Energy Expenditure in People with Diabetes Mellitus: A Review
- Laboratoire IRISSE, UFR des Sciences de l’Homme et de l’Environnement, Université de la Réunion, Le Tampon, La Réunion, France
Physical activity (PA) is an important non-therapeutic tool in primary prevention and treatment of diabetes mellitus (DM). To improve activity-based health management, patients need to quantify activity-related energy expenditure and the other components of total daily energy expenditure. This review explores differences between the components of total energy expenditure in patients with DM and healthy people and presents various tools for assessing the energy expenditure in subjects with DM. From this review, it appears that patients with uncontrolled DM have a higher basal energy expenditure (BEE) than healthy people which must be considered in the establishment of new BEE estimate equations. Moreover, studies showed a lower activity energy expenditure in patients with DM than in healthy ones. This difference may be partially explained by patient with DMs poor compliance with exercise recommendations and their greater participation in lower intensity activities. These specificities of PA need to be taken into account in the development of adapted tools to assess activity energy expenditure and daily energy expenditure in people with DM. Few estimation tools are tested in subjects with DM and this results in a lack of accuracy especially for their particular patterns of activity. Thus, future studies should examine sensors coupling different technologies or method that is specifically designed to accurately assess energy expenditure in patients with diabetes in daily life.
Introduction
In recent decades, a decrease in leisure activity followed by a rise in sedentary behaviors and the degradation of eating habits have been observed. These changes have led to an increasing risk of developing metabolic diseases such as diabetes mellitus (DM) (1–3). In 2004, Wild et al. (4) estimated that 4.4% of the population worldwide will have diabetes in 2030 (representing 366 million people). Only 7 years later, Whiting et al. (5) assessed that 366 million people had diabetes and projected an increase to 552 million people by 2030. Various solutions exist to combat this increasing prevalence (6). Physical activity (PA) has been shown to be the main factor in primary prevention. Accordingly, studies show a decrease of 15–67% in the relative risk of developing DM (7–11). When associated with a healthy diet, PA is also considered to be an imperative component in the treatment of subjects with DM (12). Indeed, in the short term, appropriate exercise can decrease glycemia by burning the overflow of blood glucose to generate energy. Long term, PA can improve insulin sensitivity, glycemic control, systolic blood pressure, and weight loss. It can also increase , reduce glycated hemoglobin and fasting plasma glucose, and finally, decrease the need for oral medications or insulin (13–18). All these improvements are considered sufficient to decrease the rate of diabetes complications (19) and the relative risk of all-cause mortality (20, 21). Nevertheless, due to the impaired glucose regulation, exercise duration and intensity must be considered attentively. Actually, long duration exercise without sugar intake may increase the risk of hypoglycemia. On the contrary, brief intense exercise may induce hyperglycemia requiring insulin intake. Underestimated energy expenditure may also lead to an underestimation of necessary medication. In these cases, exercise as a therapeutic tool must be precisely programed into daily life in order to see benefits in patients with DM.
The aim of this article is twofold. First, it presents the differences between the energy expenditure between of people with DM and healthy people. Second, it reports on the various methods for evaluating EE and their validity in subjects with DM.
Energy Expenditure in Subjects with DM
In the literature, a simple model defines the daily total energy expenditure (TEE) as the sum of the basal energy expenditure (BEE), the thermic effect of food (TEF), and the activity energy expenditure (AEE). BEE is the major component of internal heat produced. BEE, which is defined as the energy expended to maintain minimal metabolic activities during a non-active period, is the main component of TEE (60–70% of TEE) (22). The TEF symbolizes the energy used by the body when it processes certain unrefined foods as lean meats, vegetables, and whole grains. AEE represents the energy expended through PA and volitional exercise and sports. AEE is the main parameter that allows modulation of TEE since it depends on the type of PA, its duration, and its intensity. Thus, in the sections below, we will present the differences between the three EE components in patients with DM and healthy people.
BEE in Subjects with DM
Studies exploring BEE in patients with DM report no difference in absolute BEE (22–25). Nevertheless, this preliminary result could be explained by the sample heterogeneity of these studies. Indeed, after adjusting for age, sex, and fat free mass (FFM), it appeared that people with uncontrolled DM (HbA1c ≥ 8%) had a BEE 7.7% higher than healthy people (25–30).
Several possible physiological mechanisms may induce changes in BEE. FFM explains 65–90% of the interindividual variation of BEE in healthy adults (31). In subjects with DM, fasting blood glucose (FBG) may be another independent determinant of BEE (26–29). Indeed, studies found a 3–8% increase in BEE in patients with DM with high FBG (>10 mmol/l), which returns to the normal after insulin therapy (26, 29). These results are supported by the study of Ryan et al. (22) who reported no increase in the absolute and adjusted BEE in patients with a treated and stable DM. These results have been explained by two hypotheses. First, BEE may be increased by a rise in the loss of glucose in the urine (glycosuria) (29). It has been shown that hyperglycemia can increase glycosuria by 30–80 g/day, which corresponds to an energy loss of about 120–320 kcal/day (32). Finally, the second hypothesis indicated that people with impaired glucose regulation had an increase in fasting hepatic gluconeogenesis with the rise of glycemia (33). Gluconeogenesis is an important energy-consuming process that transforms free fatty acid into glucose causing by the decrease of insulin plasma level.
Overall, these studies suggest that the increase in glycosuria and/or gluconeogenesis caused by hyperglycemia seems to be behind the high BEE observed in patients with DM with poor glycemic control.
Thermic Effect of Food
Studies examining the effects of DM on the TEF estimated a lower TEF in patients with insulin resistance than in healthy people (23, 34). Another study assessing insulin-induced thermogenesis (defined as the percentage of increase in metabolic rate during an insulin/glucose infusion) showed a decrease from subjects without DM (11.7%) to patients with impaired glucose tolerance (7.3%) and finally patients with DM (6.5%) (35). Thus, it appears that TEF decreases progressively with the development of DM. Indeed, TEF is negatively correlated with FBG and insulin concentrations (both are predictors of insulin resistance). The decrease in TEF may be induced by a reduced rate of glycogenesis in the skeletal muscle (36) and an impaired activation of the sympathetic nervous system (37). As discussed later in this report, TEF will not be studied further because it does not represent an adjustable component of TEE.
Activity Energy Expenditure in Subjects with DM
A few studies directly compare the AEE in free-living conditions of patient with DM and a control group and indicate a lower AEE in patients with DM than in healthy people (694 vs 1,086 kcal/day, respectively) (24, 38). Taken together, the results of these studies suggest that people with DM present a total AEE less than that of healthy people. This result may be explained by either a lower amount of PA in subjects with DM and/or for a similar activity, by a difference in mechanical or metabolic efficiency.
Physical activity guidelines give the minimal amount of exercise required to maintain or to improve people’s health. These directives concern the general population as well as patients with DM. A first guideline recommends accumulating an AEE of at least 800 kcal/week or 600 MET.min/week to be sufficiently active (39). This recommendation conducted led to instructions for practicing PA at moderate intensity for a minimum of 30 min, 5 days/week or vigorous PA for a minimum of 20 min, 3 days/week, or accumulating 10,000 steps, daily or at least 5 days/week (40–42).
According to these guidelines, 31–54.6% of individuals with DM were considered inactive (no activity practiced) and 13–53.6% insufficiently active. In the general population, 5–62% over 45 years of age were defined as inactive and 9–43% insufficiently active. In addition, studies indicated a lower rate of patients with DM following the guidelines than in the general population (12.4–43% vs 55.5–87.2% in healthy people) (43–53). When the number of steps was observed, patients with DM without neuropathy walked 3,292–9,049 steps/day on average (54–59). Mitsui et al. (60) showed that 12–27.1% of healthy people aged over 45 years of age walk more than 10,000 steps/day while in Strycker et al. (56), no patients with DM followed this recommendation and only 15% walked 7,500–9,999 steps/day.
To further analyze the practice of PA, activities can be qualified by their type (leisure, recreational, domestic), by their intensity (light, moderate, vigorous), or by their duration. By questioning the activity preferences of 505 Mexican–American patients with DM, study reported that the most common activities were gardening (33.7%) and walking (31.8%) and the least frequent were jogging (2.4%), aerobic (2.3%), and swimming (1.3%) (44). Moreover, studies also reported that 33, 16, and 12% of patients with DM had at least one, two, and three or more regular activities in the month, respectively, and 71% achieved at least one 10-min period/day of moderate PA and 26% at least one 10-min period/day of vigorous PA (44, 61). By using a questionnaire, Kriska et al. (62) estimated a lower number of PA hours/week in men with DM than in healthy men (12.1 vs 22.9 h/week) and found no difference in women. By contrast, Ford and Herman (63) showed that patients with DM were equally likely to have engaged in PA. Based on indirect calorimetry (IC), studies noted a higher 24-h spontaneous PA (+10.5%) and total activity counts in healthy people and pre-patients with DM than in individuals with DM (25, 64). By contrast, Fontvieille et al. (23) concluded that there was no difference in spontaneous PA between the two groups.
The lower AEE observed in patients with DM may result from a smaller amount of PA accumulated throughout the day. However, other parameters such as metabolic and mechanical efficiency can also reduce energy expenditure. With equal level of activity, different AEE may reflect the different use of energy fuel. Indeed, the energetic equivalent of 1 l of oxygen changes according to the type of foodstuffs metabolized (in a simple model, 1 l of oxygen equals 5.05 and 4.48 kcal when carbohydrate and fat are oxidized, respectively). The respiratory quotient is an indicator of the fuel substrate used and it is equal to the divided by the (respiratory quotient = 1 for carbohydrate oxidation only and 0.70 for fat oxidation only). During moderate-intensity exercise (40–50% of ), studies found no significant difference between the respiratory quotient and of patients with DM and healthy people (65, 66). In a second hypothesis, mechanical efficiency may be another parameter explaining the difference in AEE between patient with DM and healthy people. For instance, walking is one of the most convenient daily PA recommended for increasing TEE. Studies of patients with DM showed a decrease in comfortable walking speed, cadence, and stride length and an increase of plantar pressure at the heel, mid-foot, and first metatarsophalangeal joint as compared with the control group (67, 68). Moreover, it was also demonstrated that patients with DM have a less total concentric work in lower limb than healthy people (69), but more cocontraction in the muscles at the ankle and knee joint during the stance period (70). This altered gait pattern observed in patients with DM seems to reflect a stabilization strategy to compensate for the development of peripheral neuropathy (diminished sensory information and maximum strength of the lower limbs). At speeds of walking range from 0.6 to 1.6 m/s, Petrovic et al. (66) presented a general pattern of a higher cost of walking (express as milliliters per kilogram per minute) in diabetic patients with neuropathy (significant difference at all speed of walking) and in diabetic patients without neuropathy (significant difference at 1.4 and 1.6 m/s) in comparison with healthy subjects. However, Maiolo et al. (71) found no significant difference in net EE of walking (at three different intensities) between patients with DM and healthy people.
In light of the previous results, the lower AEE in subjects with DM seems to be due to a low level of daily PA with more low intensity activities and an energetic inefficiency during walking. Due to the complex nature of PA, AEE assessment requires precise and adapted tools. In the remainder of our review, we will describe potential tools (validated for use in daily life) that are suitable for research with patients with DM.
Assessment of Energy Expenditure in Subjects with DM
Methods for Evaluating BEE in Subjects with DM
Measure of BEE is typically taken by IC. Although these methods are very accurate, they require significant human and financial resources. Thus, equations have been proposed to simply estimate the BEE with variables such as age, height, or weight. Many of these equations were constructed with data from the general population and then tested in people with DM (Table 1).
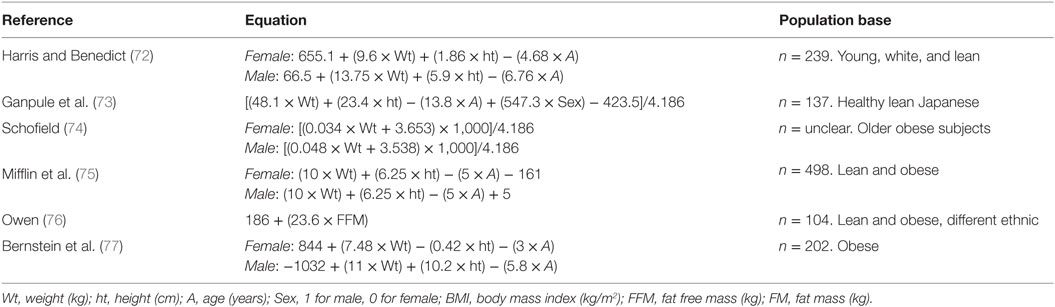
Table 1. Most popular equations to estimate basal energy expenditure (kcal/day) derived from the general population.
The Harris–Benedict equation is most frequently used to estimate BEE in the general population, but studies in patients with DM have presented mixed results. Many studies show a significant overestimation of BEE with this equation (RMSE1 = 160–184 kcal/day) (29, 78–80). Huang et al. (30) also found an overestimation of 3.3% in men with DM, but an underestimation of −3.1% in women. Finally, Miyake et al. (81) and de Figueiredo Ferreira et al. (82) observed no significant difference with IC (MD2 = −19 to 42.3 kcal/day). With the Mifflin–St Jeor equation, studies in patients with DM show an under/overestimation of BEE by −126 to 160 kcal/day (80, 81, 83), or no significant difference (29, 78). The Ganpule equation presented no difference with the reference measure (bias3 = 4.5%) (79) or underestimated BEE by −110 kcal/day (81). Those developed by Schofield and Rodrigues showed no significant difference with the reference to subjects with DM [MD = 55 kcal/day (p = 0.065) and −17.6 kcal/day (p = 0.845), respectively]. The other equations tested in diabetic population overestimated the BEE (RMSE = 155–209 kcal/day, bias = 5.9–12.3%) except for those of Owen and Bernstein, which underestimated BEE (MD = −62 to −53.5 kcal/day for the Owen equation and bias = −11.6 to −13.9% for the Bernstein equation) (79–81).
To improve the BEE estimate, other equations taking into account the physiological specificities of subjects with DM were developed (Table 2) and compared with equations established for the general population, and with IC (Table 3). The Huang and Martin equations presented comparable coefficients of determination (r2 = 0.75 and 0.79, respectively) and had better results as compared with the Harris–Benedict equation (30, 80). Besides, de Figueiredo Ferreira et al. (83) showed an overestimation of BEE (MD = 115 kcal/day) in women with DM with this equation. The Ikeda and Martin equations presented a lower RMSE (103 and 136 kcal/day, respectively) than the other general equations (140–209 kcal/day) (79, 80). Finally, the Gougeon equation, taking into account the glycemic status, presented no significant difference with IC [MD = 7.4 kcal/day (p = 0.845) and bias = −0.5 and 1.6%] (29, 83).
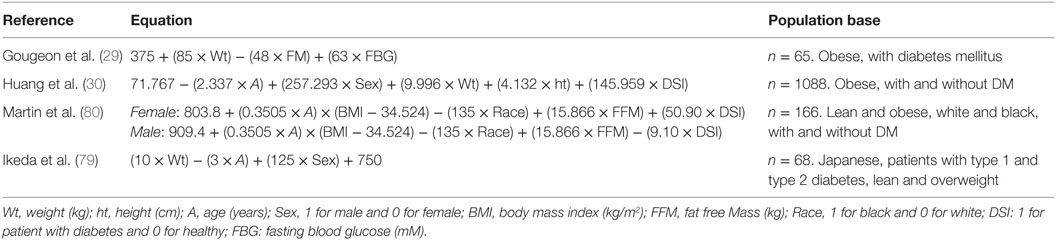
Table 2. Equations to estimate basal energy expenditure (kcal/day) derived from patient with diabetes or mixed population.
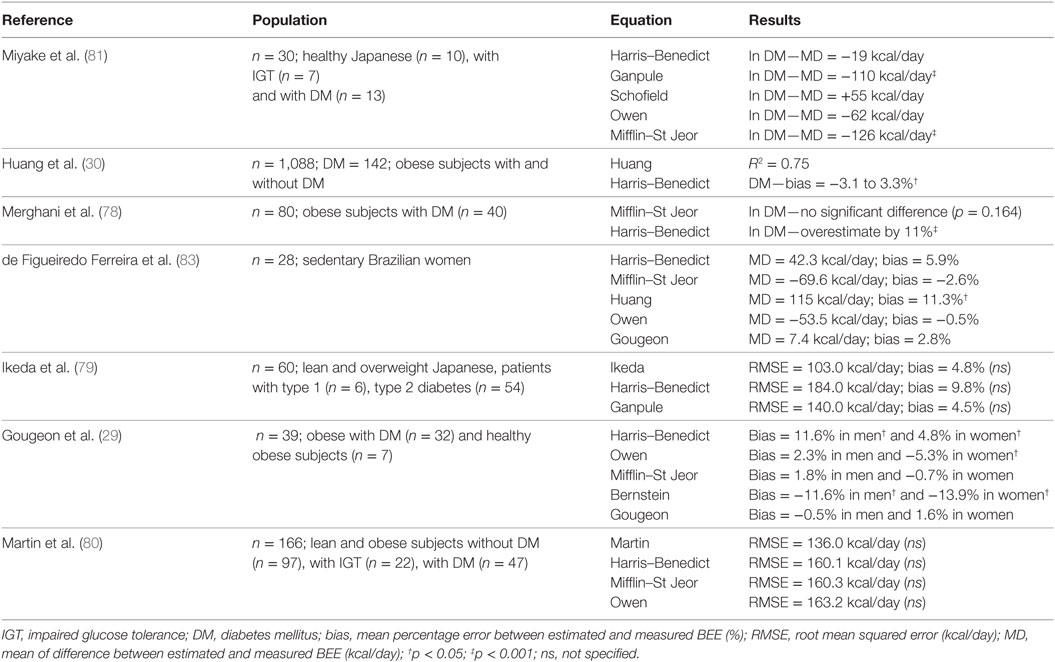
Table 3. References and results of studies comparing predictive basal energy expenditure with the gold standard in patients with type 2 diabetes.
If BEE in subjects with DM cannot be accurately assessed using direct or IC, it can be adequately estimated using equations. The results of the aforementioned studies suggest that BEE in subjects with DM is better determined by using specific and adapted equations. Thus, the best results were obtained with the Gougeon equation and this may be due to the inclusion of the FBG as a variable.
Evaluation of Free-Living Activity Energy Expenditure in Subjects with DM
Among the tools for assessing AEE, the doubly labeled water method and direct/IC are considered to be the gold standards. Although these different tools accurately estimated TEE and AEE, these methods cannot be used by a patient with DM to estimate his/her AEE and daily EE daily. For everyday use, many field evaluation tools for estimating AEE have been developed and tested in subjects with DM, such as diaries, questionnaires, or motion sensors. These methods and tools are often classified into two categories: subjective and objective methods.
Subjective Methods
Subjective methods include processes that usually require subjects to record their professional, home, and leisure activities. Among them, activity recall, logs, or questionnaires are declarative methods that provide a detailed account (nature, intensity, duration) of all daily PA. The AEE and TEE are then determined using the factorial method (84), where each activity intensity was weighted by its intensity expressed in Met, as it is referred to the compendium (85), then multiplied by its duration. As a reminder, one MET is equivalent to the BEE (1 MET = 3.5 ml/kg/min of oxygen consumption or 1 kcal/kg/h). At least, when added to the measured or predicted BEE, it is possible to predict the AEE and TEE with the sum of all activity estimates during the day. Thus, the use of questionnaires is widely reported in the literature on the topic because they are easy to use for large epidemiologic surveys. Nevertheless, few studies examined their validity with reference measures in subjects with DM.
The Modifiable Activity Questionnaire is a short survey created for the assessment of PA level (average hour/week of occupational and leisure activities over the past year) or energy expenditure in a variety of populations and age groups (86). Studies show that this questionnaire is both reliable and valid through direct comparisons with doubly labeled water (r = 0.75) (87) and accelerometer (r = 0.62) (86) in adults with DM. The International Physical Activity Questionnaire is most frequently used in epidemiologic research. It asks subjects about their PA during past week. Studies comparing the AEE estimated by this questionnaire with those estimated with accelerometer found a positive correlation in patients with DM with a correlation coefficient ranging from 0.24 (n = 143, p < 0.05) (88) to 0.53 (n = 31, p = 0.002) (89). The two questionnaires refer to the frequency and duration of activities and calculate an estimated AEE in kcal/week.
Subjective methods suffer from acknowledged limitations due to the subject’s ability to modify the information collected (90). Moreover, the use of the factorial method to assess the AEE may be inaccurate when applied to individuals with different fat mass or FMM. Indeed, the compendium was developed to identify different classes of PA and normalize MET intensities in healthy populations (85). Studies confirmed that the normally used 1 MET value overestimated the resting (35%) and BEE (20%) in healthy adults and overestimated BEE in overweight and obese subjects (91, 92).
Objective Methods
Objective methods include tools that are based on physiological data (skin temperature or heart rate), mechanical data (pedometer, accelerometer, or inertial sensor), or a combination of both such as the SenseWear Armband (BodyMedia, Pittsburgh, PA, USA) or the Actiheart (Mini-Mitter Co., USA). The accurate measurement of TEE and AEE in subjects with DM is very challenging because, as demonstrated previously, patients with DM perform primarily low intensity PA, which may influence the assessment of AEE in several tools (93, 94). Therefore, these devices must be validated specifically for this population.
Few devices have been evaluated in people with DM. Mignault et al. (95) used the SenseWear Armband in six patients with DM for a 10-day free-living period to estimate their AEE and then compared it with the doubly labeled water based estimation. No significant difference was found between the two methods with a mean error of 78 kcal/day (r = 0.97). Machac et al. (94) evaluated the SenseWear Armband and the Omron HJ-720 (Omron Healthcare, Kyoto, Japan) under three walking conditions (3 km/h, 0%; 4 km/h, 0%; 5 km/h, 5%) in comparison with IC in 19 patients with DM. Their results showed a large overestimation with both devices at the lower speeds (MD = 70 and 81% at 3 km/h and 75 and 78% at 4 km/h for the Omron and Armband, respectively). On the contrary, both tools underestimated the AEE at 5 km/h, 5% (−7.3% for the Omron and −7.8% for the Armband).
As studies involving patients with DM are rare, the results of measurements for individuals with physical activities similar to those of patients with DM (low walking speed, light intensity exercise) could be enlightening. Studies in overweight and obese people without DM compared the Ormon and the SenseWear Armband with IC showing a similar overestimation of AEE during an exercise test (96, 97). In older adults, Mackey et al. (98) showed that the SenseWear Armband presented no difference from the gold standard for the assessment of TEE, but underestimated AEE by 18.5–26.8%. Colbert et al. (99) found RMSE of 185, 210, and 213 kcal/day for Actigraph (ActiGraph, LLC, USA), the SenseWear Armband, and NL-2000 (New-Lifestyles, Inc., Lee’s Summit, MO, USA), respectively. In another study, the Caltrac (Hemokinetics, Inc., Madison, WI, USA) underestimated TEE by −55 to −50% and no correlation was found with the double labeled water (100, 101). In their study of elderly men, Rafamantanantsoa et al. (102) compared TEE estimated by a heart rate monitor (Accurex Plus, Polar Electro Oy, Kempele, Finland) and an accelerometer (LifeCorder, Suzuken Co., Japan) with the doubly labeled water estimate. The heart rate method gives a better estimation of TEE (MD = 57 vs −542 kcal/day for accelerometry) but presented a higher intra-individual variation than the LifeCorder (coefficient of variation = 15 vs 7% for the accelerometer). As observed, objective methods suffer from acknowledged limitations. Accelerometry-based tools demonstrated poor accuracy at slow walking speeds and a decrease in precision with increasing body mass index (93). The device based on heart rate had a high variation in accuracy because the relation between heart rate and energy expenditure is not linear during rest and light intensity activity (103, 104). Yet, this range of intensities represented a major part of daily life activities in type 2 diabetic person.
Consequently, limited methods tested in people with DM may be used to self-check the calories burned daily. Currently, new technologies are accessible and marketed for estimating TEE or AEE and need to be tested in diabetic population.
Future Method for Evaluating AEE in Subjects with DM
As previously discussed, different methods exist for the assessment of TEE or AEE, but few have been validated in people with DM. Future research should address the weaknesses of methods already tested or experiment with new devices, perhaps by combining several technologies.
Accelerometry showed higher error particularly during low intensity activities and cycling (99, 105, 106). In order to overcome this limitation, Bonomi et al. (107) demonstrated that the AEE estimate via accelerometry can be improved if it is combined with detection of the type of activity. These results are interesting because the use of data provided by only one accelerometer through a decision tree allows the correct detection of activity in 93% of cases (105). Kwapisz et al. (108) found similar result (91.7%) with a smartphone in detecting six activities of daily life. Several studies reported great accuracy in estimating AEE compared with IC (RMSE = 0.69–1.25 METs and MSE = 0.25 METs) and a lower error as compared to another method (MSE = 2.05 METs for the Actiheart) (109–111). Inertial sensors consisting of a three dimensions accelerometer and gyroscope and a magnetometer can be used to identify the type of activity performed like previous accelerometers (112). These methods based on activity recognition could be a reliable solution for estimating more precisely the AEE in subjects with DM. Finally, the Actiheart (combining an accelerometer and a heart rate monitor) demonstrated high accuracy in standardized and free-living conditions for the prediction of AEE in healthy adults, but need to be tested in people with DM (113, 114).
Summary and Conclusion
This review emphasizes that there are differences between energy expenditure in patients with DM and healthy people. Patients with DM presented a higher BEE than healthy people. This difference seems to be due to an increase in FBG resulting in a higher glycosuria or gluconeogenesis. In addition, people with DM seem to have a lower AEE than healthy people. This review highlights that this lower AEE in patients with DM could be linked to a lower amount of activity (low compliance with PA recommendations) and the prevalence of low intensity activities. However, more studies should be conducted to determine the influence of diabetic altered gait on energy expenditure during PA. All these results demonstrate the need to develop adapted tools and methods to estimate free-living total energy expenditure in patients with DM. The results of this review indicate that there are valid equations for estimating BEE in patients with DM, but few of the methods tested give an accurate assessment of TEE and AEE in daily life. Other methods, such as those based on activity recognition with wearable sensors should be considered in the future to improve the estimation of daily TEE in subjects with DM. However, these possibilities need to be tested under everyday life conditions with patients with DM.
Author Contributions
Drafting of manuscript: NC. Critical revision: NC, NP, TC, CV, and GD. Final approval of the version to be published: NC, NP, TC, CV, and GD. Agreement to be accountable for all aspects of the work: NC, NP, TC, CV, and GD.
Conflict of Interest Statement
None of the authors of this manuscript have a direct financial relation with the commercial identities mentioned in this paper that might lead to a conflict of interests for any of the authors.
Acknowledgments
The authors wish to express their gratitude to Karim Assaley for technical assistance and to Shannon de Viviés for revising it for English translation.
Funding
This work was supported by a Regional Research Grant (grant #D2015033168) from the Réunion Région and from the European Regional Development Fund (FEDER).
Abbreviations
AEE, activity energy expenditure; BEE, basal energy expenditure; DM, diabetes mellitus; IC, indirect calorimetry; PA, physical activity; , rate of carbon dioxide production; , rate of oxygen consumption; TEE, total energy expenditure; TEF, thermic effect of food.
Footnotes
References
1. Wang H, Naghavi M, Allen C, Barber RM, Bhutta ZA, Carter A, et al. Global, regional, and national life expectancy, all-cause mortality, and cause-specific mortality for 249 causes of death, 1980–2015: a systematic analysis for the Global Burden of Disease Study 2015. Lancet (2016) 388(10053):1459–544. doi: 10.1016/S0140-6736(16)31012-1
2. GBD 2015 Disease and Injury Incidence and Prevalence Collaborators. Global, regional, and national incidence, prevalence, and years lived with disability for 310 diseases and injuries, 1990-2015: a systematic analysis for the Global Burden of Disease Study 2015. Lancet (2016) 388(10053):1545–602. doi:10.1016/S0140-6736(16)31678-6
3. Forouzanfar MH, Afshin A, Alexander LT, Anderson HR, Bhutta ZA, Biryukov S, et al. Global, regional, and national comparative risk assessment of 79 behavioural, environmental and occupational, and metabolic risks or clusters of risks, 1990–2015: a systematic analysis for the Global Burden of Disease Study 2015. Lancet (2016) 388(10053):1659–724. doi:10.1016/S0140-6736(16)31679-8
4. Wild S, Roglic G, Green A, Sicree R, King H. Global prevalence of diabetes: estimates for the year 2000 and projections for 2030. Diabetes Care (2004) 27(5):1047–53. doi:10.2337/diacare.27.5.1047
5. Whiting DR, Guariguata L, Weil C, Shaw J. IDF diabetes atlas: global estimates of the prevalence of diabetes for 2011 and 2030. Diabetes Res Clin Pract (2011) 94(3):311–21. doi:10.1016/j.diabres.2011.10.029
6. Lim SS, Allen K, Bhutta ZA, Dandona L, Forouzanfar MH, Fullman N, et al. Measuring the health-related sustainable development goals in 188 countries: a baseline analysis from the Global Burden of Disease Study 2015. Lancet (2016) 388(10053):1813–50. doi:10.1016/S0140-6736(16)31467-2
7. Aune D, Norat T, Leitzmann M, Tonstad S, Vatten LJ. Physical activity and the risk of type 2 diabetes: a systematic review and dose-response meta-analysis. Eur J Epidemiol (2015) 30(7):529–42. doi:10.1007/s10654-015-0056-z
8. Grontved A, Pan A, Mekary RA, Stampfer M, Willett WC, Manson JE, et al. Muscle-strengthening and conditioning activities and risk of type 2 diabetes: a prospective study in two cohorts of US women. PLoS Med (2014) 11(1):e1001587. doi:10.1371/journal.pmed.1001587
9. Jefferis BJ, Whincup PH, Lennon L, Wannamethee SG. Longitudinal associations between changes in physical activity and onset of type 2 diabetes in older British men: the influence of adiposity. Diabetes Care (2012) 35(9):1876–83. doi:10.2337/dc11-2280
10. Sieverdes JC, Sui X, Lee DC, Church TS, McClain A, Hand GA, et al. Physical activity, cardiorespiratory fitness and the incidence of type 2 diabetes in a prospective study of men. Br J Sports Med (2010) 44(4):238–44. doi:10.1136/bjsm.2009.062117
11. Grontved A, Rimm EB, Willett WC, Andersen LB, Hu FB. A prospective study of weight training and risk of type 2 diabetes mellitus in men. Arch Intern Med (2012) 172(17):1306–12. doi:10.1001/archinternmed.2012.3138
12. van Baak MA. Physical activity and energy balance. Public Health Nutr (1999) 2(3A):335–9. doi:10.1017/S1368980099000452
13. Lazarevic G, Antic S, Cvetkovic T, Vlahovic P, Tasic I, Stefanovic V. A physical activity programme and its effects on insulin resistance and oxidative defense in obese male patients with type 2 diabetes mellitus. Diabetes Metab (2006) 32(6):583–90. doi:10.1016/S1262-3636(07)70312-9
14. Sukla P, Shrivastava SR, Shrivastava PS. A longitudinal study to assess the impact of exercise on clinical, biochemical, and anthropometric parameters among the type 2 diabetes patients of South India. Avicenna J Med (2015) 5(1):16–20. doi:10.4103/2231-0770.148504
15. Chudyk A, Petrella RJ. Effects of exercise on cardiovascular risk factors in type 2 diabetes: a meta-analysis. Diabetes Care (2011) 34(5):1228–37. doi:10.2337/dc10-1881
16. Emerenziani GP, Gallotta MC, Meucci M, Di Luigi L, Migliaccio S, Donini LM, et al. Effects of aerobic exercise based upon heart rate at aerobic threshold in obese elderly subjects with type 2 diabetes. Int J Endocrinol (2015) 2015:695297. doi:10.1155/2015/695297
17. Castaneda C, Layne JE, Munoz-Orians L, Gordon PL, Walsmith J, Foldvari M, et al. A randomized controlled trial of resistance exercise training to improve glycemic control in older adults with type 2 diabetes. Diabetes Care (2002) 25(12):2335–41. doi:10.2337/diacare.25.12.2335
18. Sigal RJ, Kenny GP, Boule NG, Wells GA, Prud’homme D, Fortier M, et al. Effects of aerobic training, resistance training, or both on glycemic control in type 2 diabetes: a randomized trial. Ann Intern Med (2007) 147(6):357–69. doi:10.7326/0003-4819-147-6-200709180-00005
19. Janevic MR, McLaughlin SJ, Connell CM. The association of diabetes complications with physical activity in a representative sample of older adults in the United States. Chronic Illn (2013) 9(4):251–7. doi:10.1177/1742395313475461
20. Martinez-Gomez D, Guallar-Castillon P, Mota J, Lopez-Garcia E, Rodriguez-Artalejo F. Physical activity, sitting time and mortality in older adults with diabetes. Int J Sports Med (2015) 36(14):1206–11. doi:10.1055/s-0035-1555860
21. Hu G, Jousilahti P, Barengo NC, Qiao Q, Lakka TA, Tuomilehto J. Physical activity, cardiovascular risk factors, and mortality among Finnish adults with diabetes. Diabetes Care (2005) 28(4):799–805. doi:10.2337/diacare.28.4.799
22. Ryan M, Salle A, Guilloteau G, Genaitay M, Livingstone MB, Ritz P. Resting energy expenditure is not increased in mildly hyperglycaemic obese diabetic patients. Br J Nutr (2006) 96(5):945–8. doi:10.1017/BJN20061744
23. Fontvieille AM, Lillioja S, Ferraro RT, Schulz LO, Rising R, Ravussin E. Twenty-four-hour energy expenditure in Pima Indians with type 2 (non-insulin-dependent) diabetes mellitus. Diabetologia (1992) 35(8):753–9.
24. Ucok K, Yalcinkaya H, Acay A, Coban NF, Aslanalp S, Akkan G, et al. Do patients with newly diagnosed type 2 diabetes have impaired physical fitness, and energy expenditures? Neth J Med (2015) 73(6):276–83.
25. Bitz C, Toubro S, Larsen TM, Harder H, Rennie KL, Jebb SA, et al. Increased 24-h energy expenditure in type 2 diabetes. Diabetes Care (2004) 27(10):2416–21. doi:10.2337/diacare.27.10.2416
26. Franssila-Kallunki A, Groop L. Factors associated with basal metabolic rate in patients with type 2 (non-insulin-dependent) diabetes mellitus. Diabetologia (1992) 35(10):962–6. doi:10.1007/BF00401426
27. Buscemi S, Donatelli M, Grosso G, Vasto S, Galvano F, Costa F, et al. Resting energy expenditure in type 2 diabetic patients and the effect of insulin bolus. Diabetes Res Clin Pract (2014) 106(3):605–10. doi:10.1016/j.diabres.2014.09.016
28. Alawad AO, Merghani TH, Ballal MA. Resting metabolic rate in obese diabetic and obese non-diabetic subjects and its relation to glycaemic control. BMC Res Notes (2013) 6:382. doi:10.1186/1756-0500-6-382
29. Gougeon R, Lamarche M, Yale JF, Venuta T. The prediction of resting energy expenditure in type 2 diabetes mellitus is improved by factoring for glycemia. Int J Obes Relat Metab Disord (2002) 26(12):1547–52. doi:10.1038/sj.ijo.0802178
30. Huang KC, Kormas N, Steinbeck K, Loughnan G, Caterson ID. Resting metabolic rate in severely obese diabetic and nondiabetic subjects. Obes Res (2004) 12(5):840–5. doi:10.1038/oby.2004.101
31. Cunningham JJ. Body composition as a determinant of energy expenditure: a synthetic review and a proposed general prediction equation. Am J Clin Nutr (1991) 54(6):963–9.
32. Ferrannini E. Sodium-glucose transporter-2 inhibition as an antidiabetic therapy. Nephrol Dial Transplant (2010) 25(7):2041–3. doi:10.1093/ndt/gfq249
33. Piaggi P, Thearle MS, Bogardus C, Krakoff J. Fasting hyperglycemia predicts lower rates of weight gain by increased energy expenditure and fat oxidation rate. J Clin Endocrinol Metab (2015) 100(3):1078–87. doi:10.1210/jc.2014-3582
34. Tataranni PA, Larson DE, Snitker S, Ravussin E. Thermic effect of food in humans: methods and results from use of a respiratory chamber. Am J Clin Nutr (1995) 61(5):1013–9.
35. Weyer C, Bogardus C, Pratley RE. Metabolic factors contributing to increased resting metabolic rate and decreased insulin-induced thermogenesis during the development of type 2 diabetes. Diabetes (1999) 48(8):1607–14. doi:10.2337/diabetes.48.8.1607
36. Felber JP, Meyer HU, Curchod B, Iselin HU, Rousselle J, Maeder E, et al. Glucose storage and oxidation in different degrees of human obesity measured by continuous indirect calorimetry. Diabetologia (1981) 20(1):39–44. doi:10.1007/BF00253814
37. Christin L, Nacht CA, Vernet O, Ravussin E, Jequier E, Acheson KJ. Insulin. Its role in the thermic effect of glucose. J Clin Invest (1986) 77(6):1747–55. doi:10.1172/JCI112497
38. Fagour C, Gonzalez C, Pezzino S, Florenty S, Rosette-Narece M, Gin H, et al. Low physical activity in patients with type 2 diabetes: the role of obesity. Diabetes Metab (2013) 39(1):85–7. doi:10.1016/j.diabet.2012.09.003
39. U.S Department of Health and Human Services. Physical Activity and Health: A Report of the Surgeon Genera. Atlanta, GA: U.S. Department of Health and Human Services, Centers for Disease Control and Prevention, National Center for Chronic Disease Prevention and Health Promotion (1996).
40. Tudor-Locke C, Bassett DR Jr. How many steps/day are enough? Preliminary pedometer indices for public health. Sports Med (2004) 34(1):1–8. doi:10.2165/00007256-200434010-00001
41. Le Masurier GC, Sidman CL, Corbin CB. Accumulating 10,000 steps: does this meet current physical activity guidelines? Res Q Exerc Sport (2003) 74(4):389–94. doi:10.1080/02701367.2003.10609109
42. Pate RR, Pratt M, Blair SN, Haskell WL, Macera CA, Bouchard C, et al. Physical activity and public health. A recommendation from the Centers for Disease Control and Prevention and the American College of Sports Medicine. JAMA (1995) 273(5):402–7. doi:10.1001/jama.1995.03520290054029
43. Hays LM, Clark DO. Correlates of physical activity in a sample of older adults with type 2 diabetes. Diabetes Care (1999) 22(5):706–12. doi:10.2337/diacare.22.5.706
44. Wood FG. Leisure time activity of Mexican Americans with diabetes. J Adv Nurs (2004) 45(2):190–6. doi:10.1046/j.1365-2648.2003.02880.x
45. Nelson KM, Reiber G, Boyko EJ, Nhanes III. Diet and exercise among adults with type 2 diabetes: findings from the third national health and nutrition examination survey (NHANES III). Diabetes Care (2002) 25(10):1722–8. doi:10.2337/diacare.25.10.1722
46. Zhao G, Ford ES, Li C, Balluz LS. Physical activity in U.S. older adults with diabetes mellitus: prevalence and correlates of meeting physical activity recommendations. J Am Geriatr Soc (2011) 59(1):132–7. doi:10.1111/j.1532-5415.2010.03236.x
47. Resnick HE, Foster GL, Bardsley J, Ratner RE. Achievement of American Diabetes Association clinical practice recommendations among U.S. adults with diabetes, 1999-2002: the National Health and Nutrition Examination Survey. Diabetes Care (2006) 29(3):531–7. doi:10.2337/diacare.29.03.06.dc05-1254
48. Scheers T, Philippaerts R, Lefevre J. Compliance with different physical activity recommendations and its association with socio-demographic characteristics using an objective measure. BMC Public Health (2013) 13:136. doi:10.1186/1471-2458-13-136
49. Baldew SS, Krishnadath IS, Smits CC, Toelsie JR, Vanhees L, Cornelissen V. Self-reported physical activity behavior of a multi-ethnic adult population within the urban and rural setting in Suriname. BMC Public Health (2015) 15:485. doi:10.1186/s12889-015-1807-1
50. Hamrik Z, Sigmundova D, Kalman M, Pavelka J, Sigmund E. Physical activity and sedentary behaviour in Czech adults: results from the GPAQ study. Eur J Sport Sci (2014) 14(2):193–8. doi:10.1080/17461391.2013.822565
51. Bauman A, Bull F, Chey T, Craig CL, Ainsworth BE, Sallis JF, et al. The International Prevalence Study on physical activity: results from 20 countries. Int J Behav Nutr Phys Act (2009) 6:21. doi:10.1186/1479-5868-6-21
52. Hallal PC, Andersen LB, Bull FC, Guthold R, Haskell W, Ekelund U, et al. Global physical activity levels: surveillance progress, pitfalls, and prospects. Lancet (2012) 380(9838):247–57. doi:10.1016/S0140-6736(12)60646-1
53. Barrett JE, Plotnikoff RC, Courneya KS, Raine KD. Physical activity and type 2 diabetes: exploring the role of gender and income. Diabetes Educ (2007) 33(1):128–43. doi:10.1177/0145721706297453
54. Araiza P, Hewes H, Gashetewa C, Vella CA, Burge MR. Efficacy of a pedometer-based physical activity program on parameters of diabetes control in type 2 diabetes mellitus. Metabolism (2006) 55(10):1382–7. doi:10.1016/j.metabol.2006.06.009
55. Richardson CR, Mehari KS, McIntyre LG, Janney AW, Fortlage LA, Sen A, et al. A randomized trial comparing structured and lifestyle goals in an internet-mediated walking program for people with type 2 diabetes. Int J Behav Nutr Phys Act (2007) 4:59. doi:10.1186/1479-5868-4-59
56. Strycker LA, Duncan SC, Chaumeton NR, Duncan TE, Toobert DJ. Reliability of pedometer data in samples of youth and older women. Int J Behav Nutr Phys Act (2007) 4:4. doi:10.1186/1479-5868-4-4
57. Bjorgaas M, Vik JT, Saeterhaug A, Langlo L, Sakshaug T, Mohus RM, et al. Relationship between pedometer-registered activity, aerobic capacity and self-reported activity and fitness in patients with type 2 diabetes. Diabetes Obes Metab (2005) 7(6):737–44. doi:10.1111/j.1463-1326.2004.00464.x
58. Tudor-Locke C. A preliminary study to determine instrument responsiveness to change with a walking program: physical activity logs versus pedometers. Res Q Exerc Sport (2001) 72(3):288–92. doi:10.1080/02701367.2001.10608962
59. Tudor-Locke CE, Bell RC, Myers AM, Harris SB, Lauzon N, Rodger NW. Pedometer-determined ambulatory activity in individuals with type 2 diabetes. Diabetes Res Clin Pract (2002) 55(3):191–9. doi:10.1016/S0168-8227(01)00317-5
60. Mitsui T, Shimaoka K, Tsuzuku S, Kajioka T, Sakakibara H. Pedometer-determined physical activity and indicators of health in Japanese adults. J Physiol Anthropol (2008) 27(4):179–84. doi:10.2114/jpa2.27.179
61. Jakicic JM, Gregg E, Knowler W, Kelley DE, Lang W, Miller GD, et al. Activity patterns of obese adults with type 2 diabetes in the look AHEAD study. Med Sci Sports Exerc (2010) 42(11):1995–2005. doi:10.1249/MSS.0b013e3181e054f0
62. Kriska AM, Hanley AJ, Harris SB, Zinman B. Physical activity, physical fitness, and insulin and glucose concentrations in an isolated native Canadian population experiencing rapid lifestyle change. Diabetes Care (2001) 24(10):1787–92. doi:10.2337/diacare.24.10.1787
63. Ford ES, Herman WH. Leisure-time physical activity patterns in the U.S. diabetic population. Findings from the 1990 National Health Interview Survey – health promotion and disease prevention supplement. Diabetes Care (1995) 18(1):27–33. doi:10.2337/diacare.18.1.27
64. Steeves JA, Murphy RA, Crainiceanu CM, Zipunnikov V, Van Domelen DR, Harris TB. Daily patterns of physical activity by type 2 diabetes definition: comparing diabetes, prediabetes, and participants with normal glucose levels in NHANES 2003-2006. Prev Med Rep (2015) 2:152–7. doi:10.1016/j.pmedr.2015.02.007
65. Colberg SR, Hagberg JM, McCole SD, Zmuda JM, Thompson PD, Kelley DE. Utilization of glycogen but not plasma glucose is reduced in individuals with NIDDM during mild-intensity exercise. J Appl Physiol (1985) (1996) 81(5):2027–33.
66. Blaak EE, van Aggel-Leijssen DP, Wagenmakers AJ, Saris WH, van Baak MA. Impaired oxidation of plasma-derived fatty acids in type 2 diabetic subjects during moderate-intensity exercise. Diabetes (2000) 49(12):2102–7. doi:10.2337/diabetes.49.12.2102
67. Raspovic A. Gait characteristics of people with diabetes-related peripheral neuropathy, with and without a history of ulceration. Gait Posture (2013) 38(4):723–8. doi:10.1016/j.gaitpost.2013.03.009
68. Fernando M, Crowther R, Lazzarini P, Sangla K, Cunningham M, Buttner P, et al. Biomechanical characteristics of peripheral diabetic neuropathy: a systematic review and meta-analysis of findings from the gait cycle, muscle activity and dynamic barefoot plantar pressure. Clin Biomech (Bristol, Avon) (2013) 28(8):831–45. doi:10.1016/j.clinbiomech.2013.08.004
69. Petrovic M, Deschamps K, Verschueren SM, Bowling FL, Maganaris CN, Boulton AJ, et al. Is the metabolic cost of walking higher in people with diabetes? J Appl Physiol (1985) (2016) 120(1):55–62. doi:10.1152/japplphysiol.00413.2015
70. Kwon OY, Minor SD, Maluf KS, Mueller MJ. Comparison of muscle activity during walking in subjects with and without diabetic neuropathy. Gait Posture (2003) 18(1):105–13. doi:10.1016/S0966-6362(02)00166-2
71. Maiolo C, Mohamed EI, Servidio MF, De Luna A, Meloni P, Bertoli A, et al. The effect of physical activities of various intensities on the energy expenditure of type 2 diabetic men. Acta Diabetol (2003) 40(Suppl 1):S126–9. doi:10.1007/s00592-003-0044-8
72. Harris JA, Benedict FG. A biometric study of human basal metabolism. Proc Natl Acad Sci U S A (1918) 4(12):370–3. doi:10.1073/pnas.4.12.370
73. Ganpule AA, Tanaka S, Ishikawa-Takata K, Tabata I. Interindividual variability in sleeping metabolic rate in Japanese subjects. Eur J Clin Nutr (2007) 61(11):1256–61. doi:10.1038/sj.ejcn.1602645
74. Schofield WN. Predicting basal metabolic rate, new standards and review of previous work. Hum Nutr Clin Nutr (1985) 39(Suppl 1):5–41.
75. Mifflin MD, St Jeor ST, Hill LA, Scott BJ, Daugherty SA, Koh YO. A new predictive equation for resting energy expenditure in healthy individuals. Am J Clin Nutr (1990) 51(2):241–7.
76. Owen OE. Resting metabolic requirements of men and women. Mayo Clin Proc (1988) 63(5):503–10. doi:10.1016/S0025-6196(12)65649-3
77. Bernstein RS, Thornton JC, Yang MU, Wang J, Redmond AM, Pierson RN Jr, et al. Prediction of the resting metabolic rate in obese patients. Am J Clin Nutr (1983) 37(4):595–602.
78. Merghani TH, Alawad AO, Ballal MA. Measured versus predicted resting metabolic rate in obese diabetic and obese non-diabetic subjects. J Dent Med Sci (2013) 10(2):63–7. doi:10.9790/0853-01026367
79. Ikeda K, Fujimoto S, Goto M, Yamada C, Hamasaki A, Ida M, et al. A new equation to estimate basal energy expenditure of patients with diabetes. Clin Nutr (2013) 32(5):777–82. doi:10.1016/j.clnu.2012.11.017
80. Martin K, Wallace P, Rust PF, Garvey WT. Estimation of resting energy expenditure considering effects of race and diabetes status. Diabetes Care (2004) 27(6):1405–11. doi:10.2337/diacare.27.6.1405
81. Miyake R, Ohkawara K, Ishikawa-Takata K, Morita A, Watanabe S, Tanaka S. Obese Japanese adults with type 2 diabetes have higher basal metabolic rates than non-diabetic adults. J Nutr Sci Vitaminol (Tokyo) (2011) 57(5):348–54. doi:10.3177/jnsv.57.348
82. Ferraro R, Ravussin E. Fat mass in predicting resting metabolic rate. Am J Clin Nutr (1992) 56(2):460–1.
83. de Figueiredo Ferreira M, Detrano F, Coelho GM, Barros ME, Serrao Lanzillotti R, Firmino Nogueira Neto J, et al. Body composition and basal metabolic rate in women with type 2 diabetes mellitus. J Nutr Metab (2014) 2014:574057. doi:10.1155/2014/574057
84. Levine JA. Measurement of energy expenditure. Public Health Nutr (2005) 8(7A):1123–32. doi:10.1079/PHN2005800
85. Ainsworth BE, Haskell WL, Whitt MC, Irwin ML, Swartz AM, Strath SJ, et al. Compendium of physical activities: an update of activity codes and MET intensities. Med Sci Sports Exerc (2000) 32(9 Suppl):S498–504. doi:10.1097/00005768-200009001-00009
86. Kriska AM, Knowler WC, LaPorte RE, Drash AL, Wing RR, Blair SN, et al. Development of questionnaire to examine relationship of physical activity and diabetes in Pima Indians. Diabetes Care (1990) 13(4):401–11. doi:10.2337/diacare.13.4.401
87. Schulz LO, Harper IT, Smith CJ, Kriska AM, Ravussin E. Energy intake and physical activity in Pima Indians: comparison with energy expenditure measured by doubly-labeled water. Obes Res (1994) 2(6):541–8. doi:10.1002/j.1550-8528.1994.tb00103.x
88. Criniere L, Lhommet C, Caille A, Giraudeau B, Lecomte P, Couet C, et al. Reproducibility and validity of the French version of the long international physical activity questionnaire in patients with type 2 diabetes. J Phys Act Health (2011) 8(6):858–65. doi:10.1123/jpah.8.6.858
89. Mynarski W, Psurek A, Borek Z, Rozpara M, Grabara M, Strojek K. Declared and real physical activity in patients with type 2 diabetes mellitus as assessed by the International Physical Activity Questionnaire and Caltrac accelerometer monitor: a potential tool for physical activity assessment in patients with type 2 diabetes mellitus. Diabetes Res Clin Pract (2012) 98(1):46–50. doi:10.1016/j.diabres.2012.05.024
90. Shephard RJ. Limits to the measurement of habitual physical activity by questionnaires. Br J Sports Med (2003) 37(3):197–206; discussion 206. doi:10.1136/bjsm.37.3.197
91. Wilms B, Ernst B, Thurnheer M, Weisser B, Schultes B. Correction factors for the calculation of metabolic equivalents (MET) in overweight to extremely obese subjects. Int J Obes (Lond) (2014) 38(11):1383–7. doi:10.1038/ijo.2014.22
92. Byrne NM, Hills AP, Hunter GR, Weinsier RL, Schutz Y. Metabolic equivalent: one size does not fit all. J Appl Physiol (1985) (2005) 99(3):1112–9. doi:10.1152/japplphysiol.00023.2004
93. Tyo BM, Fitzhugh EC, Bassett DR Jr, John D, Feito Y, Thompson DL. Effects of body mass index and step rate on pedometer error in a free-living environment. Med Sci Sports Exerc (2011) 43(2):350–6. doi:10.1249/MSS.0b013e3181e9b133
94. Machac S, Prochazka M, Radvansky J, Slaby K. Validation of physical activity monitors in individuals with diabetes: energy expenditure estimation by the multisensor SenseWear Armband Pro3 and the step counter Omron HJ-720 against indirect calorimetry during walking. Diabetes Technol Ther (2013) 15(5):413–8. doi:10.1089/dia.2012.0235
95. Mignault D, St-Onge M, Karelis AD, Allison DB, Rabasa-Lhoret R. Evaluation of the portable HealthWear armband: a device to measure total daily energy expenditure in free-living type 2 diabetic individuals. Diabetes Care (2005) 28(1):225–7. doi:10.2337/diacare.28.1.225-a
96. Swartz AM, Strath SJ, Miller NE, Grimm EK, Ewalt LA, Loy MS, et al. Validity of physical activity monitors in assessing energy expenditure in normal, overweight, and obese adults. Open Sports Sci J (2009) 2:58–64. doi:10.2174/1875399X00902010058
97. Papazoglou D, Augello G, Tagliaferri M, Savia G, Marzullo P, Maltezos E, et al. Evaluation of a multisensor armband in estimating energy expenditure in obese individuals. Obesity (Silver Spring) (2006) 14(12):2217–23. doi:10.1038/oby.2006.260
98. Mackey DC, Manini TM, Schoeller DA, Koster A, Glynn NW, Goodpaster BH, et al. Validation of an armband to measure daily energy expenditure in older adults. J Gerontol A Biol Sci Med Sci (2011) 66(10):1108–13. doi:10.1093/gerona/glr101
99. Colbert LH, Matthews CE, Havighurst TC, Kim K, Schoeller DA. Comparative validity of physical activity measures in older adults. Med Sci Sports Exerc (2011) 43(5):867–76. doi:10.1249/MSS.0b013e3181fc7162
100. Starling RD, Matthews DE, Ades PA, Poehlman ET. Assessment of physical activity in older individuals: a doubly labeled water study. J Appl Physiol (1985) (1999) 86(6):2090–6.
101. Choquette S, Chuin A, Lalancette DA, Brochu M, Dionne IJ. Predicting energy expenditure in elders with the metabolic cost of activities. Med Sci Sports Exerc (2009) 41(10):1915–20. doi:10.1249/MSS.0b013e3181a6164a
102. Rafamantanantsoa HH, Ebine N, Yoshioka M, Higuchi H, Yoshitake Y, Tanaka H, et al. Validation of three alternative methods to measure total energy expenditure against the doubly labeled water method for older Japanese men. J Nutr Sci Vitaminol (Tokyo) (2002) 48(6):517–23. doi:10.3177/jnsv.48.517
103. Li R, Deurenberg P, Hautvast JG. A critical evaluation of heart rate monitoring to assess energy expenditure in individuals. Am J Clin Nutr (1993) 58(5):602–7.
104. Ainslie P, Reilly T, Westerterp K. Estimating human energy expenditure: a review of techniques with particular reference to doubly labelled water. Sports Med (2003) 33(9):683–98. doi:10.2165/00007256-200333090-00004
105. Bonomi AG, Goris AH, Yin B, Westerterp KR. Detection of type, duration, and intensity of physical activity using an accelerometer. Med Sci Sports Exerc (2009) 41(9):1770–7. doi:10.1249/MSS.0b013e3181a24536
106. Nelson MB, Kaminsky LA, Dickin DC, Montoye AH. Validity of consumer-based physical activity monitors for specific activity types. Med Sci Sports Exerc (2016) 48(8):1619–28. doi:10.1249/MSS.0000000000000933
107. Bonomi AG, Plasqui G, Goris AH, Westerterp KR. Improving assessment of daily energy expenditure by identifying types of physical activity with a single accelerometer. J Appl Physiol (1985) (2009) 107(3):655–61. doi:10.1152/japplphysiol.00150.2009
108. Kwapisz JR, Weiss GM, Moore SA. Activity recognition using cell phone accelerometers. ACM SIGKDD Explor (2010) 12(2):74–82. doi:10.1145/1964897.1964918
109. Staudenmayer J, Pober D, Crouter S, Bassett D, Freedson P. An artificial neural network to estimate physical activity energy expenditure and identify physical activity type from an accelerometer. J Appl Physiol (1985) (2009) 107(4):1300–7. doi:10.1152/japplphysiol.00465.2009
110. Schneller MB, Pedersen MT, Gupta N, Aadahl M, Holtermann A. Validation of five minimally obstructive methods to estimate physical activity energy expenditure in young adults in semi-standardized settings. Sensors (Basel) (2015) 15(3):6133–51. doi:10.3390/s150306133
111. Sazonov E, Hegde N, Browning RC, Melanson EL, Sazonova NA. Posture and activity recognition and energy expenditure estimation in a wearable platform. IEEE J Biomed Health Inform (2015) 19(4):1339–46. doi:10.1109/JBHI.2015.2432454
112. Freedson PS, Miller K. Objective monitoring of physical activity using motion sensors and heart rate. Res Q Exerc Sport (2000) 71(2 Suppl):S21–9. doi:10.1080/02701367.2000.11082782
113. Bareira TV, Kang M, Caputo JL, Farley RS, Renfrow MS. Validation of the actiheart monitor for the measurement of physical activity. Int J Med Sci (2009) 2(1):60–71.
Keywords: type 2 diabetes, metabolic cost, physical activity, guideline, sensor
Citation: Caron N, Peyrot N, Caderby T, Verkindt C and Dalleau G (2016) Energy Expenditure in People with Diabetes Mellitus: A Review. Front. Nutr. 3:56. doi: 10.3389/fnut.2016.00056
Received: 15 November 2016; Accepted: 08 December 2016;
Published: 22 December 2016
Edited by:
Jan Polák, Charles University, Czech RepublicReviewed by:
Rade Vukovic, Mother and Child Health Care Institute of Serbia, SerbiaMihajlo (Michael) Jakovljevic, University of Kragujevac, Serbia; Hosei University, Japan
Copyright: © 2016 Caron, Peyrot, Caderby, Verkindt and Dalleau. This is an open-access article distributed under the terms of the Creative Commons Attribution License (CC BY). The use, distribution or reproduction in other forums is permitted, provided the original author(s) or licensor are credited and that the original publication in this journal is cited, in accordance with accepted academic practice. No use, distribution or reproduction is permitted which does not comply with these terms.
*Correspondence: Nathan Caron, nathan.caron@univ-reunion.fr