Abstract
Objective. Central and obstructive apneas are sources of morbidity and mortality associated with primary patient conditions as well as secondary to medical care such as sedation/analgesia in post-operative patients. This research investigates the predictive value of the respirophasic variation in the noninvasive photoplethysmography (PPG) waveform signal in detecting airway obstruction. Methods. PPG data from 20 consenting healthy adults (12 male, 8 female) undergoing anesthesia were collected directly after surgery and before transfer to the Post Anesthesia Care Unit (PACU). Features of the PPG waveform were calculated and used in a neural network to classify normal and obstructive events. Results. During the postoperative period studied, the neural network classifier yielded an average (±standard deviation) 75.4 (±3.7)% sensitivity, 91.6 (±2.3)% specificity, 84.7 (±3.5)% positive predictive value, 85.9 (±1.8)% negative predictive value, and an overall accuracy of 85.4 (±2.0)%. Conclusions. The accuracy of this method shows promise for use in real-time monitoring situations.
Similar content being viewed by others
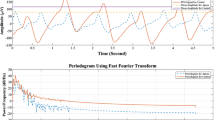
References
Gravestein J. Let no patient be harmed by anesthesia. APSF Newslett 2000; 14: 44–46
Joint Commission on Accreditation of Healthcare Organizations. Guide to Hospital Accreditation Resources. Department of Anesthesiology, 1995; 16–17.
Practice guidelines for sedation and analgesia by non-anesthesiologists. A report by the American Society of Anesthesiologists Task Force on Sedation and Analgesia by Non-Anesthesiologists. Anesthesiology 1996; 84(2): 459–471.
Hudson-Civetta J. 1989 Allocating nursing care. In: Civetta J, Taylor R, Kirby R eds. Introduction to critical care. Philadelphia: J.B. Lippincott
Varady P, Bongar S, Benyo Z. 2003 Detection of airway obstructions and sleep apnea by analyzing the phase relation of respiration movement signals. IEEE Trans Instrum Meas 52:2–6
Toubas P, Duke J, Sekar K, McCaffree M. 1990 Microphonic versus end-tidal carbion dioxide nasal airflow detection in neonates with apnea. Pediatrics 86:950–954
Arnold DH, Spiro DM, Desmond RA, Hagood JS. Estimation of airway obstruction using oximeter plethysmograph waveform data. Respir Res 2005; 6: 65
Percival DB, Walden AT. Spectral analysis for physical applications: multitaper and conventional univariate techniques. Cambridge, New York, NY, USA: Cambridge University Press, 1993; xxvii, 583 pp.
Akay M. Biomedical signal processing. San Diego: Academic Press, 1994; xiv, 377 pp.
Fontenla-Romero O, Guijarro-Berdinas B, Alonso-Betanzos A, Moret-Bonillo V. 2005 A new method for sleep apnea classification using wavelets and feedforward neural networks. Artif Intell Med 34(1):65–76
Hudson D, Cohen M. 1999 Neural networks and artificial intelligence for biomedical engineering. New York: IEEE Press
Khan ZH, Mohapatra SK, Khodiar PK, Ragu Kumar SN. 1998 Artificial neural network and medicine. Indian J Physiol Pharmacol 42:321–342
Hudson DL, Cohen ME, IEEE Engineering in Medicine and Biology Society. Neural networks and artificial intelligence for biomedical engineering. New York: IEEE Press, 2000; xxiii, 306 pp.
Johansson A. 2003 Neural network for photoplethysmographic respiratory rate monitoring. Med Biol Eng Comp 41(3):242–248
Haykin S. 1999 Neural networks: a comprehensive foundation. Upper Saddle River, NJ: Prentice Hall
Lalitha V, Eswaran C. 2007 Automated detection of anesthetic depth levels using chaotic features with artificial neural networks. J Med Syst 31:445–452
Zhang XS, Huang JW, Roy RJ. 2002 Modeling for nuromonitoring depth of anesthesia. Crit Rev Biomed Eng 30:1049–1046
Aarabi A, Grebe R, Wallois F. 2007 A multistage knowledge-based system for EEG seizure detection in newborn infants. Clin Neurophysiol 118:2781–2797
Wexler L, Bergel D, Gabe I, Makin G, Mils C. 1968 Velocity of blood flow in normal human venae cavae. Circ Res 23:349–359
Katz J, Hynson J. 1995 Respiratory monitoring. In: Blitt CD, Hines RL eds. Monitoring in anesthesia and critical care medicine, 3rd Edition. New York: Churchill Livingstone, 315–362
Shelley K, Shelley S. 2001 Pulse oximeter waveform: photoelectric plethysmography. In: Lake C ed. Clinical monitoring: practical applications for anesthesia and critical care. Philadelphia: W.B Saunders, 420–428
Hartert T, Wheeler A, Sheller J. 1999 Use of pulse oximetry to recognize severity of airflow obstruction in obstructive airway disease. Chest 115:475–481
Nakajima K, Tamura T, Miike H. 1996 Monitoring of heart and respiratory rates by photoplethysmography using a digital filtering technique. Med Eng Phys 18:365–372
Nilsson L, Johansson A, Kalman S. 2005 Respiration can be monitored by photoplethysmography with high sensitivity and specificity regardless of anesthesia and ventilatory mode. Acta Anaesthesiol Scand 49:1157–1162
Leonard P, Grubb NR, Addison PS, Clifton D, Watson JN. 2004 An algorithm for the detection of individual breaths from the pulse oximeter waveform. J Clin Monit Comput 18(5–6):309–312
Johansson A, Stromberg T. 2000 Influence of tidal volume and thoraco-abdominal separation on the respiratory induced variation of the photoplethysmogram. J Clin Monit 16:575–581
Nijboer J, Dorlas J, Mahieu H. 1981 Photoelectric plethysmography–some fundamental aspects of the reflection and transmission method. Clin Phys Physiol Meas 2:205–215
Wiklund L, Hok B, Stahl K, Jordeby-Jonsson A. 1994 Post-anesthesia monitoring revisited: frequency of true and false alarms from different monitoring devices. J Clin Anesth 6:182–188
Drummond GB, Nimmo AF, Elton RA. 1996 Thoracic impedance used for measuring chest wall movement in postoperative patients. Br J Anaesth 77(3):327–332
Clarenbach CF, Senn O, Brack T, Kohler M, Bloch KE. 2005 Monitoring of ventilation during exercise by a portable respiratory inductive plethysmograph. Chest 128(3):1282–1290
Author information
Authors and Affiliations
Corresponding author
Additional information
Knorr-Chung BR, McGrath SP, Blike GT. Identifying airway obstructions using Photoplethysmography (PPG).
Rights and permissions
About this article
Cite this article
Knorr-Chung, B.R., McGrath, S.P. & Blike, G.T. Identifying Airway Obstructions Using Photoplethysmography (PPG). J Clin Monit Comput 22, 95–101 (2008). https://doi.org/10.1007/s10877-008-9110-7
Received:
Accepted:
Published:
Issue Date:
DOI: https://doi.org/10.1007/s10877-008-9110-7