- Department of Neurosurgery, School of Medicine, University of Colorado, Aurora, CO, United States
In the treatment of brain tumors, surgical intervention remains a common and effective therapeutic option. Recent advances in neuroimaging have provided neurosurgeons with new tools to overcome the challenge of differentiating healthy tissue from tumor-infiltrated tissue, with the aim of increasing the likelihood of maximizing the extent of resection volume while minimizing injury to functionally important regions. Novel applications of diffusion tensor imaging (DTI), and DTI-derived tractography (DDT) have demonstrated that preoperative, non-invasive mapping of eloquent cortical regions and functionally relevant white matter tracts (WMT) is critical during surgical planning to reduce postoperative deficits, which can decrease quality of life and overall survival. In this review, we summarize the latest developments of applying DTI and tractography in the context of resective surgery and highlight its utility within each stage of the neurosurgical workflow: preoperative planning and intraoperative management to improve postoperative outcomes.
Introduction
Surgical resection remains the front-line treatment for many forms of intracranial tumor (1). Evidence from multiple large case series clearly supports the benefit of greater extent of resection (EOR) for patients with low-grade, high-grade, and recurrent gliomas with respect to their overall survival and progression-free survival outcomes (2–8). Moreover, preserving cortical and subcortical function is important for better postoperative outcomes and improved quality of life (9). Recent advances in neurosurgical planning and navigation technology seek to address two main issues: (a) non-invasive means of identifying tumor pathology and (b) non-invasive mapping of functional brain areas. Currently, the gold standard for each issue involves invasive methods such as biopsy and direct electrical stimulation (DES), respectively.
Surgical planning has benefited greatly from advances in neuroimaging. Yet, there remain a number of barriers preventing accurate determination of tumor pathology using noninvasive means alone. Biopsy is typically relied upon to determine pathology and grading. However, it is subject to sampling error (10, 11) and is often limited to easily accessible, enhancing regions of the tumor (12). More importantly, unnecessary surgical intervention poses a risk to patient comfort and quality of life, and risks complications especially when performed in eloquent regions of the brain (13). While DES is currently the most trusted method to identify functional tissue, depending on the geometry and grid arrangement of the electrode array, this technique has 2–3 mm or greater spatial resolution (14). As a result, DES is often accompanied with a margin of safety so that functional areas have a higher probability to be preserved. Yet, it does not always provide reliable responses and can potentially induce seizures. DES can also cause anxiety and fatigue for patients who must remain awake for extended periods of time for mapping during tumor excision.
Recent case series have highlighted the use of DTI to circumvent the weaknesses of both DES and biopsy. DTI measures water diffusion properties of neural tissue and can be used to approximate functionally relevant anatomical WMT in the brain. Within the context of intracranial tumor diagnosis and treatment, DTI has been used for planning neurosurgical interventions (15, 16), defining areas to excise and areas to avoid (17, 18), preoperatively differentiating tumor pathology, (19, 20), intraoperative estimation of brain shift (21), improving location/orientation targeting for DES sites (15, 22), improving postoperative functionality scores (23), assessing postoperative morbidity and mortality using preoperative information (24–26), and so on. Despite the diverse clinical applications for DTI within neurosurgical oncology, the method remains underutilized and lacks Level I evidence support. In resective surgery, DDT is primarily used in preoperative surgical planning and as an adjunct for the intraoperative functional information provided by DES. In the latter usage, tractography plays a limited role—providing location estimates for DES stimulation or as a coarse substitute for functional brain areas when DES is unavailable or not working properly.
In this review, we will discuss applications of DTI and DDT in the context of neurosurgical tumor resection, provide an in-depth description of current developments, and consider their limitations.
Preoperative Applications of DTI
Tumor Pathology Classification
Treatment strategies for intracranial tumors differ considerably based on pathology and grading, and optimal prognosis is dependent on strategy (27, 28). Non-invasive methods for diagnosis, such as conventional magnetic resonance imaging (MRI), currently do not provide the accuracy required for clinical decision-making (29). Gliomas are known to be a heterogeneous group with highly variable imaging qualities—attributed to variation in genetic and epigenetic mutations occurring early in tumorigenesis (30). To make matters more difficult, gliomas, and metastases can be difficult to visually differentiate, as these tumors display similar signal intensities and contrast enhancement patterns (31). While conventional MRI demonstrates some ability to classify pathology, resulting in a correct basic diagnosis 81% of the time (32), additional information is necessary to be clinically useful.
Diffusion coefficients in neoplastic brain tissue provide additional information for detecting tissue differences in and proximal to the tumor (33). Water movement in the brain is a blend of locally unrestricted, uniform fluid movement (isotropic component) within regions like the ventricles and restricted fluid movement (anisotropic component) within axonal membranes, myelin sheaths, and the extracellular matrix. At each voxel, an ellipsoid is calculated to approximate diffusivity in three-dimensional space, and each dimension (or aggregate of dimensions) may relay information about tissue properties or types (34, 35). Links between diffusion and cellularity, cell density, and proliferation have been reported: mean diffusivity (MD; average diffusion across spatial dimensions) decreases with increasing cellularity (29) and fractional anisotropy (FA; measure of directionality of diffusion in a given voxel) increases with increasing cell density and proliferation (36). It is important to note that these relationships are often specific to the pathology under investigation. Studies have shown that tumor pathology alters the diffusion properties of neural tissue in distinct ways (37–39). For instance, restricted water diffusion in solid tumors has been reported by many groups (36, 40).
Numerous studies have investigated the possibility of differentiating between gliomas and metastases (39, 41–52) and between low- and high-grade gliomas (53, 54) with mixed success. The lack of consensus may well be due to differences in DTI acquisition, preprocessing and postprocessing protocols, region of interest (ROI) location selection, and sample size. One commonality these articles share is applying logistic regression or receiver-operator curve analysis to classify pathology from ROI-derived TI coefficient averages, in or around the tumor (e.g., enhancing or immediate peritumoral regions) (49). Ultimately, two diffusion trends between high-grade gliomas and metastases were uncovered: increased FA intratumorally and peritumorally in gliomas and increased MD peritumorally in metastases (Table 1).
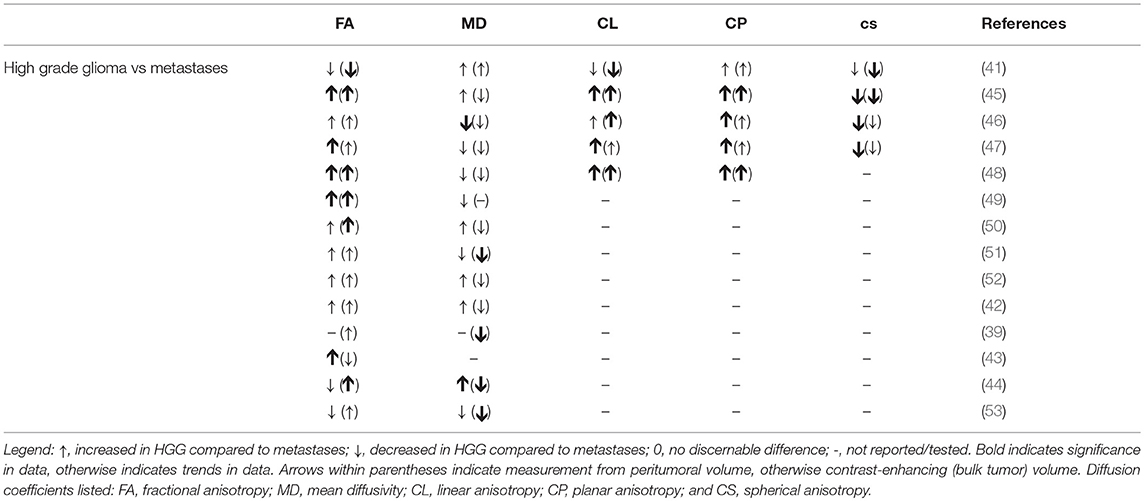
Table 1. Comparisons of diffusion coefficients in high-grade glioma(HGG) and metastasesfrom ROIs of enhancing and immediate peritumoral volumes.
Advanced techniques, including additional imaging modalities and/or more sophisticated machine learning methods, such as support vector machines and neural networks, may result in improved diagnostic performance (50, 55). For example, Chen et al. (55) applied a Bayesian network model incorporating DTI, T1-weighted, FLAIR, and MR perfusion measurements to differentiate between glioblastomas and solitary brain metastases. The predictive accuracy of the model was 0.94 with sensitivity = 0.96 and specificity = 0.92. For comparison, the specificity and sensitivity of those listed in Table 1 range between (0.77, 1.0) and (0.63, 0.92), respectively. Moreover, the research demonstrated 8 of 36 biomarkers retained its predictive power: 6 from DTI, 2 from MR perfusion, and 1 from FLAIR. Not only does this show the utility of the information contained in diffusion coefficients, it also demonstrates that combining imaging modalities with an advanced classification model may hold the key to accurate non-invasive histopathology.
Presurgical Planning
Incorporating tractography in presurgical planning is beneficial for both neurosurgeon and patient as it improves defining the boundaries between eloquent areas and tumor, helping to formulate a patient-specific approach, thereby reducing the risk of postoperative neurological deficits. Studies have shown tractography aids in selecting the optimal surgical approach for corticectomy by comparing how potential access routes would impact WMT (56, 57). In planning the surgical approach, DDT provides the neurosurgeon with tumor proximity to WMT (58, 59), tumor impact on WMT (60), and extent of tumor invasion into the surrounding tissue (61, 62). Another improvement in surgical planning using DTI is the process of defining the resection boundary. Defining a resection boundary is a balance between maximally excising the lesion and preserving functional tissue. Here, altered WMT position and/or direction reflects displacement via tumor and decreased WMT density corresponds with vasogenic edema, tumor projections, or WMT destruction (63). DTI-defined tractography has also shown value in selecting patients for resective surgery, for example, removing patients with tumors found to be embedded in or in close proximity to functionally important regions (64). Furthermore, as methods and analyses continue to improve, DTI and DDT can aid in benefiting patients who are unable to undergo awake mapping and instead have surgeries under general anesthesia (21, 65).
Extent of Resection (EOR) and Surgical Outcome Prediction
DTI and DDT show promise in preoperatively predicting the EOR, giving patients a more informed prognosis of their surgical outcome. Aggressive resection of DTI-defined abnormalities demonstrated a greater chance of progression free and overall survival (66). In a different study, DDT showed that maintaining corticospinal tract (CST) integrity correlated with a higher chance of total EOR (67). A rating scale has also been introduced, accounting for several clinical and radiological factors to predict successful EOR. Ranging from 1 to 8, the scale analyzes tumor margins, imaging, symptoms, and volume, with values >4 indicative of successful EOR (68). DTI helped to validate this score and is an area of future study.
Preoperative and intraoperative DTI and DDT have been explored as a means to predict functional ability and recovery following tumor resection. Specifically, reconstructing eloquent pathways and indirectly measuring axonal health have produced schemes to predict the effects that resection can have on motor, language, and visual function. Postoperative motor deficits have been linked to lower FA averages and higher MD averages in the tumorous hemisphere CST (69), as well as distances ≤8 mm between tumor and CST (70). Preservation of arcuate fasciculus (AF) and superior longitudinal fasciculus (SLF) correlate to lack of long-term language dysfunction, while damage to these DTI-defined tracts showed short-term and potential long-term deficits (25). Also interhemispheric connectivity, determined preoperatively with DDT, has been regarded as a risk factor of surgery-related aphasia (71). Injury to optic radiation (OR) during surgery commonly results in postoperative visual field deficits (VFD) (72, 73). One study showed a linear relationship between VFD score and OR injury defined by tractography, which ultimately may be avoided through intraoperative visualization of the tract (74). Also, shorter distances between tumor and Meyer's loop (a subdivision of OR) has been linked to postoperative VFD (75, 76).
Intraoperative Applications of DTI
Intraoperative Use of Preoperative DT Images
Recent publications indicate that preoperative DDT may reduce postoperative neurological deficits through visualizing eloquent WMT intraoperatively. The general consensus in the literature concerning tumors near the CST is that DDT can aid in preserving functional tissue and results in positive post-surgical outcomes (15, 77, 78). However, validation may require a combination of methods such as assessing tract preservation when used in conjunction with another brain-mapping software, such as the NY Tract Finder. The NY Tract Finder is a 3D brain mapping method that electrically stimulates and identifies motor tracts, so that they can be preserved during surgery (79). Tumor resections in 40 patients were performed safely without damaging motor tracts or causing any postoperative neurological deficit using NY Tract Finder. DTI-defined CST has also been successfully used in other scenarios including assessment of CST projection from the lesioned motor cortex in children with unilateral spastic cerebral palsy (80). The authors reported DDT was able to identify the CST controlling the more pathologically affected hand and found to be consistent with transcranial magnetic stimulation (TMS) data.
Language tracts are particularly variable between patients, and thus they are difficult to precisely trace. When implemented in conjunction with navigated TMS (nTMS), DDT visualization during surgery aided in preserving speech for patients with tumors adjacent to the SLF (16, 81). Negwer et al. (81) found that when performing nTMS-based DDT, 76.0% of language fiber tracts, such as the SLF, were detected in the examined patients, as opposed to 39.9% when using the cubic ROI-based protocol. Out of 37 patients with left-sided perisylvian lesions, 22 patients (59.5%) received nTMS with 5 pulses of 5 Hz, 8 patients (21.6%) received 5 pulses of 7 Hz, and 7 patients (18.9%) received 7 pulses of 7 Hz. Compared with the nTMS-based DDT, the cubic ROI-based protocol displayed a better visualization of the AF (97.3% vs. 75.7%; p < 0.05) and of the SLF. The shorter language tracts, such as AF or the uncinate fascicle (UF), were visualized more effectively using the nTMS-based approach (p < 0.001); the UF was not detectable with the cubic ROIs-based protocol in either case. The fibers-to-tract ratio using nTMS-based DDT was 236 ± 73 and 286 ± 9 using the cubic ROIs-based protocol, which are comparable values and are both within the range Negwer et al. defined as ideal for a clear DDT result (fibers/tract 0–500) (81). The authors state that these results examining language-related DDT by nTMS still have to be confirmed by subcortical stimulation in future studies. This technique has also been used to aid in preserving motor function in patients with tumors adjacent to the CST in another study (82).
A “functional” approach based on preoperative fMRI and DTI scans in tandem with intraoperative neurophysiological monitoring shows promise as the current standard in the management of lesions in the dominant atrium (DA), a crossroad of eloquent WMT difficult to preserve with a standard “anatomical” approach (83). In a study consisting of asleep surgery in 43 patients, the combination of fMRI and DDT identified locations of the AF, inferior fronto-occipital fasciculus (IFOF), OR, and CST proximal to the DA resulting in gross total resections (< 2mm3 residual tumor) in 93.0% of patients and few postoperative deficits (9 transient, 3 permanent cases) (84).
Intraoperative DTI (IDTI)
Brain shift is known to affect the reliability of preoperatively generated DDT (85, 86). This hurdle can be reduced by updating tractography through intraoperative imaging. Image processing and fiber tract reconstruction can be completed in under 20 minutes (hence it is feasible to carry out DTI and DDT during surgery) and then integrated into navigational datasets (21, 87, 88). Marongiu et al. (88) directly compared postoperative outcomes of supratentorial glioblastoma resections accompanied by iDTI to those without accompaniment (due to MR equipment outage). For surgeries with iDTI, EOR was markedly higher (88.5% vs. 44%) and six-month progression free survival was greater as well (73.1% vs. 38.9%). Another benefit of increasing the reliability of tractography via iDTI, is performing more asleep surgeries. Research has shown an average of >95% resection in asleep resection near speech and language areas is possible using a combination of iDTI and fMRI (21).
That said, intraoperative imaging requires additional time and surgical preparation (89). A method to circumvent additional resources is by incorporating models compensating for intraoperative brain shift. Miga et al. (90) created a sparse-data-driven biomechanical model to predict physiological brain shift by accounting for typical deformation-inducing events such as cerebrospinal fluid drainage, hyperosmotic drugs, swelling, retraction, resection, and tumor cavity collapse. Their results are encouraging and await further comparisons that include: (1) detailed intraoperative MR validation, (2) workflow analysis, and (3) optimal forms of data visualization. Alternatively, Morin et al. (91) proposed an intraoperative ultrasound technique where a navigated ultrasound acquisition is performed directly in contact with the organ and doppler and B-mode images are recorded simultaneously, enabling the extraction of the blood vessels and probe footprint. Then, a constraint-based simulation registers the pre- and intraoperative vascular trees along with the cortical surface with the probe footprint. Finally, preoperative images are updated to provide the surgeon with current images of brain shape to navigate during resection.
Combining DDT and DES
Direct electrical stimulation (DES) is regarded as the gold standard for mapping brain function, though evidence suggests improvements are needed. While DES provides real-time, approximate spatial information about eloquent fibers—enhancing surgical performance and safety—it is time consuming and places the patient at risk for intraoperative seizures due to repeated direct electrical stimulation (92, 93). Moreover, DES has been shown to induce hemodynamic changes across large areas (77 sq. mm to 350 sq. mm) and the effect is difficult to predict as it depends on many local and remote physiological and morphological factors (94). It is misleading to assume DES allows us to draw unequivocal conclusions about the role of stimulated brain areas.
A collection of studies have demonstrated a direct relationship between DES current magnitude and the distance from probe to DTI-defined tracts; as the proximity to tracts increases, stimulation current decreases (22, 95–99). This relationship becomes more reliable when tract locations are updated via intraoperative imaging or ultrasonography (22, 86, 100–103). Figure 1 illustrates the general clustering of published DES current to DTI-defined tract distance correlations with respect to intraoperatively acquired images. Until verified models relating current-to-distance, the gold standard for mapping functionality may not be DES alone but DTI combined with DES. Combining these methods has been shown to be more effective at delineating motor pathways, yielding mean EOR values of 97.2 and 97.7% of cerebral glioma in eloquent areas (26, 100).
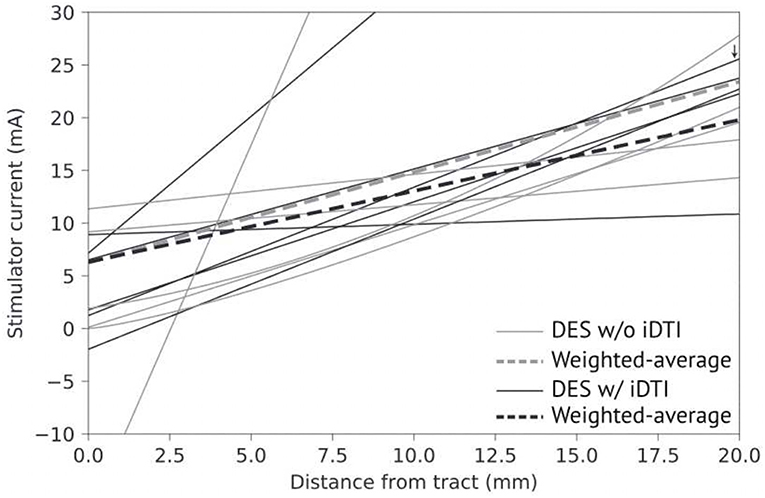
Figure 1. Comparison of DES current to distancefrom DDTfrom imaging performed intraoperatively (black) or pre/postoperatively (gray). Averages weighted by number of stimulation points divided by number of patients per study for both imaging methodologies (dash-lines). One publication studied the optic radiation (small arrow), all others studied the CST.
Further support for the efficacy of combining tractography and electrical stimulation (ES) was demonstrated in a study by Yamao et al. (104) evaluating cortico-cortical evoked potentials (CCEP) as a new intraoperative monitoring method of the dorsal language pathway. The preoperative AF tract can be dislocated or interrupted due to brain edema or infiltration of the tumor; tools like CCEP address this issue with the aid of preoperative fMRI and probabilistic tractography. When high-frequency ES to the floor of the resection cavity elicited language impairment (4 out of 21 patients), the distance between the subcortical stimulus site and the AF tract was within 5 mm. This illustrates DTT's value as a sophisticated neurosurgical approach for preserving language function. However, according to the authors, further studies are needed to establish a solid cut-off value to develop the CCEP monitoring as an efficient intraoperative method for preservation of the dorsal language pathway.
Improvements to DTI and DDT
Although DTI and DTT present many advantages and have demonstrated utility in approximating the geometry of WMT in the context of neurosurgical interventions, it is limited in its ability to resolve multiple fibers and is susceptible to partial volume effects (105). Progress in addressing these issues has been made by using alternative models to calculate diffusion properties, more complex image sequences acquired from additional magnetic field gradient directions and magnitudes, or a combination of the two.
Alternative models involve expanding the diffusion model to account for higher order diffusion features [e.g., diffusion kurtosis imaging (DKI)], substituting the tensor with another shape [e.g., constrained spherical convolution (CSD)], or modeling the Fourier transform relationship between the diffusion MR signals and the underlying diffusion displacement [e.g., generalized Q-ball imaging (GQI)]. While models like DKI and CSD require more extensive image sets, improvements to DDT are possible by applying models other than DTI. For example, Zhang et al. (106) demonstrated CSTs passing through areas of edema could be visualized with the GQI model but not DTI (106).
Advanced diffusion imaging sequences include diffusion spectrum imaging (DSI), high angular resolution diffusion imaging (HARDI), DKI, and Crossing Fiber Angular Resolution Intra-voxel structure (CFARI). Tractography based on DSI has the capacity to image crossing fibers in neural tissue using probability density functions, which specify microscopic displacements of MR-visible spins using 3D Fourier encoding of displacements. Unlike DTI, DSI is able to resolve crossing fibers, accurately reconstruct confluence of pathways, and demonstrate the decussation of fibers in the optic chiasm (107). In contrast, HARDI constructs maps approximate the diffusion orientation density functions. Caverzasi et al. (25) demonstrated that combining HARDI and Q-ball imaging improved the resolution of tractography in regions with crossing fiber populations, presenting more complex structures than DDT. During a study of 35 patients with gliomas, they found that preservation of the left AF and the temporoparietal component of the SLF (SLF-tp) was consistent in patients without language deficits at the long-term follow-up after glioma resection. The AF and SLF-tp were affected in patients with short-term language deficit, and predictive of long-term deficits. The authors concluded that the lack of postoperative deficits supports intraoperative use of language tracts. More advanced image sequences that calculate diffusion magnitudes and directions involving complex distributions of intravoxel fiber orientation are available. Across the board, these techniques are based on improving resolution by acquiring more images from more magnetic field gradient directions.
Overall, novel applications of DTI and DDT have demonstrated that preoperative, non-invasive mapping of eloquent cortical regions and functionally relevant WMT and their use with other intraoperative adjuncts like DES and nTMS help to reduce postoperative deficits, improving quality of life and overall survival in glioma patients. Future more advanced imaging sequences will continue to improve the utility of WMT depiction in neurosurgery, improving functional outcomes in glioma patients.
Author Contributions
JC devised the structure and focus of the article, wrote a significant portion of it, and managed the manuscript through its composition and publication processes. EA contributed to the publication by writing and editing portions of the intraoperative and limitations sections. SD contributed to the publication by writing and editing portions of the preoperative section. JT and DO were equally important to crafting the manuscript’s focus and editing of the article. JT and DO share senior authorship.
Funding
This work was funded by Synaptive Medical (industry, fellowship donation) and by Cancer League of Colorado, Inc. (183430-DO).
Conflict of Interest Statement
The authors declare that the research was conducted in the absence of any commercial or financial relationships that could be construed as a potential conflict of interest.
References
1. Mut M. Surgical treatment of brain metastasis: a review. Clin Neurol Neurosurg. (2011) 114:1–8. doi: 10.1016/j.clineuro.2011.10.013
2. Pallud J, Taillandier L, Capelle L, Fontaine D, Peyre M, Ducray F, et al. Quantitative morphological magnetic resonance imaging follow-up of low-grade glioma: a plea for systematic measurement of growth rates. Neurosurgery. (2012) 71:729–39; discussion 739–40. doi: 10.1227/NEU.0b013e31826213de
3. Bloch O, Han SJ, Cha S, Sun MZ, Aghi MK, McDermott MW, et al. Impact of extent of resection for recurrent glioblastoma on overall survival. J Neurosurg. (2012) 117:1032–8. doi: 10.3171/2012.9.JNS12504
4. Ius T, Isola M, Budai R, Pauletto G, Tomasino B, Fadiga L, et al. Low-grade glioma surgery in eloquent areas: volumetric analysis of extent of resection and its impact on overall survival. a single-institution experience in 190 patients: clinical article. J Neurosurg. (2012) 117:1039–52. doi: 10.3171/2012.8.JNS12393
5. Chaichana KL, Zadnik P, Weingart JD, Olivi A, Gallia GL, Blakeley J, et al. Multiple resections for patients with glioblastoma: prolonging survival. J Neurosurg. (2013) 118:812–20. doi: 10.3171/2012.9.JNS1277
6. Hervey-Jumper SL, Berger MS. Role of surgical resection in low-and high-grade gliomas. Curr Treat Options Neurol. (2014) 16:284. doi: 10.1007/s11940-014-0284-7
7. Sanai N, Polley M-Y, McDermott MW, Parsa AT, Berger MS. An extent of resection threshold for newly diagnosed glioblastomas. J Neurosurg. (2011) 115:3–8. doi: 10.3171/2011.7.JNS10238
8. Sanai N, Berger MS. Extent of resection influences outcomes for patients with gliomas. Rev Neurol. (2011) 167:648–654. doi: 10.1016/j.neurol.2011.07.004
9. Kinoshita M, Yamada K, Hashimoto N, Kato A, Izumoto S, Baba T, et al. Fiber-tracking does not accurately estimate size of fiber bundle in pathological condition: initial neurosurgical experience using neuronavigation and subcortical white matter stimulation. Neuroimage. (2005) 25:424–9. doi: 10.1016/J.NEUROIMAGE.2004.07.076
10. Rubinstein, LJ, Russel D. Pathology of tumours of the nervous system. London: Edward Arnold (1989). p. 481–3.
11. Glantz MJ, Burger PC, Herndon JE, Friedman AH, Cairncross JG, Vick NA, et al. Influence of the type of surgery on the histologic diagnosis in patients with anaplastic gliomas. Neurology. (1991) 41:1741–4. doi: 10.1212/WNL.41.11.1741
12. Chang P, Grinband J, Weinberg BD, Bardis M, Khy M, Cadena G, et al. Deep-learning convolutional neural networks accurately classify genetic mutations in gliomas. Am J Neuroradiol. (2018) 39:1201–7. doi: 10.3174/ajnr.A5667
13. Verma N, Cowperthwaite MC, Burnett MG, Markey MK. Differentiating tumor recurrence from treatment necrosis: a review of neuro-oncologic imaging strategies. Neuro Oncol. (2013) 15:515–34. doi: 10.1093/neuonc/nos307
14. Pouratian N, Cannestra AF, Bookheimer SY, Martin NA, Toga AW. Variability of intraoperative electrocortical stimulation mapping parameters across and within individuals. J Neurosurg. (2004) 101:458–66. doi: 10.3171/jns.2004.101.3.0458
15. Vassal F, Schneider F, Nuti C. Intraoperative use of diffusion tensor imaging-based tractography for resection of gliomas located near the pyramidal tract: comparison with subcortical stimulation mapping and contribution to surgical outcomes. Br J Neurosurg. (2013) 27:668–75. doi: 10.3109/02688697.2013.771730
16. Sollmann N, Kelm A, Ille S, Schröder A, Zimmer C, Ringel F, et al. Setup presentation and clinical outcome analysis of treating highly language-eloquent gliomas via preoperative navigated transcranial magnetic stimulation and tractography. Neurosurg Focus. (2018) 44:E2. doi: 10.3171/2018.3.focus1838
17. Essayed WI, Zhang F, Unadkat P, Cosgrove GR, Golby AJ, O'Donnell LJ. White matter tractography for neurosurgical planning: a topography-based review of the current state of the art. NeuroImage Clin. (2017) 15:659–672. doi: 10.1016/j.nicl.2017.06.011
18. Farshidfar Z, Faeghi F, Mohseni M, Seddighi A, Kharrazi HH, Abdolmohammadi J. Diffusion tensor tractography in the presurgical assessment of cerebral gliomas. Neuroradiol J. (2014) 27:75–84. doi: 10.15274/NRJ-2014-10008
19. Caverzasi E, Papinutto N, Castellano A, Zhu AH, Scifo P, Riva M, et al. Neurite orientation dispersion and density imaging color maps to characterize brain diffusion in neurologic disorders. J Neuroimag. (2016) 26:494–8. doi: 10.1111/jon.12359
20. Jones TL, Byrnes TJ, Yang G, Howe FA, Bell BA, Barrick TR. Brain tumor classification using the diffusion tensor image segmentation (D-SEG) technique. Neuro Oncol. (2015) 17:466–76. doi: 10.1093/neuonc/nou159
21. D'Andrea G, Familiari P, Di Lauro A, Angelini A, Sessa G. Safe resection of gliomas of the dominant angular gyrus availing of preoperative fmri and intraoperative dti: preliminary series and surgical technique. World Neurosurg. (2016) 87:627–39. doi: 10.1016/j.wneu.2015.10.076
22. Ostrý S, Belšan T, Otáhal J, Beneš V, Netuka D. Is intraoperative diffusion tensor imaging at 3.0T comparable to subcortical corticospinal tract mapping? Neurosurgery. (2013) 73:797–807. doi: 10.1227/NEU.0000000000000087
23. Barbosa BJAP, Dimostheni A, Teixeira MJ, Tatagiba M, Lepski G. Insular gliomas and the role of intraoperative assistive technologies: results from a volumetry-based retrospective cohort. Clin Neurol Neurosurg. (2016) 149:104–10. doi: 10.1016/j.clineuro.2016.08.001
24. Meyer EJ, Gaggl W, Gilloon B, Swan B, Greenstein M, Voss J, et al. The impact of intracranial tumor proximity to white matter tracts on morbidity and mortality: A retrospective diffusion tensor imaging study. Neurosurgery. (2017) 80:193–200. doi: 10.1093/neuros/nyw040
25. Caverzasi E, Hervey-Jumper SL, Jordan KM, Lobach IV, Li J, et al. Identifying preoperative language tracts and predicting postoperative functional recovery using HARDI q-ball fiber tractography in patients with gliomas. J Neurosurg. (2015) 125:33–45. doi: 10.3171/2015.6.jns142203
26. Zhu FP, Wu JS, Song YY, Yao CJ, Zhuang DX, Xu G, et al. Clinical application of motor pathway mapping using diffusion tensor imaging tractography and intraoperative direct subcortical stimulation in cerebral glioma surgery: a prospective cohort study. Neurosurgery. (2012) 71:1170–83. doi: 10.1227/NEU.0b013e318271bc61
27. Giese A, Westphal M. Treatment of malignant glioma: a problem beyond the margins of resection. J Cancer Res Clin Oncol. (2001) 127:217–25. doi: 10.1007/s004320000188
28. O'Neill BP, Buckner JC, Coffey RJ, Dinapoli RP, Shaw EG. Brain metastatic lesions. Mayo Clin Proc. (1994) 69:1062–8.
29. Sugahara T, Korogi Y, Kochi M, Ikushima I, Shigematu Y, Hirai T, et al. Usefulness of diffusion-weighted MRI with echo-planar technique in the evaluation of cellularity in gliomas. J Magn Reson Imaging. (1999) 9:53–60. doi: 10.1002/(SICI)1522-2586(199901)9:1<53::AID-JMRI7>3.0.CO;2-2
30. Yan H, Parsons DW, Jin G, McLendon R, Rasheed BA, Yuan W, et al. IDH1 and IDH2 Mutations in Gliomas. N Engl J Med. (2009) 360:765–73. doi: 10.1056/NEJMoa0808710
31. Yamasaki F, Kurisu K, Satoh K, Arita K, Sugiyama K, Ohtaki M, et al. Apparent diffusion coefficient of human brain tumors at MR imaging. Radiology. (2005) 235:985–91. doi: 10.1148/radiol.2353031338
32. Villanueva-Meyer JE, Mabray MC, Cha S. Current clinical brain tumor imaging. Clin Neurosurg. (2017) 81:397–415. doi: 10.1093/neuros/nyx103
33. Basser PJ. Inferring microstructural features and the physiological state of tissues from diffusion-weighted images. NMR Biomed. (1995) 8:333–44. doi: 10.1002/nbm.1940080707
34. Pierpaoli C, Jezzard P, Basser PJ, Barnett A, Di Chiro G. Diffusion tensor MR imaging of the human brain. Radiology. (1996) 201:637–48. doi: 10.1148/radiology.201.3.8939209
35. Song SK, Sun SW, Ramsbottom MJ, Chang C, Russell J, Cross AH. Dysmyelination revealed through MRI as increased radial (but unchanged axial) diffusion of water. Neuroimage. (2002) 17:1429–36. doi: 10.1006/nimg.2002.1267
36. Beppu T, Inoue T, Shibata Y, Yamada N, Kurose A, Ogasawara K, et al. Fractional anisotropy value by diffusion tensor magnetic resonance imaging as a predictor of cell density and proliferation activity of glioblastomas. Surg Neurol. (2005) 63:56–61. doi: 10.1016/j.surneu.2004.02.034
37. Pavlisa G, Rados M, Pavlisa G, Pavic L, Potocki K, Mayer D. The differences of water diffusion between brain tissue infiltrated by tumor and peritumoral vasogenic edema. Clin Imag. (2009) 33:96–101. doi: 10.1016/j.clinimag.2008.06.035
38. Ormond DR, Souza SD', Thompson JA. Global and targeted pathway impact of gliomas on white matter integrity based on lobar localization. Cureus. (2017) 9:1660. doi: 10.7759/cureus.1660
39. Lu S, Ahn D, Johnson G, Cha S. Peritumoral diffusion tensor imaging of high-grade gliomas and metastatic brain tumors. Am J Neuroradiol. (2003) 24:937–41.
40. Stadlbauer A, Ganslandt O, Buslei R, Hammen T, Gruber S, Moser, et al. Gliomas: histopathologic evaluation of changes in directionality and magnitude of water diffusion at diffusion-tensor MR imaging. Radiology. (2006) 240:803–10. doi: 10.1148/radiol.2403050937
41. El-Serougy LG, Abdel Razek AAK, Mousa AE, Eldawoody HAF, El-Morsy AEME. Differentiation between high-grade gliomas and metastatic brain tumors using Diffusion Tensor Imaging metrics. Egypt J Radiol Nucl Med. (2015) 46:1099–104. doi: 10.1016/j.ejrnm.2015.08.005
42. Tan Y, Wang XC, Zhang H, Wang J, Qin JB, Wu XF, et al. Differentiation of high-grade-astrocytomas from solitary-brain-metastases: Comparing diffusion kurtosis imaging and diffusion tensor imaging. Eur J Radiol. (2015) 84:2618–24. doi: 10.1016/j.ejrad.2015.10.007
43. Bette S, Huber T, Wiestler B, Boeckh-Behrens T, Gempt J, Ringel F, et al. Analysis of fractional anisotropy facilitates differentiation of glioblastoma and brain metastases in a clinical setting. Eur J Radiol. (2016) 85:2182–7. doi: 10.1016/j.ejrad.2016.10.002
44. Byrnes TJD, Barrick TR, Bell BA, Clark CA. Diffusion tensor imaging discriminates between glioblastoma and cerebral metastases in vivo. NMR Biomed. (2011) 24:54–60. doi: 10.1002/nbm.1555
45. Wang S, Kim S, Chawla S, Wolf RL, Knipp DE, Vossough A, et al. Differentiation between glioblastomas, solitary brain metastases, and primary cerebral lymphomas using diffusion tensor and dynamic susceptibility contrast-enhanced MR imaging. Am J Neuroradiol. (2011) 32:507–14. doi: 10.3174/ajnr.A2333
46. Papageorgiou TS, Chourmouzi D, Drevelengas A, Kouskouras K, Siountas A. Diffusion Tensor Imaging in brain tumors: a study on gliomas and metastases. Phys Medica. (2015) 31:767–73. doi: 10.1016/j.ejmp.2015.03.010
47. Toh CH, Wei KC, Ng SH, Wan YL, Lin CP, Castillo M. Differentiation of brain abscesses from necrotic glioblastomas and cystic metastatic brain tumors with diffusion tensor imaging. Am J Neuroradiol. (2011) 32:1646–51. doi: 10.3174/ajnr.A2581
48. Wang S, Kim S, Chawla S, Wolf RL, Zhang WG, O'Rourke DM, et al. Differentiation between glioblastomas and solitary brain metastases using diffusion tensor imaging. Neuroimage. (2009) 44:653–660. doi: 10.1016/j.neuroimage.2008.09.027
49. Wang S, Kim SJ, Poptani H, Woo JH, Mohan S, Jin R, et al. Diagnostic utility of diffusion tensor imaging in differentiating glioblastomas from brain metastases. Am J Neuroradiol. (2014) 39:928–34. doi: 10.3174/ajnr.A3871
50. Svolos P, Tsolaki E, Kapsalaki E, Theodorou K, Fountas K, Fezoulidis I, et al. Investigating brain tumor differentiation with diffusion and perfusion metrics at 3T MRI using pattern recognition techniques. Magn Reson Imag. (2013) 31:1567–77. doi: 10.1016/j.mri.2013.06.010
51. Bauer AH, Erly W, Moser FG, Maya M, Nael K. Differentiation of solitary brain metastasis from glioblastoma multiforme: a predictive multiparametric approach using combined MR diffusion and perfusion. Neuroradiology. (2015) 57:697–703. doi: 10.1007/s00234-015-1524-6
52. Tsougos I, Svolos P, Kousi E, Fountas K, Theodorou K, Fezoulidis I, et al. Differentiation of glioblastoma multiforme from metastatic brain tumor using proton magnetic resonance spectroscopy, diffusion and perfusion metrics at 3 T. Cancer Imag. (2012) 12:423–36. doi: 10.1102/1470-7330.2012.0038
53. Lu S, Ahn D, Johnson G, Law M, Zagzag D, Grossman RI. Diffusion-Tensor MR imaging of intracranial neoplasia and associated peritumoral edema: introduction of the tumor infiltration index. Radiology. (2004) 232:221–8. doi: 10.1148/radiol.2321030653
54. Jiang R, Du F-Z, He C, Gu M, Ke Z-W, Li J-H. The value of diffusion tensor imaging in differentiating high-grade gliomas from brain metastases: a systematic review and meta-analysis. PLoS ONE. (2014) 9:e0112550. doi: 10.1371/journal.pone.0112550
55. Chen R, Wang S, Poptani H, Melhem ER, Herskovits EH. A Bayesian diagnostic system to differentiate glioblastomas from solitary brain metastases. Neuroradiol J. (2013) 26:175–83. doi: 10.1177/197140091302600207
56. Romano NA, Minniti AG, Mastronardi L, Ferrante L, Fantozzi LM, Bozzao A. Pre-surgical planning and MR-tractography utility in brain tumour resection. Eur Radiol. (2009) 19:2798–808. doi: 10.1007/s00330-009-1483-6
57. Zakaria H, Haider S, Lee I. Automated whole brain tractography affects preoperative surgical decision making. Cureus. (2017) 9:e1656. doi: 10.7759/cureus.1656
58. D'Andrea G, Trillo' G, Picotti V, Raco A. “Functional magnetic resonance imaging (fMRI), pre-intraoperative tractography in neurosurgery: the experience of Sant'Andrea Rome University Hospital,” in Acta Neurochirurgica Supplement. 241–50. doi: 10.1007/978-3-319-39546-3_36
59. Sunaert S. Presurgical planning for tumor resectioning. J Magn Reson Imag. (2006) 23:887–905. doi: 10.1002/jmri.20582
60. Celtikci E, Celtikci P, Fernandes-Cabral DT, Ucar M, Fernandez-Miranda JC, Borcek AO. High-definition fiber tractography in evaluation and surgical planning of thalamopeduncular pilocytic astrocytomas in pediatric population: case series and review of literature. World Neurosurg. (2017) 98:463–9. doi: 10.1016/j.wneu.2016.11.061
61. Yu CS, Li KC, Xuan Y, Ji XM, Qin W. Diffusion tensor tractography in patients with cerebral tumors: a helpful technique for neurosurgical planning and postoperative assessment. Eur J Radiol. (2005) 56:197–204. doi: 10.1016/J.EJRAD.2005.04.010
62. Dimou S, Battisti RA, Hermens DF, Lagopoulos J. A systematic review of functional magnetic resonance imaging and diffusion tensor imaging modalities used in presurgical planning of brain tumour resection. Neurosurg Rev. (2013) 36:205–14. doi: 10.1007/s10143-012-0436-8
63. Jellison BJ, Field AS, Medow J, Lazar M, Salamat MS, Alexander AL. Diffusion tensor imaging of cerebral white matter: a pictorial review of physics, fiber tract anatomy, and tumor imaging patterns. AJNR Am J Neuroradiol. (2004) 25:356–69.
64. Berntsen EM, Gulati S, Solheim O, Kvistad KA, Torp SH, Selbekk T, et al. Functional magnetic resonance imaging and diffusion tensor tractography incorporated into an intraoperative 3-dimensional ultrasound-based neuronavigation system: impact on therapeutic strategies, extent of resection, and clinical outcome. Neurosurgery. (2010) 67:251–64. doi: 10.1227/01.NEU.0000371731.20246.AC
65. Soni N, Mehrotra A, Behari S, Kumar S, Gupta N. Diffusion-tensor Imaging and Tractography Application in Pre-operative Planning of Intra-axial Brain Lesions. Cureus. (2017) 9:e1739 doi: 10.7759/cureus.1739
66. Yan J, van der Hoorn A, Larkin timothy J, Boonzaier NR, Matys T, Price stephen J. Extent of resection of peritumoral diffusion tensor imaging–detected abnormality as a predictor of survival in adult glioblastoma patients. J Neurosurg. (2017) 126:234–41. doi: 10.3171/2016.1.JNS152153
67. Castellano A, Bello L, Michelozzi C, Gallucci M, Fava E, Iadanza A, et al. Role of diffusion tensor magnetic resonance tractography in predicting the extent of resection in glioma surgery. Neuro Oncol. (2012) 14:192–202. doi: 10.1093/neuonc/nor188
68. Spena G, D'Agata F, Panciani PP, Buttolo L, di Monale Bastia MB, Fontanella MM. Practical prognostic score for predicting the extent of resection and neurological outcome of gliomas in the sensorimotor area. Clin Neurol Neurosurg. (2018) 164:25–31. doi: 10.1016/j.clineuro.2017.11.009
69. Rosenstock T, Giampiccolo D, Schneider H, Runge SJ, Bährend I, Vajkoczy P, et al. Specific DTI seeding and diffusivity-analysis improve the quality and prognostic value of TMS-based deterministic DTI of the pyramidal tract. NeuroImage Clin. (2017) 16:276–85. doi: 10.1016/J.NICL.2017.08.010
70. Rosenstock T, Grittner U, Acker G, Schwarzer V, Kulchytska N, Vajkoczy P, et al. Risk stratification in motor area-related glioma surgery based on navigated transcranial magnetic stimulation data. J Neurosurg. (2016) 126:1–11. doi: 10.3171/2016.4.JNS152896
71. Sollmann N, Negwer C, Tussis L, Hauck T, Ille S, Maurer S, et al. Interhemispheric connectivity revealed by diffusion tensor imaging fiber tracking derived from navigated transcranial magnetic stimulation maps as a sign of language function at risk in patients with brain tumors. J Neurosurg. (2016) 126:222–33. doi: 10.3171/2016.1.jns152053
72. Anderson DR, Trobe JD, Hood TW, Gebarski SS. Optic tract injury after anterior temporal lobectomy. Ophthalmology. (1989) 96:1065–70. doi: 10.1016/S0161-6420(89)32783-7
73. Krolak-Salmon P, Guenot M, Tiliket C, Isnard J, Sindou M, Mauguiere F, et al. Anatomy of optic nerve radiations as assessed by static perimetry and MRI after tailored temporal lobectomy. Br J Ophthalmol. (2000) 84:884–9. doi: 10.1136/bjo.84.8.884
74. Chen X, Weigel D, Ganslandt O, Buchfelder M, Nimsky C. Prediction of visual field deficits by diffusion tensor imaging in temporal lobe epilepsy surgery. Neuroimage. (2009) 45:286–97. doi: 10.1016/j.neuroimage.2008.11.038
75. James JS, Radhakrishnan A, Thomas B, Madhusoodanan M, Kesavadas C, Abraham M, et al. Diffusion tensor imaging tractography of Meyer's loop in planning resective surgery for drug-resistant temporal lobe epilepsy. Epilepsy Res. (2015) 110:95–104. doi: 10.1016/j.eplepsyres.2014.11.020
76. Taoka T, Sakamoto M, Nakagawa H, Nakase H, Iwasaki S, Takayama K, et al. Diffusion tensor tractography of the Meyer loop in cases of temporal lobe resection for temporal lobe epilepsy: correlation between postsurgical visual field defect and anterior limit of Meyer loop on tractography. Am J Neuroradiol. (2008) 29:1329–34. doi: 10.3174/ajnr.A1101
77. Bello L, Gambini A, Castellano A, Carrabba G, Acerbi F, Fava E, et al. Motor and language DTI Fiber Tracking combined with intraoperative subcortical mapping for surgical removal of gliomas. Neuroimage. (2008) 39:369–82. doi: 10.1016/j.neuroimage.2007.08.031
78. Bello L, Castellano A, Fava E, Casaceli G, Riva M, Scotti G, et al. Intraoperative use of diffusion tensor imaging fiber tractography and subcortical mapping for resection of gliomas: technical considerations. Neurosurg Focus. (2010) 28:E6. doi: 10.3171/2009.12.FOCUS09240
79. Yamaguchi F, Ten H, Higuchi T, Omura T, Kojima T, Adachi K, et al. An intraoperative motor tract positioning method in brain tumor surgery: technical note. J Neurosurg. (2017) 129:576–82. doi: 10.3171/2017.5.JNS162978
80. Kuo H-C, Ferre CL, Carmel JB, Gowatsky JL, Stanford AD, Rowny SB, et al. Using diffusion tensor imaging to identify corticospinal tract projection patterns in children with unilateral spastic cerebral palsy. Dev Med Child Neurol. (2017) 59:65–71. doi: 10.1111/dmcn.13192
81. Negwer C, Sollmann N, Ille S, Hauck T, Maurer S, Kirschke JS, et al. Language pathway tracking: comparing nTMS-based DTI fiber tracking with a cubic ROIs-based protocol. J Neurosurg. (2016) 126:1006–14. doi: 10.3171/2016.2.jns152382
82. Sollmann N, Wildschuetz N, Kelm A, Conway N, Moser T, Bulubas L, et al. Associations between clinical outcome and navigated transcranial magnetic stimulation characteristics in patients with motor-eloquent brain lesions: a combined navigated transcranial magnetic stimulation–diffusion tensor imaging fiber tracking approach. J Neurosurg. (2017) 128:800–10. doi: 10.3171/2016.11.jns162322
83. Walter J, Kuhn SA, Waschke A, Kalff R, Ewald C. Operative treatment of subcortical metastatic tumours in the central region. J Neurooncol. (2011) 103:567–573. doi: 10.1007/s11060-010-0420-5
84. Frati A, Pesce A, D'Andrea G, Fraschetti F, Salvati M, Cimatti M, et al. A purely functional Imaging based approach for transcortical resection of lesion involving the dominant atrium: toward safer, imaging-guided, tailored cortico-leucotomies. J Clin Neurosci. (2018) 50:252–61. doi: 10.1016/j.jocn.2018.01.045
85. Nimsky C, Ganslandt O, Merhof D, Sorensen AG, Fahlbusch R. Intraoperative visualization of the pyramidal tract by diffusion-tensor-imaging-based fiber tracking. Neuroimage. (2006) 30:1219–29. doi: 10.1016/J.NEUROIMAGE.2005.11.001
86. Maesawa S, Fujii M, Nakahara N, Watanabe T, Wakabayashi T, Yoshida J. Intraoperative tractography and motor evoked potential (MEP) monitoring in surgery for gliomas around the corticospinal tract. World Neurosurg. (2010) 74:153–161. doi: 10.1016/j.wneu.2010.03.022
87. Nimsky C, Ganslandt O, Hastreiter P, Wang R, Benner T, Sorensen AG, et al. Preoperative and intraoperative diffusion tensor imaging-based fiber tracking in glioma surgery. Neurosurgery. (2005) 56:130–8. doi: 10.1227/01.NEU.0000144842.18771.30
88. Marongiu A, D'Andrea G, Raco A. 1.5-T Field Intraoperative Magnetic Resonance Imaging Improves Extent of Resection and Survival in Glioblastoma Removal. World Neurosurg. (2017) 98:578–86. doi: 10.1016/j.wneu.2016.11.013
89. Nimsky C, Grummich P, Sorensen AG, Fahlbusch R, Ganslandt O, Nimsky CC. Visualization of the pyramidal tract in glioma surgery by integrating diffusion tensor imaging in functional neuronavigation intraoperative darstellung der pyramidenbahn in der gliomchirurgie durch integration der diffusions-tensor-bildgebung in funktione. Bibliogr Zentralbl Neurochir. (2005) 66:133–41. doi: 10.1055/s-2005-836606
90. Miga MI, Sun K, Chen I, Clements LW, Pheiffer TS, Simpson AL, et al. Clinical evaluation of a model-updated image-guidance approach to brain shift compensation: experience in 16 cases. Int J Comput Assist Radiol Surg. (2016) 11:1467–74. doi: 10.1007/s11548-015-1295-x
91. Morin F, Courtecuisse H, Reinertsen I, Le Lann F, Palombi O, Payan Y, et al. Brain-shift compensation using intraoperative ultrasound and constraint-based biomechanical simulation. Med Image Anal. (2017) 40:133–53. doi: 10.1016/j.media.2017.06.003
92. Duffau H. Contribution of cortical and subcortical electrostimulation in brain glioma surgery: Methodological and functional considerations. Neurophysiol Clin. (2007) 37:373–82. doi: 10.1016/j.neucli.2007.09.003
93. Szelényi A, Bello L, Duffau H, Fava E, Feigl GC, Galanda M, et al. Intraoperative electrical stimulation in awake craniotomy: methodological aspects of current practice. Neurosurg Focus. (2010) 28:E7. doi: 10.3171/2009.12.FOCUS09237
94. Borchers S, Himmelbach M, Logothetis N, Karnath H-O. Direct electrical stimulation of human cortex — the gold standard for mapping brain functions? Nat Rev Neurosci. (2011) 13:63–70. doi: 10.1038/nrn3140
95. Zolal A, Hejčl A, Vachata P, Bartoš R, Humhej I, Malucelli A, et al. The use of diffusion tensor images of the corticospinal tract in intrinsic brain tumor surgery: a comparison with direct subcortical stimulation. Neurosurgery. (2012) 71:331–340. doi: 10.1227/NEU.0b013e31825b1c18
96. Kamada K, Todo T, Ota T, Ino K, Masutani Y, Aoki S, et al. The motor-evoked potential threshold evaluated by tractography and electrical stimulation. J Neurosurg. (2009) 111:785–95. doi: 10.3171/2008.9.JNS08414
97. Zhuang DX, Wu JS, Yao CJ, Qiu TM, Lu JF, Zhu FP, et al. Intraoperative multi-information-guided resection of dominant-sided insular gliomas in a 3-t intraoperative magnetic resonance imaging integrated neurosurgical suite. World Neurosurg. (2016) 89:84–92. doi: 10.1016/j.wneu.2016.01.067
98. Ohue S, Kohno S, Inoue A, Yamashita D, Harada H, Kumon Y, et al. Accuracy of diffusion tensor magnetic resonance imaging-based tractography for surgery of gliomas near the pyramidal tract. Neurosurgery. (2012) 70:283–94. doi: 10.1227/NEU.0b013e31823020e6
99. Shiban E, Krieg SM, Haller B, Buchmann N, Obermueller T, Boeckh-Behrens T, et al. Intraoperative subcortical motor evoked potential stimulation: how close is the corticospinal tract? J Neurosurg. (2015) 123:711–20. doi: 10.3171/2014.10.JNS141289
100. Javadi SA, Nabavi A, Giordano M, Faghihzadeh E, Samii A. Evaluation of diffusion tensor imaging–based tractography of the corticospinal tract: a correlative study with intraoperative magneticresonance imaging and direct electrical subcortical stimulation. Neurosurgery. (2017) 80:287–99. doi: 10.1227/NEU.0000000000001347
101. Prabhu SS, Gasco J, Tummala S, Weinberg JS, Rao G. Intraoperative magnetic resonance imaging–guided tractography with integrated monopolar subcortical functional mapping for resection of brain tumors. J Neurosurg. (2011) 114:719–26. doi: 10.3171/2010.9.JNS10481
102. Nossek E, Korn A, Shahar T, Kanner AA, Yaffe H, Marcovici D, et al. Intraoperative mapping and monitoring of the corticospinal tracts with neurophysiological assessment and 3-dimensional ultrasonography-based navigation. J Neurosurg. (2011) 114:738–46. doi: 10.3171/2010.8.JNS10639
103. Shahar T, Korn A, Barkay G, Biron T, Hadanny A, Gazit T, et al. Elaborate mapping of the posterior visual pathway in awake craniotomy. J Neurosurg. (2017) 128:1–9. doi: 10.3171/2017.2.JNS162757
104. Yamao Y, Suzuki K, Kunieda T, Matsumoto R, Arakawa Y, Nakae T, et al. Clinical impact of intraoperative CCEP monitoring in evaluating the dorsal language white matter pathway. Hum Brain Mapp. (2017) 38:1977–91. doi: 10.1002/hbm.23498
105. Abhinav K, Yeh F-C, Mansouri A, Zadeh G, Fernandez-Miranda JC. High-definition fiber tractography for the evaluation of perilesional white matter tracts in high-grade glioma surgery. Neuro Oncol. (2015) 17:nov113. doi: 10.1093/neuonc/nov113
106. Zhang H, Wang Y, Lu T, Qiu B, Tang Y, Ou S, et al. Differences between generalized q-sampling imaging and diffusion tensor imaging in the preoperative visualization of the nerve fiber tracts within peritumoral edema in brain. Neurosurgery. (2013) 73:1044–53; discussion 1053. doi: 10.1227/NEU.0000000000000146
Keywords: diffusion tensor imaging, tractography, glioma, resection, intracranial electrical stimulation
Citation: Costabile JD, Alaswad E, D’Souza S, Thompson JA and Ormond DR (2019) Current Applications of Diffusion Tensor Imaging and Tractography in Intracranial Tumor Resection. Front. Oncol. 9:426. doi: 10.3389/fonc.2019.00426
Received: 11 November 2018; Accepted: 07 May 2019;
Published: 29 May 2019.
Edited by:
Sandro M. Krieg, Technische Universität München, GermanyReviewed by:
Miriam H. A. Bopp, University of Marburg, GermanySujit Prabhu, University of Texas MD Anderson Cancer Center, United States
Copyright © 2019 Costabile, Alaswad, D’Souza, Thompson and Ormond. This is an open-access article distributed under the terms of the Creative Commons Attribution License (CC BY). The use, distribution or reproduction in other forums is permitted, provided the original author(s) and the copyright owner(s) are credited and that the original publication in this journal is cited, in accordance with accepted academic practice. No use, distribution or reproduction is permitted which does not comply with these terms.
*Correspondence: Jamie D. Costabile, jamie.costabile@ucdenver.edu
†These authors have contributed equally to this work