNA PRINS. Sci Rep (2016) 6(1):18424. doi: 10.1038/srep18424
326. Leisching G, Ali A, Cole V, Baker B. 2′-5′-Oligoadenylate Synthetase-Like Protein Inhibits Intracellular M. Tuberculosis Replication and Promotes Proinflammatory Cytokine Secretion. Mol Immunol (2020) 118:73–8. doi: 10.1016/j.molimm.2019.12.004
327. Gao X, Liu Y, Zou S, Liu P, Zhao J, Yang C, et al. Genome-Wide Screening of SARS-CoV-2 Infection-Related Genes Based on the Blood Leukocytes Sequencing Data Set of Patients With COVID-19. J Med Virol (2021) 93(9):5544–54. doi: 10.1002/jmv.27093
328. Ramakrishnan V. Ribosome Structure and the Mechanism of Translation. Cell (2002) 108(4):557–72. doi: 10.1016/S0092-8674(02)00619-0
329. Yang B, Fan J, Huang J, Guo E, Fu Y, Liu S, et al. Clinical and Molecular Characteristics of COVID-19 Patients With Persistent SARS-CoV-2 Infection. Nat Commun (2021) 12(1):3501. doi: 10.1038/s41467-021-23621-y
330. Lapointe CP, Grosely R, Johnson AG, Wang J, Fernández IS, Puglisi JD. Dynamic Competition Between SARS-CoV-2 NSP1 and mRNA on the Human Ribosome Inhibits Translation Initiation. Proc Natl Acad Sci (2021) 118(6):e2017715118. doi: 10.1073/pnas.2017715118
331. Thoms M, Buschauer R, Ameismeier M, Koepke L, Denk T, Hirschenberger M, et al. Structural Basis for Translational Shutdown and Immune Evasion by the Nsp1 Protein of SARS-CoV-2. Science (2020) 369(6508):1249–55. doi: 10.1126/science.abc8665
332. Schubert K, Karousis ED, Jomaa A, Scaiola A, Echeverria B, Gurzeler L-A, et al. SARS-CoV-2 Nsp1 Binds the Ribosomal mRNA Channel to Inhibit Translation. Nat Struct Mol Biol (2020) 27(10):959–66. doi: 10.1038/s41594-020-0511-8
333. Li Z, Yang L. Underlying Mechanisms and Candidate Drugs for COVID-19 Based on the Connectivity Map Database. Front Genet (2020) 11:558557(1168). doi: 10.3389/fgene.2020.558557
334. Battaglini D, Robba C, Ball L, Cruz FF, Silva PL, Pelosi P, et al. Emerging Therapies for COVID-19 Pneumonia. Expert Opin Invest Drugs (2020) 29(7):633–7. doi: 10.1080/13543784.2020.1771694
335. Erlanson DA. Many Small Steps Towards a COVID-19 Drug. Nat Commun (2020) 11(1):5048. doi: 10.1038/s41467-020-18710-3
336. Ramirez Alvarez C, Kee C, Sharma AK, Thomas L, Schmidt FI, Stanifer ML, et al. The Endogenous Cellular Protease Inhibitor SPINT2 Controls SARS-CoV-2 Viral Infection and is Associated to Disease Severity. PloS Pathog (2021) 17(6):e1009687. doi: 10.1371/journal.ppat.1009687
337. Alfi O, Yakirevitch A, Wald O, Wandel O, Izhar U, Oiknine-Djian E, et al. Human Nasal and Lung Tissues Infected Ex Vivo With SARS-CoV-2 Provide Insights Into Differential Tissue-Specific and Virus-Specific Innate Immune Responses in the Upper and Lower Respiratory Tract. J Virol (2021) 95(14):e00130–21. doi: 10.1128/JVI.00130-21
338. Kumar N, Mishra B, Mehmood A, Mohammad A, Mukhtar MS. Integrative Network Biology Framework Elucidates Molecular Mechanisms of SARS-CoV-2 Pathogenesis. iScience (2020) 23(9):101526. doi: 10.1016/j.isci.2020.101526
339. Jha PK, Vijay A, Halu A, Uchida S, Aikawa M. Gene Expression Profiling Reveals the Shared and Distinct Transcriptional Signatures in Human Lung Epithelial Cells Infected With SARS-CoV-2, MERS-CoV, or SARS-CoV: Potential Implications in Cardiovascular Complications of COVID-19. Front Cardiovasc Med (2021) 7:623012(349). doi: 10.3389/fcvm.2020.623012
340. Wyler E, Mösbauer K, Franke V, Diag A, Gottula LT, Arsiè R, et al. Transcriptomic Profiling of SARS-CoV-2 Infected Human Cell Lines Identifies HSP90 as Target for COVID-19 Therapy. iScience (2021) 24(3):102151. doi: 10.1016/j.isci.2021.102151
341. Ma D, Liu S, Hu L, He Q, Shi W, Yan D, et al. Single-Cell RNA Sequencing Identify SDCBP in ACE2-Positive Bronchial Epithelial Cells Negatively Correlates With COVID-19 Severity. J Cell Mol Med (2021) 25(14):7001–12. doi: 10.1111/jcmm.16714
342. Lee J-S, Han D, Kim SY, Hong KH, Jang M-J, Kim MJ, et al. Longitudinal Proteomic Profiling Provides Insights Into Host Response and Proteome Dynamics in COVID-19 Progression. Proteomics (2021) 21(11-12):2000278. doi: 10.1002/pmic.202000278
343. Lee AC, Castaneda G, Li WT, Chen C, Shende N, Chakladar J, et al. COVID-19 Severity Potentially Modulated by Cardiovascular-Disease-Associated Immune Dysregulation. Viruses (2021) 13(6):1018. doi: 10.3390/v13061018
344. Wicik Z, Eyileten C, Jakubik D, Simões SN, Martins DC, Pavão R, et al. ACE2 Interaction Networks in COVID-19: A Physiological Framework for Prediction of Outcome in Patients With Cardiovascular Risk Factors. J Clin Med (2020) 9(11):3743. doi: 10.3390/jcm9113743
345. Suvarna K, Biswas D, Pai MGJ, Acharjee A, Bankar R, Palanivel V, et al. Proteomics and Machine Learning Approaches Reveal a Set of Prognostic Markers for COVID-19 Severity With Drug Repurposing Potential. Front Physiol (2021) 12:652799(432). doi: 10.3389/fphys.2021.652799
346. Li Y, Li H, Zhou L. EZH2-Mediated H3K27me3 Inhibits ACE2 Expression. Biochem Biophys Res Commun (2020) 526(4):947–52. doi: 10.1016/j.bbrc.2020.04.010
347. Prasad K, AlOmar SY, Alqahtani SAM, Malik MZ, Kumar V. Brain Disease Network Analysis to Elucidate the Neurological Manifestations of COVID-19. Mol Neurobiol (2021) 58(5):1875–93. doi: 10.1007/s12035-020-02266-w
348. Sun J, Fahmi NA, Nassereddeen H, Cheng S, Martinez I, Fan D, et al. Computational Methods to Study Human Transcript Variants in COVID-19 Infected Lung Cancer Cells. Int J Mol Sci (2021) 22(18):9684. doi: 10.3390/ijms22189684
349. Tavakolpour S, Rakhshandehroo T, Wei EX, Rashidian M. Lymphopenia During the COVID-19 Infection: What it Shows and What can be Learned. Immunol Lett (2020) 225:31–2. doi: 10.1016/j.imlet.2020.06.013
350. Ouyang Y, Yin J, Wang W, Shi H, Shi Y, Xu B, et al. Downregulated Gene Expression Spectrum and Immune Responses Changed During the Disease Progression in Patients With COVID-19. Clin Infect Dis (2020) 71(16):2052–60. doi: 10.1093/cid/ciaa462
351. Narożna M, Rubiś B. Anti-SARS-CoV-2 Strategies and the Potential Role of miRNA in the Assessment of COVID-19 Morbidity, Recurrence, and Therapy. Int J Mol Sci (2021) 22(16):8663. doi: 10.3390/ijms22168663
352. Guzzi PH, Mercatelli D, Ceraolo C, Giorgi FM. Master Regulator Analysis of the SARS-CoV-2/Human Interactome. J Clin Med (2020) 9(4):982. doi: 10.3390/jcm9040982
353. Hussman JP. Severe Clinical Worsening in COVID-19 and Potential Mechanisms of Immune-Enhanced Disease. Front Med (2021) 8:637642(949). doi: 10.3389/fmed.2021.637642
354. Huang L, Shi Y, Gong B, Jiang L, Zhang Z, Liu X, et al. Dynamic Blood Single-Cell Immune Responses in Patients With COVID-19. Signal Transduction Targeted Ther (2021) 6(1):110. doi: 10.1038/s41392-021-00526-2
355. Ferrarini MG, Lal A, Rebollo R, Gruber AJ, Guarracino A, Gonzalez IM, et al. Genome-Wide Bioinformatic Analyses Predict Key Host and Viral Factors in SARS-CoV-2 Pathogenesis. Commun Biol (2021) 4(1):590. doi: 10.1038/s42003-021-02095-0
356. Schmidt N, Lareau CA, Keshishian H, Ganskih S, Schneider C, Hennig T, et al. The SARS-CoV-2 RNA–protein Interactome in Infected Human Cells. Nat Microbiol (2021) 6(3):339–53. doi: 10.1038/s41564-020-00846-z
357. Li R, Li Y, Liang X, Yang L, Su M, Lai KP. Network Pharmacology and Bioinformatics Analyses Identify Intersection Genes of Niacin and COVID-19 as Potential Therapeutic Targets. Briefings Bioinf (2020) 22(2):1279–90. doi: 10.1093/bib/bbaa300
358. Sałkowska A, Karwaciak I, Karaś K, Dastych J, Ratajewski M. SARS-CoV-2 Proteins Induce IFNG in Th1 Lymphocytes Generated From CD4+ Cells From Healthy, Unexposed Polish Donors. Vaccines (2020) 8(4):673. doi: 10.3390/vaccines8040673
359. Kato K, Ikliptikawati DK, Kobayashi A, Kondo H, Lim K, Hazawa M, et al. Overexpression of SARS-CoV-2 Protein ORF6 Dislocates RAE1 and NUP98 From the Nuclear Pore Complex. Biochem Biophys Res Commun (2021) 536:59–66. doi: 10.1016/j.bbrc.2020.11.115
360. Singh K, Chen Y-C, Hassanzadeh S, Han K, Judy JT, Seifuddin F, et al. Network Analysis and Transcriptome Profiling Identify Autophagic and Mitochondrial Dysfunctions in SARS-CoV-2 Infection. Front Genet (2021) 12:599261(289). doi: 10.3389/fgene.2021.599261
361. Cao W, Feng Q, Wang X. Computational Analysis of TMPRSS2 Expression in Normal and SARS-CoV-2-Infected Human Tissues. Chem-Bio Interact (2021) 346:109583. doi: 10.1016/j.cbi.2021.109583
362. García-Pérez BE, González-Rojas JA, Salazar MI, Torres-Torres C, Castrejón-Jiménez NS. Taming the Autophagy as a Strategy for Treating COVID-19. Cells (2020) 9(12):2679. doi: 10.3390/cells9122679
363. Yang N, Shen H-M. Targeting the Endocytic Pathway and Autophagy Process as a Novel Therapeutic Strategy in COVID-19. Int J Biol Sci (2020) 16(10):1724–31. doi: 10.7150/ijbs.45498
364. Qu Y, Wang X, Zhu Y, Wang W, Wang Y, Hu G, et al. ORF3a-Mediated Incomplete Autophagy Facilitates Severe Acute Respiratory Syndrome Coronavirus-2 Replication. Front Cell Dev Biol (2021) 9:716208(2012). doi: 10.3389/fcell.2021.716208
365. Koepke L, Hirschenberger M, Hayn M, Kirchhoff F, Sparrer KMJ. Manipulation of Autophagy by SARS-CoV-2 Proteins. Autophagy (2021) 17(9):2659–61. doi: 10.1080/15548627.2021.1953847
366. Shroff A, Nazarko TY. The Molecular Interplay Between Human Coronaviruses and Autophagy. Cells (2021) 10(8):2022. doi: 10.3390/cells10082022
367. Maity S, Saha A. Therapeutic Potential of Exploiting Autophagy Cascade Against Coronavirus Infection. Front Microbiol (2021) 12:675419(1173). doi: 10.3389/fmicb.2021.675419
368. Zhu H, Chen CZ, Sakamuru S, Zhao J, Ngan DK, Simeonov A, et al. Mining of High Throughput Screening Database Reveals AP-1 and Autophagy Pathways as Potential Targets for COVID-19 Therapeutics. Sci Rep (2021) 11(1):6725. doi: 10.1038/s41598-021-86110-8
369. Sharma P, McAlinden KD, Ghavami S, Deshpande DA. Chloroquine: Autophagy Inhibitor, Antimalarial, Bitter Taste Receptor Agonist in Fight Against COVID-19, a Reality Check? Eur J Pharmacol (2021) 897:173928. doi: 10.1016/j.ejphar.2021.173928
370. Bello-Perez M, Sola I, Novoa B, Klionsky DJ, Falco A. Canonical and Noncanonical Autophagy as Potential Targets for COVID-19. Cells (2020) 9(7):1619. doi: 10.3390/cells9071619
371. Bonam SR, Muller S, Bayry J, Klionsky DJ. Autophagy as an Emerging Target for COVID-19: Lessons From an Old Friend, Chloroquine. Autophagy (2020) 16(12):2260–6. doi: 10.1080/15548627.2020.1779467
372. Miller K, McGrath ME, Hu Z, Ariannejad S, Weston S, Frieman M, et al. Coronavirus Interactions With the Cellular Autophagy Machinery. Autophagy (2020) 16(12):2131–9. doi: 10.1080/15548627.2020.1817280
373. Vomero M, Barbati C, Colasanti T, Celia AI, Speziali M, Ucci FM, et al. Autophagy Modulation in Lymphocytes From COVID-19 Patients: New Therapeutic Target in SARS-COV-2 Infection. Front Pharmacol (2020) 11:569849(1759). doi: 10.3389/fphar.2020.569849
374. Mosleh G, Badr P, Zaeri M, Mohagheghzadeh A. Potentials of Antitussive Traditional Persian Functional Foods for COVID-19 Therapy†. Front Pharmacol (2021) 12:624006(1654). doi: 10.3389/fphar.2021.624006
375. Taylor EW, Radding W. Understanding Selenium and Glutathione as Antiviral Factors in COVID-19: Does the Viral Mpro Protease Target Host Selenoproteins and Glutathione Synthesis? Front Nutr (2020) 7:143(143). doi: 10.3389/fnut.2020.00143
376. Pinto BGG, Oliveira AER, Singh Y, Jimenez L, Gonçalves ANA, Ogava RLT, et al. ACE2 Expression is Increased in the Lungs of Patients With Comorbidities Associated With Severe COVID-19. J Infect Dis (2020) 222(4):556–63. doi: 10.1093/infdis/jiaa332
377. Keikha R, Hashemi-Shahri SM, Jebali A. The Relative Expression of miR-31, miR-29, miR-126, and miR-17 and Their mRNA Targets in the Serum of COVID-19 Patients With Different Grades During Hospitalization. Eur J Med Res (2021) 26(1):75. doi: 10.1186/s40001-021-00544-4
378. Luo H, Liu D, Liu W, Wang G, Chen L, Cao Y, et al. Germline Variants in UNC13D and AP3B1 are Enriched in COVID-19 Patients Experiencing Severe Cytokine Storms. Eur J Hum Genet (2021) 29(8):1312–5. doi: 10.1038/s41431-021-00886-x
379. Gebhard C, Regitz-Zagrosek V, Neuhauser HK, Morgan R, Klein SL. Impact of Sex and Gender on COVID-19 Outcomes in Europe. Biol Sex Dif (2020) 11(1):29. doi: 10.1186/s13293-020-00304-9
380. Didangelos A, Rosenberg HF. COVID-19 Hyperinflammation: What About Neutrophils? mSphere (2020) 5(3):e00367–20. doi: 10.1128/mSphere.00367-20
381. Pushparaj PN, Abdulkareem AA, Naseer MI. Identification of Novel Gene Signatures Using Next-Generation Sequencing Data From COVID-19 Infection Models: Focus on Neuro-COVID and Potential Therapeutics. Front Pharmacol (2021) 12:688227(1948). doi: 10.3389/fphar.2021.688227
382. Torretta E, Garziano M, Poliseno M, Capitanio D, Biasin M, Santantonio TA, et al. Severity of COVID-19 Patients Predicted by Serum Sphingolipids Signature. Int J Mol Sci (2021) 22(19):10198. doi: 10.3390/ijms221910198
383. Siri M, Dastghaib S, Zamani M, Rahmani-Kukia N, Geraylow KR, Fakher S, et al. Autophagy, Unfolded Protein Response, and Neuropilin-1 Cross-Talk in SARS-CoV-2 Infection: What can be Learned From Other Coronaviruses. Int J Mol Sci (2021) 22(11):5992. doi: 10.3390/ijms22115992
384. Das AB. Lung Disease Network Reveals Impact of Comorbidity on SARS-CoV-2 Infection and Opportunities of Drug Repurposing. BMC Med Genomics (2021) 14(1):226. doi: 10.1186/s12920-021-01079-7
385. Nain Z, Barman SK, Sheam MM, Syed SB, Samad A, Quinn JMW, et al. Transcriptomic Studies Revealed Pathophysiological Impact of COVID-19 to Predominant Health Conditions. Briefings Bioinf (2021). doi: 10.1093/bib/bbab197
386. Xia S, Zhong Z, Gao B, Vong CT, Lin X, Cai J, et al. The Important Herbal Pair for the Treatment of COVID-19 and its Possible Mechanisms. Chin Med (2021) 16(1):25. doi: 10.1186/s13020-021-00427-0
387. Wang H, Zhang J, Lu Z, Dai W, Ma C, Xiang Y, et al. Identification of Potential Therapeutic Targets and Mechanisms of COVID-19 Through Network Analysis and Screening of Chemicals and Herbal Ingredients. Briefings Bioinf (2021). doi: 10.1093/bib/bbab373
388. Gao K, Song Y-P, Song A. Exploring Active Ingredients and Function Mechanisms of Ephedra-Bitter Almond for Prevention and Treatment of Corona Virus Disease 2019 (COVID-19) Based on Network Pharmacology. BioData Min (2020) 13(1):19. doi: 10.1186/s13040-020-00229-4
389. Dai Y-J, Wan S-Y, Gong S-S, Liu J-C, Li F, Kou J-P. Recent Advances of Traditional Chinese Medicine on the Prevention and Treatment of COVID-19. Chin J Nat Med (2020) 18(12):881–9. doi: 10.1016/S1875-5364(20)60031-0
390. Li R, Guo C, Li Y, Qin Z, Huang W. Therapeutic Targets and Signaling Mechanisms of Vitamin C Activity Against Sepsis: A Bioinformatics Study. Briefings Bioinf (2020) 22(3):bbaa079. doi: 10.1093/bib/bbaa079
391. Gomez Marti JL, Wells A, Brufsky AM. Dysregulation of the Mevalonate Pathway During SARS-CoV-2 Infection: An in Silico Study. J Med Virol (2021) 93(4):2396–405. doi: 10.1002/jmv.26743
392. Mu J, Fang Y, Yang Q, Shu T, Wang A, Huang M, et al. SARS-CoV-2 N Protein Antagonizes Type I Interferon Signaling by Suppressing Phosphorylation and Nuclear Translocation of STAT1 and STAT2. Cell Discov (2020) 6(1):65. doi: 10.1038/s41421-020-00208-3
393. Jiang H-W, Zhang H-N, Meng Q-F, Xie J, Li Y, Chen H, et al. SARS-CoV-2 Orf9b Suppresses Type I Interferon Responses by Targeting TOM70. Cell Mol Immunol (2020) 17(9):998–1000. doi: 10.1038/s41423-020-0514-8
394. Xia H, Cao Z, Xie X, Zhang X, Chen JY-C, Wang H, et al. Evasion of Type I Interferon by SARS-CoV-2. Cell Rep (2020) 33(1):108234. doi: 10.1016/j.celrep.2020.108234
395. Acharya D, Liu G, Gack MU. Dysregulation of Type I Interferon Responses in COVID-19. Nat Rev Immunol (2020) 20(7):397–8. doi: 10.1038/s41577-020-0346-x
396. Blanco-Melo D, Nilsson-Payant BE, Liu W-C, Uhl S, Hoagland D, Møller R, et al. Imbalanced Host Response to SARS-CoV-2 Drives Development of COVID-19. Cell (2020) 181(5):1036–45.e9. doi: 10.1016/j.cell.2020.04.026
397. Sallard E, Lescure F-X, Yazdanpanah Y, Mentre F, Peiffer-Smadja N. Type 1 Interferons as a Potential Treatment Against COVID-19. Antiviral Res (2020) 178:104791. doi: 10.1016/j.antiviral.2020.104791
398. Nayak T, De D, Dhal PK. The Differences in SARS-CoV and SARS-CoV-2 Specific Co-Expression Network Mediated Biological Process in Human Gut Enterocytes. Infect Genet Evol (2021) 92:104892. doi: 10.1016/j.meegid.2021.104892
399. Ong EZ, Kalimuddin S, Chia WC, Ooi SH, Koh CWT, Tan HC, et al. Temporal Dynamics of the Host Molecular Responses Underlying Severe COVID-19 Progression and Disease Resolution. EBioMedicine (2021) 65:103262. doi: 10.1016/j.ebiom.2021.103262
400. Chirumbolo S, Valdenassi L, Simonetti V, Bertossi D, Ricevuti G, Franzini M, et al. Insights on the Mechanisms of Action of Ozone in the Medical Therapy Against COVID-19. Int Immunopharmacol (2021) 96:107777. doi: 10.1016/j.intimp.2021.107777
401. Emanuele S, Celesia A, D’Anneo A, Lauricella M, Carlisi D, De Blasio A, et al. The Good and Bad of Nrf2: An Update in Cancer and New Perspectives in COVID-19. Int J Mol Sci (2021) 22(15):7963. doi: 10.3390/ijms22157963
402. Liao M-T, Wu C-C, Wu S-FV, Lee M-C, Hu W-C, Tsai K-W, et al. Resveratrol as an Adjunctive Therapy for Excessive Oxidative Stress in Aging COVID-19 Patients. Antioxidants (2021) 10(9):1440. doi: 10.3390/antiox10091440
403. Keikha R, Hashemi-Shahri SM, Jebali A. The miRNA Neuroinflammatory Biomarkers in COVID-19 Patients With Different Severity of Illness. Neurología (2021). doi: 10.1016/j.nrl.2021.06.005
404. Cava C, Bertoli G, Castiglioni I. A Protein Interaction Map Identifies Existing Drugs Targeting SARS-CoV-2. BMC Pharmacol Toxicol (2020) 21(1):65. doi: 10.1186/s40360-020-00444-z
405. Liao M, Liu Y, Yuan J, Wen Y, Xu G, Zhao J, et al. Single-Cell Landscape of Bronchoalveolar Immune Cells in Patients With COVID-19. Nat Med (2020) 26(6):842–4. doi: 10.1038/s41591-020-0901-9
406. Tan Y, Zhang W, Zhu Z, Qiao N, Ling Y, Guo M, et al. Integrating Longitudinal Clinical Laboratory Tests With Targeted Proteomic and Transcriptomic Analyses Reveal the Landscape of Host Responses in COVID-19. Cell Discov (2021) 7(1):42. doi: 10.1038/s41421-021-00274-1
407. Palacios Y, Ruiz A, Ramón-Luing LA, Ocaña-Guzman R, Barreto-Rodriguez O, Sánchez-Monciváis A, et al. Severe COVID-19 Patients Show an Increase in Soluble TNFR1 and ADAM17, With a Relationship to Mortality. Int J Mol Sci (2021) 22(16):8423. doi: 10.3390/ijms22168423
408. Chen H, Liu W, Wang Y, Liu D, Zhao L, Yu J. SARS-CoV-2 Activates Lung Epithelial Cell Proinflammatory Signaling and Leads to Immune Dysregulation in COVID-19 Patients. EBioMedicine (2021) 70:103500. doi: 10.1016/j.ebiom.2021.103500
409. Ahmed SSSJ, Paramasivam P, Kamath M, Sharma A, Rome S, Murugesan R. Genetic Exchange of Lung-Derived Exosome to Brain Causing Neuronal Changes on COVID-19 Infection. Mol Neurobiol (2021). doi: 10.1007/s12035-021-02485-9
410. Ayeh SK, Abbey EJ, Khalifa BAA, Nudotor RD, Osei AD, Chidambaram V, et al. Statins Use and COVID-19 Outcomes in Hospitalized Patients. PloS One (2021) 16(9):e0256899. doi: 10.1371/journal.pone.0256899
411. Domdom M-A, Brest P, Grosjean I, Roméo B, Landi MT, Gal J, et al. A Multifactorial Score Including Autophagy for Prognosis and Care of COVID-19 Patients. Autophagy (2020) 16(12):2276–81. doi: 10.1080/15548627.2020.1844433
412. Kucia M, Ratajczak J, Bujko K, Adamiak M, Ciechanowicz A, Chumak V, et al. An Evidence That SARS-Cov-2/COVID-19 Spike Protein (SP) Damages Hematopoietic Stem/Progenitor Cells in the Mechanism of Pyroptosis in Nlrp3 Inflammasome-Dependent Manner. Leukemia (2021) 35(10):3026–9. doi: 10.1038/s41375-021-01332-z
413. Zhao H, Zhang Q, Chen H, Rahman MR, Faruquee HM. Integrated Multi-Omics Approach Identified Molecular Mechanism and Pathogenetic Processes of COVID-19 That Affect Patient With Parkinson’s Disorder. Saudi J Biol Sci (2021). doi: 10.1016/j.sjbs.2021.07.074
414. Abers MS, Delmonte OM, Ricotta EE, Fintzi J, Fink DL, de Jesus AAA, et al. An Immune-Based Biomarker Signature is Associated With Mortality in COVID-19 Patients. JCI Insight (2021) 6(1):e144455. doi: 10.1172/jci.insight.144455
415. Kong Q, Wu Y, Gu Y, Lv Q, Qi F, Gong S, et al. Analysis of the Molecular Mechanism of Pudilan (PDL) Treatment for COVID-19 by Network Pharmacology Tools. Biomed Pharmacother (2020) 128:110316. doi: 10.1016/j.biopha.2020.110316
416. Liu F, Li Y, Yang Y, Li M, Du Y, Zhang Y, et al. Study on Mechanism of Matrine in Treatment of COVID-19 Combined With Liver Injury by Network Pharmacology and Molecular Docking Technology. Drug Deliv (2021) 28(1):325–42. doi: 10.1080/10717544.2021.1879313
417. Redwan EM, Alghamdi MF, El-Aziz TMA, Adadi P, Aljabali AAA, Attrish D, et al. The Mechanism Behind Flaring/Triggering of Autoimmunity Disorders Associated With COVID-19. Autoimmun Rev (2021) 20(10):102909. doi: 10.1016/j.autrev.2021.102909
418. Lin H, Wang X, Liu M, Huang M, Shen Z, Feng J, et al. Exploring the Treatment of COVID-19 With Yinqiao Powder Based on Network Pharmacology. Phytother Res (2021) 35(5):2651–64. doi: 10.1002/ptr.7012
419. Jia S, Luo H, Liu X, Fan X, Huang Z, Lu S, et al. Dissecting the Novel Mechanism of Reduning Injection in Treating Coronavirus Disease 2019 (COVID-19) Based on Network Pharmacology and Experimental Verification. J Ethnopharmacol (2021) 273:113871. doi: 10.1016/j.jep.2021.113871
420. Wang J, Ge W, Peng X, Yuan L, He S, Fu X. Investigating the Active Compounds and Mechanism of HuaShi XuanFei Formula for Prevention and Treatment of COVID-19 Based on Network Pharmacology and Molecular Docking Analysis. Mol Divers (2021) 9:1–16. doi: 10.1007/s11030-021-10244-0
Keywords: systems biology, systems immunology, WGCNA, hub-high traffic genes, immunopathogenesis, therapeutic targets in infectious diseases, COVID-19 pandemic
Citation: Hasankhani A, Bahrami A, Sheybani N, Aria B, Hemati B, Fatehi F, Ghaem Maghami Farahani H, Javanmard G, Rezaee M, Kastelic JP and Barkema HW (2021) Differential Co-Expression Network Analysis Reveals Key Hub-High Traffic Genes as Potential Therapeutic Targets for COVID-19 Pandemic. Front. Immunol. 12:789317. doi: 10.3389/fimmu.2021.789317
Received: 04 October 2021; Accepted: 26 November 2021;
Published: 15 December 2021.
Edited by:
Otavio Cabral-Marques, University of São Paulo, BrazilReviewed by:
Lasse Melvær Giil, Haraldsplass Deaconal Hospital (HDS), NorwayLucia Elena Alvarado-Arnez, Franz Tamayo University, Bolivia
Thiago Dominguez Crespo Hirata, University of São Paulo, Brazil
Copyright © 2021 Hasankhani, Bahrami, Sheybani, Aria, Hemati, Fatehi, Ghaem Maghami Farahani, Javanmard, Rezaee, Kastelic and Barkema. This is an open-access article distributed under the terms of the Creative Commons Attribution License (CC BY). The use, distribution or reproduction in other forums is permitted, provided the original author(s) and the copyright owner(s) are credited and that the original publication in this journal is cited, in accordance with accepted academic practice. No use, distribution or reproduction is permitted which does not comply with these terms.
*Correspondence: Aliakbar Hasankhani, A.hasankhani74@ut.ac.ir; Abolfazl Bahrami, A.Bahrami@ut.ac.ir
†These authors have contributed equally to this work